Transformer-based Compound Correlation Miner for Smart Home Anomaly Detection.
IEEE International Conference on Cloud Networking(2023)
Abstract
IoT-enabled smart homes can be double-edged swords. On one side, the convenience and efficiency brought about by smart device integrations can improve the standard of living dramatically. However, on the other side, the prevalent interconnectivity among home devices can drastically increase the potential risk of attack. Therefore, in order to reduce the possibility of a successful attack we propose a complex correlation-based anomaly detection system powered by intricate two-to-one correlations. Through the use of a state-of-the-art transformer model, we present a novel correlation mining mechanism that leverages the power of attention weights to develop an understanding of the underlying correlations that exist between IoT events in a smart home environment. Using this knowledge, we use a special validation algorithm to verify 52 two-to-one correlations in our system. Furthermore, we simulate four distinct attack scenarios and attain an average detection accuracy and recall of 96.59% and 97.38% respectively. Our results indicate that our method is effective at identifying a range of IoT attacks and successfully demonstrates the capabilities of IoT correlations.
MoreTranslated text
Key words
Smart home,Security,Anomaly Detection
AI Read Science
Must-Reading Tree
Example
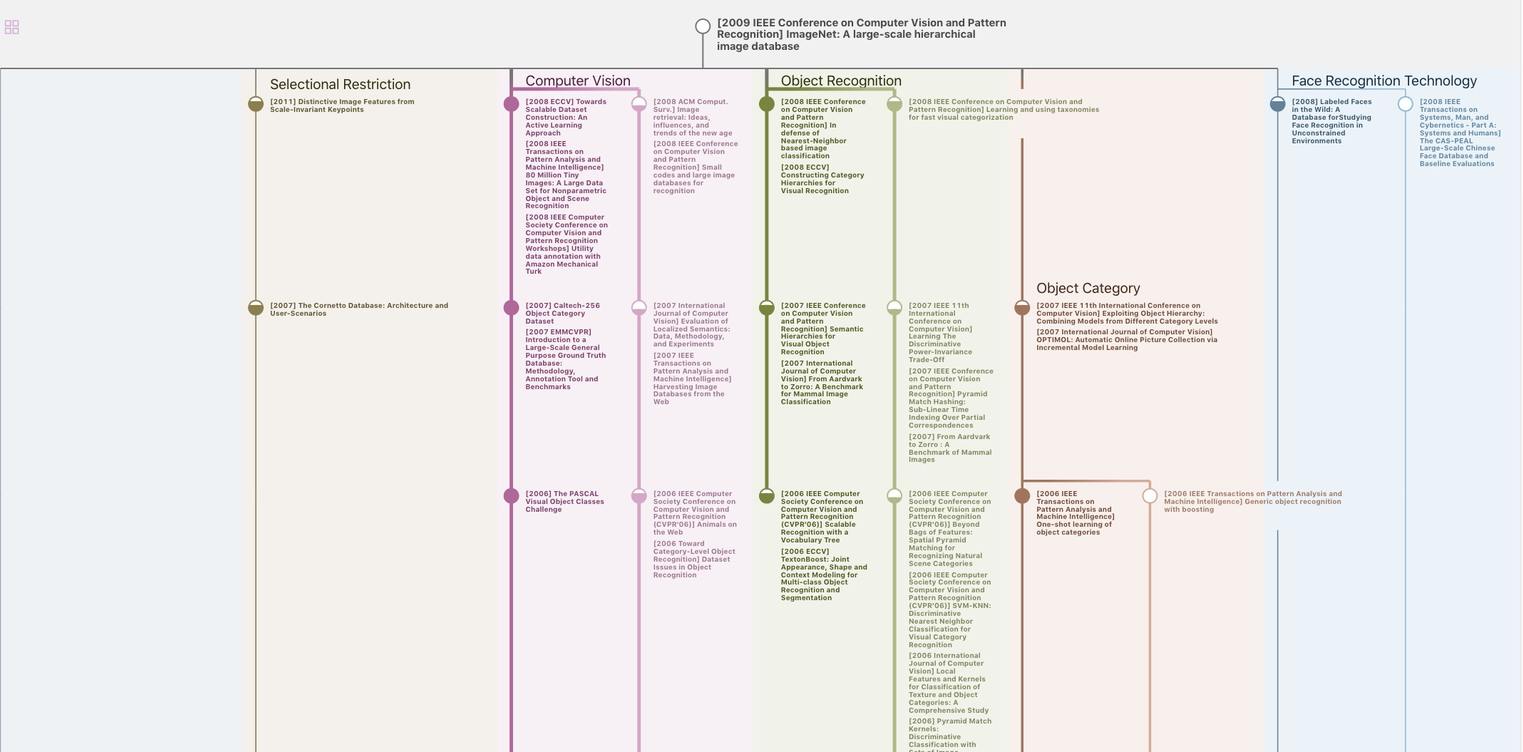
Generate MRT to find the research sequence of this paper
Chat Paper
Summary is being generated by the instructions you defined