LipAT: Beyond Style Transfer for Controllable Neural Simulation of Lipstick using Cosmetic Attributes.
IEEE/CVF Winter Conference on Applications of Computer Vision(2024)
摘要
Lipstick virtual try-on (VTO) experiences have become widespread across the e-commerce sector and assist users in eliminating the guesswork of shopping online. However, such experiences still lack in both realism and accuracy. In this work, we propose LipAT, a neural framework that blends the strengths of Physics-Based Rendering (PBR) and Neural Style Transfer (NST) approaches to directly apply lipstick onto face images given lipstick attributes (e.g., colour, finish type). LipAT consists of a physics aware neural lipstick application module (LAM) to apply lipstick on face images given its attributes and Lipstick Refiner Module (LRM) to improve the realism by refining the imperfections. Unlike the NST approaches, LipAT allows precise and controllable lipstick attribute preservation, without requiring crude approximations and inference of various intertwined environment factors (e.g., scene lighting, face structure etc) involved in image generation that is required for accurate PBR. We propose an experimental framework with quantitative metrics to evaluate different desirable aspects of the lipstick attribute driven try-on alongside user studies to further validate our findings. Our results show that LipAT considerably outperforms fully-automated PBR approaches in preserving realism and the NST approaches in preserving various lipstick attributes such as finish types.
更多查看译文
关键词
Applications,Commercial / retail,Algorithms,Computational photography,image and video synthesis
AI 理解论文
溯源树
样例
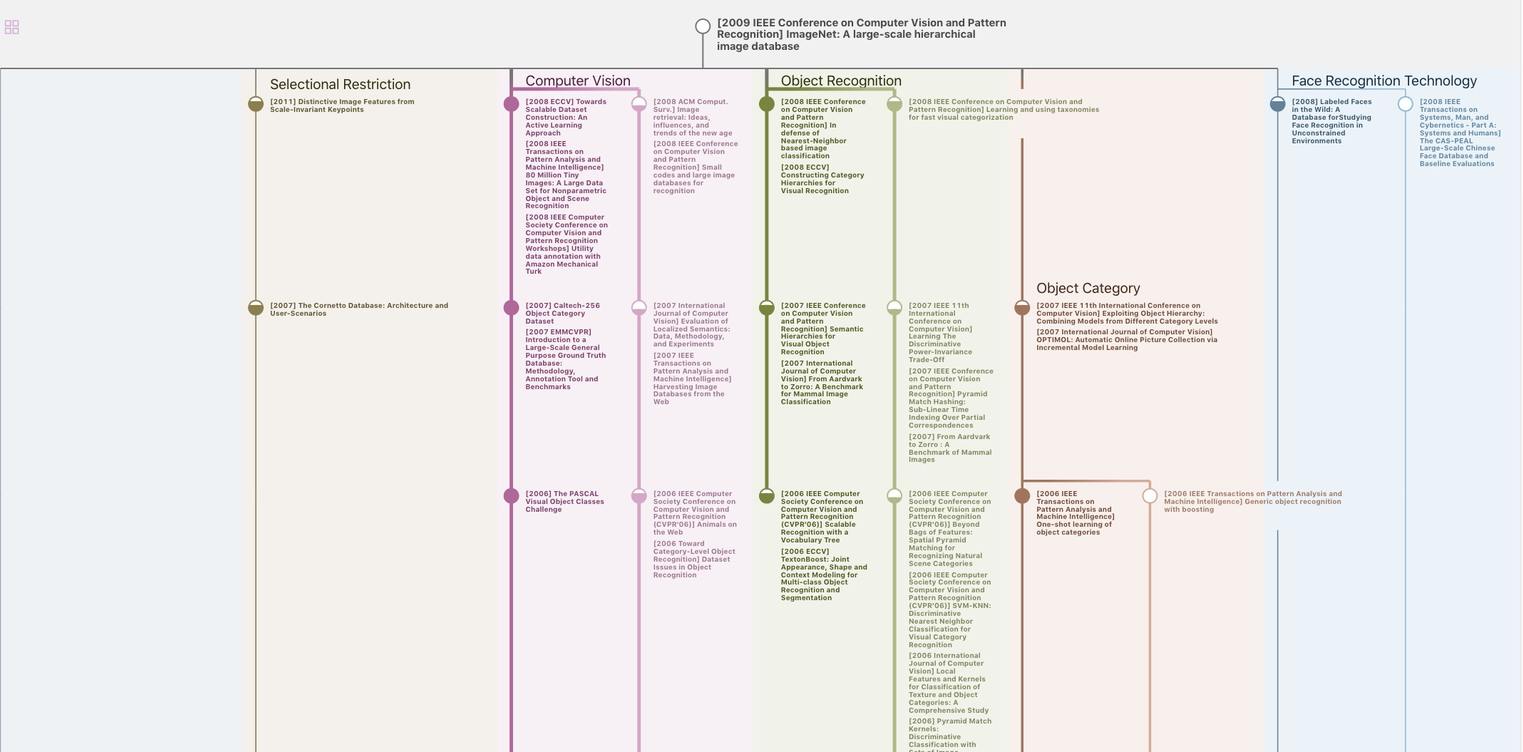
生成溯源树,研究论文发展脉络
Chat Paper
正在生成论文摘要