Physical-space Multi-body Mesh Detection Achieved by Local Alignment and Global Dense Learning.
IEEE/CVF Winter Conference on Applications of Computer Vision(2024)
摘要
From monocular RGB images captured in the wild, detecting multi-body 3D meshes in physical sizes and locations is notoriously difficult due to the diverse visual ambiguity and lack of explicit depth measurement. Modern DNN approaches made numerous advances based on either two-stage Region-of-Interests(RoI)-Align or single-stage fixed Field-of-View (FoV) detector frameworks for two main subtasks: local pelvis-centered mesh regression and global body-to-camera translation regression. However, sub-meter-level physical-space monocular mesh detection is still out of reach by existing solutions. In this paper, we recognize two common drawbacks: (1) The local meshes are usually estimated without explicitly aligning body features under image-space scaling, occlusion, and truncation; (2) The global translations are estimated based on a weak-perspective assumption, which tricks the network into prioritizing image-space (front-view) mesh alignment and leads to inaccurate mesh depth. We introduce Physical-space Multi-body Mesh Detection (PMMD), in which (1) Locally, we preserve the body aspect ratio, align the body-to-RoI layout, and densely refine the person-wise RoI features for robustness; (2) Globally, we learn dense-depth-guided features to amend the body-wise local feature for physical depth estimation. With the cleaned local features and explicit local-global associations, PMMD achieves the best centimeter-level local mesh metrics and the first sub-meter-level global mesh metrics from monocular images in 3DPW and AGORA datasets.
更多查看译文
关键词
Algorithms,Image recognition and understanding,Algorithms,Biometrics,face,gesture,body pose
AI 理解论文
溯源树
样例
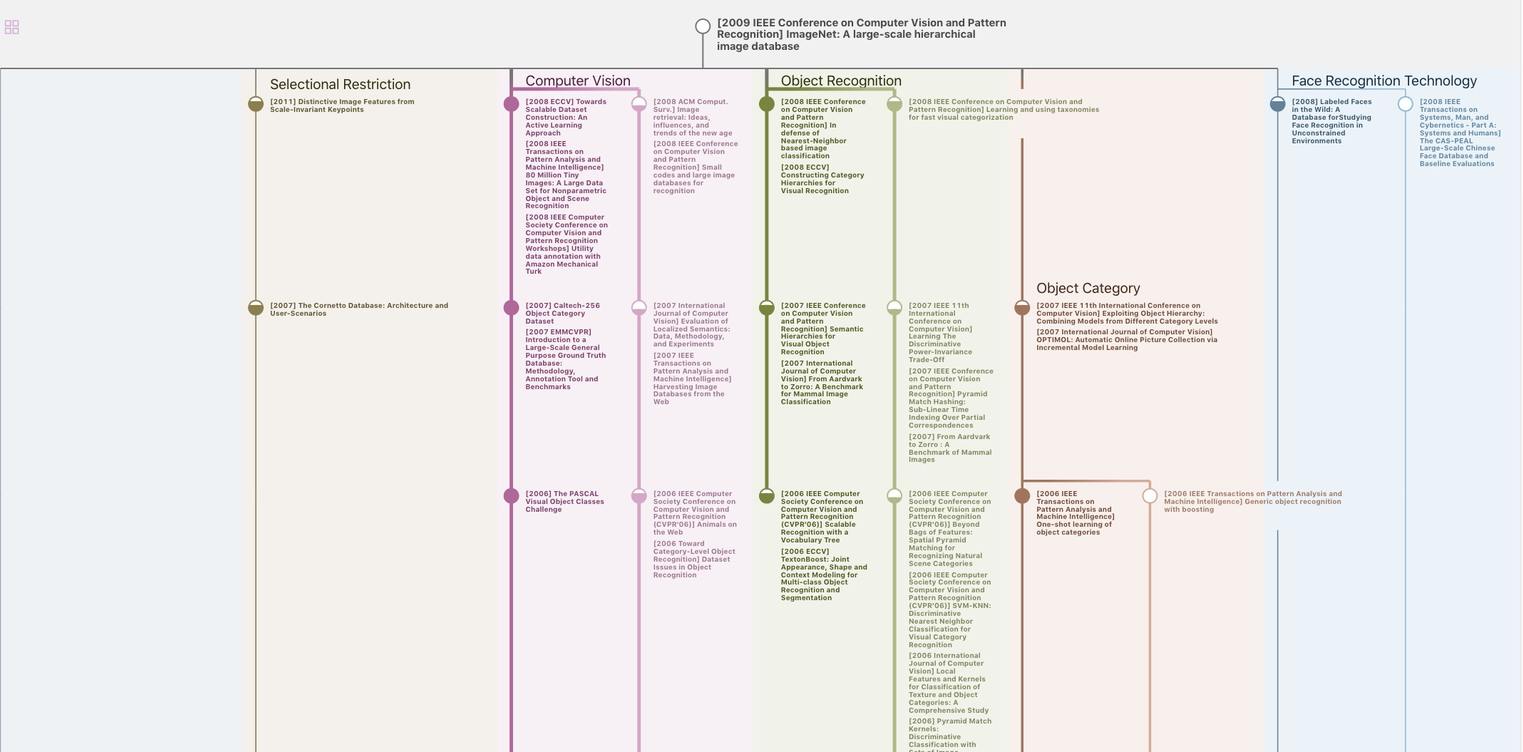
生成溯源树,研究论文发展脉络
Chat Paper
正在生成论文摘要