SOC Estimation for Lithium-Ion Battery Charging and Discharging Based on AMLRLS and MIAEKF Algorithm
2023 2nd Asia Power and Electrical Technology Conference (APET)(2023)
摘要
In order to solve the problems of time-consuming testing of lithium-ion battery SOC and low accuracy of SOC estimation method in lithium-ion battery power metering, this paper proposes a lithium-ion battery charging and discharging SOC estimation method based on AMLRLS (Adaptive Forgetting Factor Recursive Least Squares) and MIAEKF (Multi-Innovation Adaptive Extended Kalman Filtering) algorithms. Since the accuracy of model parameters is the basis for ensuring the accuracy of the battery charging and discharging SOC estimation, this paper adopts the AMLRLS algorithm to identify the model parameters online, and the experimental results show that: AMLRLS algorithm reduces the root-mean-square error between the model output voltage and the test voltage by about 11.38%, and the maximum error reduces by about 5.3691% compared to the FFRLS algorithm; In order to ensure the accuracy of SOC estimation method, this paper adopts MAIEKF algorithm to achieve the online estimation of SOC of lithium-ion battery, and the experimental results show that the error of SOC estimation under the battery charging and discharging conditions is within ±2%. Under the charging condition, the MIAEKF algorithm reduces the root-mean-square error by about 56% compared with the EKF algorithm, and the maximum error is reduced by about 55.96%. Under the discharge condition, the MIAEKF algorithm reduces the root-mean-square error by about 79.73% and the maximum error by about 94.57% compared with the EKF algorithm.
更多查看译文
关键词
lithiumion battery,SOC online estimation,AMLRLS algorithm,model parameter identification,MAIEKF algorithm
AI 理解论文
溯源树
样例
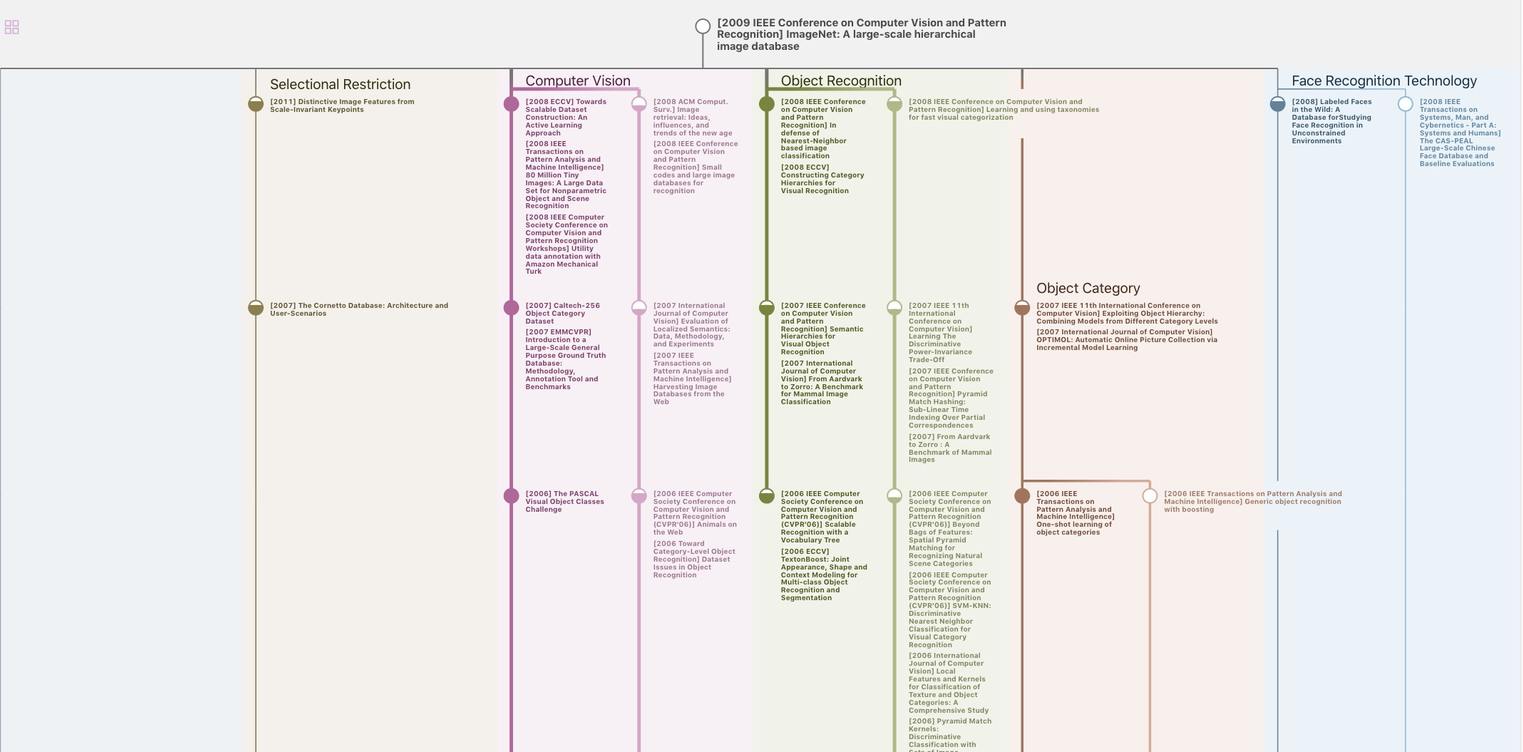
生成溯源树,研究论文发展脉络
Chat Paper
正在生成论文摘要