Resource Allocation in Multi-User Cellular Networks: A Transformer-Based Deep Reinforcement Learning Approach
CHINA COMMUNICATIONS(2024)
摘要
To meet the communication services with diverse requirements, dynamic resource allocation has shown increasing importance. In this paper, we consider the multi -slot and multi-user resource allocation (MSMU-RA) in a downlink cellular scenario with the aim of maximizing system spectral efficiency while guaranteeing user fairness. We first model the MSMURA problem as a dual -sequence decision -making process, and then solve it by a novel Transformerbased deep reinforcement learning (TDRL) approach. Specifically, the proposed TDRL approach can be achieved based on two aspects: 1) To adapt to the dynamic wireless environment, the proximal policy optimization (PPO) algorithm is used to optimize the multi -slot RA strategy. 2) To avoid co -channel interference, the Transformer -based PPO algorithm is presented to obtain the optimal multi-user RA scheme by exploring the mapping between user sequence and resource sequence. Experimental results show that: i) the proposed approach outperforms both the traditional and DRL methods in spectral efficiency and user fairness, ii) the proposed algorithm is superior to DRL approaches in terms of convergence speed and generalization performance.
更多查看译文
关键词
dynamic resource allocation,multi-user cellular network,spectrum efficiency,user fairness
AI 理解论文
溯源树
样例
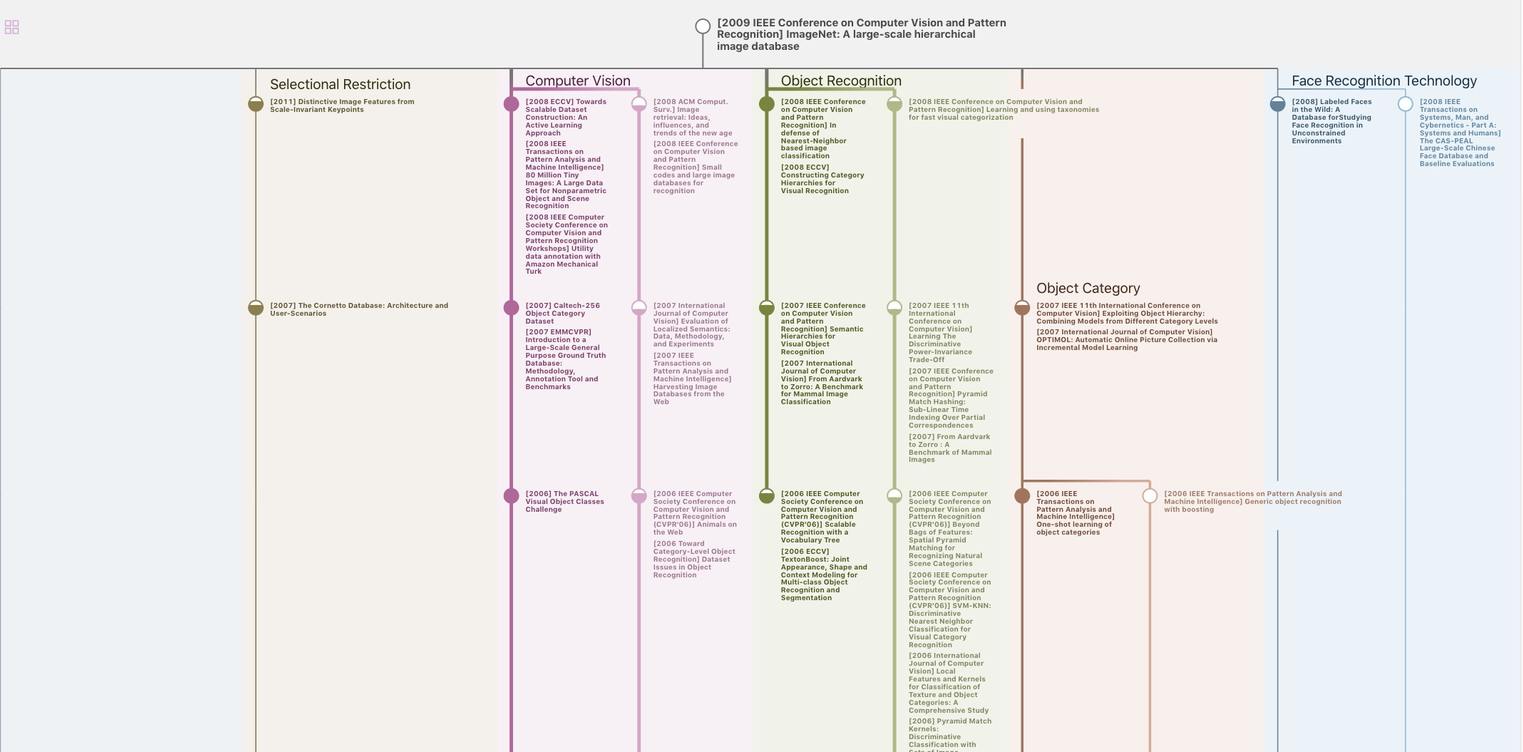
生成溯源树,研究论文发展脉络
Chat Paper
正在生成论文摘要