MagneticPillars: Efficient Point Cloud Registration through Hierarchized Birds-Eye-View Cell Correspondence Refinement.
IEEE/CVF Winter Conference on Applications of Computer Vision(2024)
摘要
Recent point cloud registration approaches often deal with a consecutive determination of coarse and fine feature correspondences for hierarchical pose refinement. Due to the unordered nature of point clouds, a common way to generate a subsampled representation for the coarse matching step is by applying 3D-sensitive convolution approaches. However, expensive grouping mechanisms such as nearest neighbour search have to be used to determine the associated fine features, generating individual associations for each point cloud and leading to an increased overall run-time. Furthermore current methods often tend to predict deficient point correspondences and rely on additional filtering by expensive registration backends like RANSAC impeding their application in time critical systems.To overcome these challenges, we present MagneticPillars utilizing a Birds-Eye-View (BEV) grid representation, entailing fixed affiliations between coarse and fine feature cells. We show that by extracting correspondences in this manner, a small amount of key points is already sufficient to achieve an accurate pose estimation without external optimization methods like RANSAC. We evaluate our approach on two autonomous driving datasets for the task of point cloud registration by applying SVD as the backend, where we outperform recent state-of-the-art methods, reducing the rotation and translation error by 12% and 40%, respectively, and to top it all off, cutting runtime in half.
更多查看译文
关键词
Applications,Autonomous Driving,Algorithms,Machine learning architectures,formulations,and algorithms,Applications,Robotics
AI 理解论文
溯源树
样例
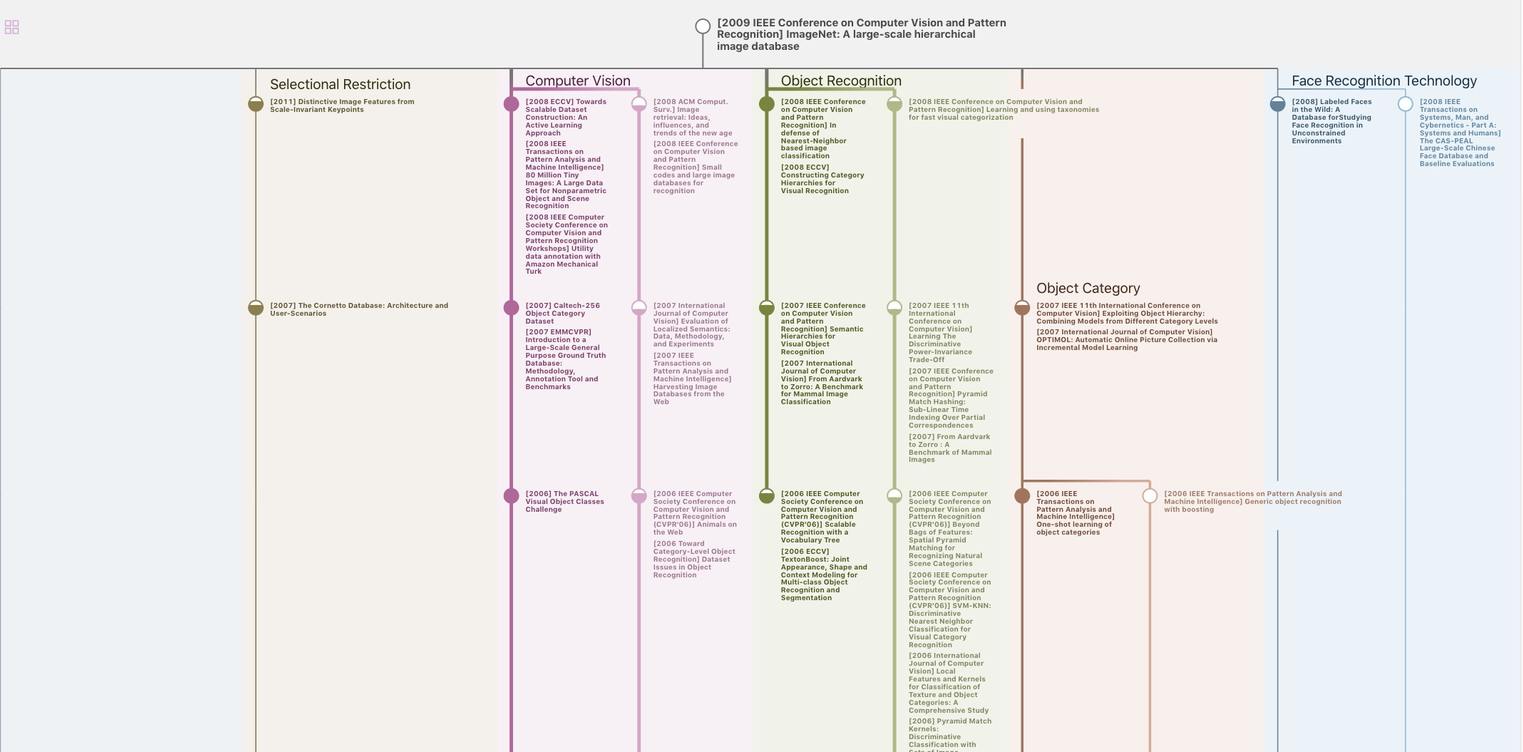
生成溯源树,研究论文发展脉络
Chat Paper
正在生成论文摘要