Electrode Placement Optimization for Electrical Impedance Tomography Using Active Learning
ADVANCED ENGINEERING MATERIALS(2024)
摘要
Electrical impedance tomography (EIT) offers a versatile imaging modality with a multitude of applications, although it encounters accuracy limitations. Herein, a novel systematic framework is presented that integrates a neural network (NN), active learning, and transfer learning to optimize electrode placement, improving image reconstruction performance based on user-defined metrics. Given the many-to-one mapping between electrode configuration and the performance metric, the approach utilizes a NN that predicts performance metrics from electrode placement input. To maintain NN's prediction accuracy for unseen electrode configurations, performance metrics are maximized while iteratively updating the NN via active learning during the optimization process. Transfer learning is employed to expedite optimization of electrode placements for time-consuming iterative reconstruction techniques by fine-tuning a NN initially trained on one-step reconstruction data. The method is validated using two representative reconstruction methods: one-step reconstruction with Newton's one-step error reconstructor prior and the iterative total variation method. This research underscores the potential of the proposed framework in addressing EIT's inherent limitations and augmenting its performance across diverse applications and reconstruction methods. The framework could potentially contribute to the advancement of noninvasive medical imaging, structural health monitoring, strain sensing, robotics, and other fields that depend on EIT. This study presents an innovative framework for improving electrical impedance tomography image quality through electrode placement optimization. Utilizing a genetic algorithm guided by neural network predictions and enhanced with transfer learning for data efficiency, the approach iteratively refines image accuracy metrics. Validated across both one-step and iterative reconstruction methods, it shows promise for advancing nondestructive imaging in engineering applications.image (c) 2024 WILEY-VCH GmbH
更多查看译文
关键词
data-driven optimization,electrical impedance tomography,machine learning
AI 理解论文
溯源树
样例
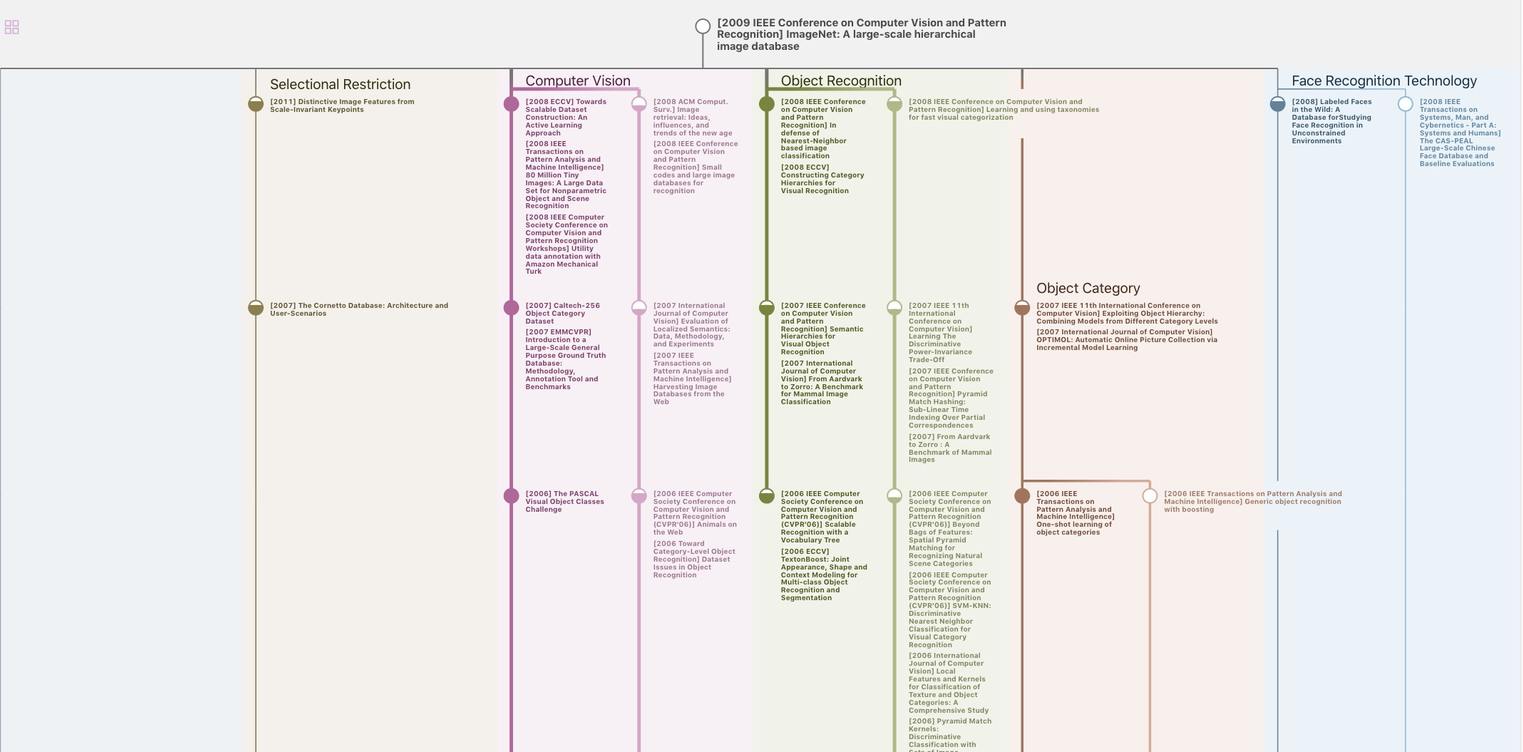
生成溯源树,研究论文发展脉络
Chat Paper
正在生成论文摘要