Guided Cluster Aggregation: A Hierarchical Approach to Generalized Category Discovery.
IEEE/CVF Winter Conference on Applications of Computer Vision(2024)
摘要
Despite advances in image recognition, recognizing novel categories in unlabeled data remains challenging for machine learning methods, even though humans can perform this task with ease. A recently developed setting to tackle this problem is Generalized Category Discovery (GCD), in which the task is to, given a labeled dataset, classify an unlabeled dataset, where the unlabeled dataset contains both known classes and novel classes that do not appear in the labeled data. Existing GCD methods mostly focus on learning strong image representations, on which they then apply a clustering algorithm such as k-means. Despite obtaining good performance, they do not fully exploit the potential of the learned features due to the simple nature of the clustering mechanism. To address this issue, we make use of the fact that local neighborhoods in self-supervised feature spaces are highly homogeneous. We leverage this observation to develop Guided Cluster Aggregation (GCA), a hierarchical approach that first groups the data into small clusters of high purity, then aggregates them into larger clusters. Experiments show that GCA outperforms semi-supervised k-means in most cases, especially in fine-grained classification tasks. Code available at https://github.com/J-L-O/guidedcluster-aggregation.
更多查看译文
关键词
Algorithms,Machine learning architectures,formulations,and algorithms,Algorithms,Image recognition and understanding
AI 理解论文
溯源树
样例
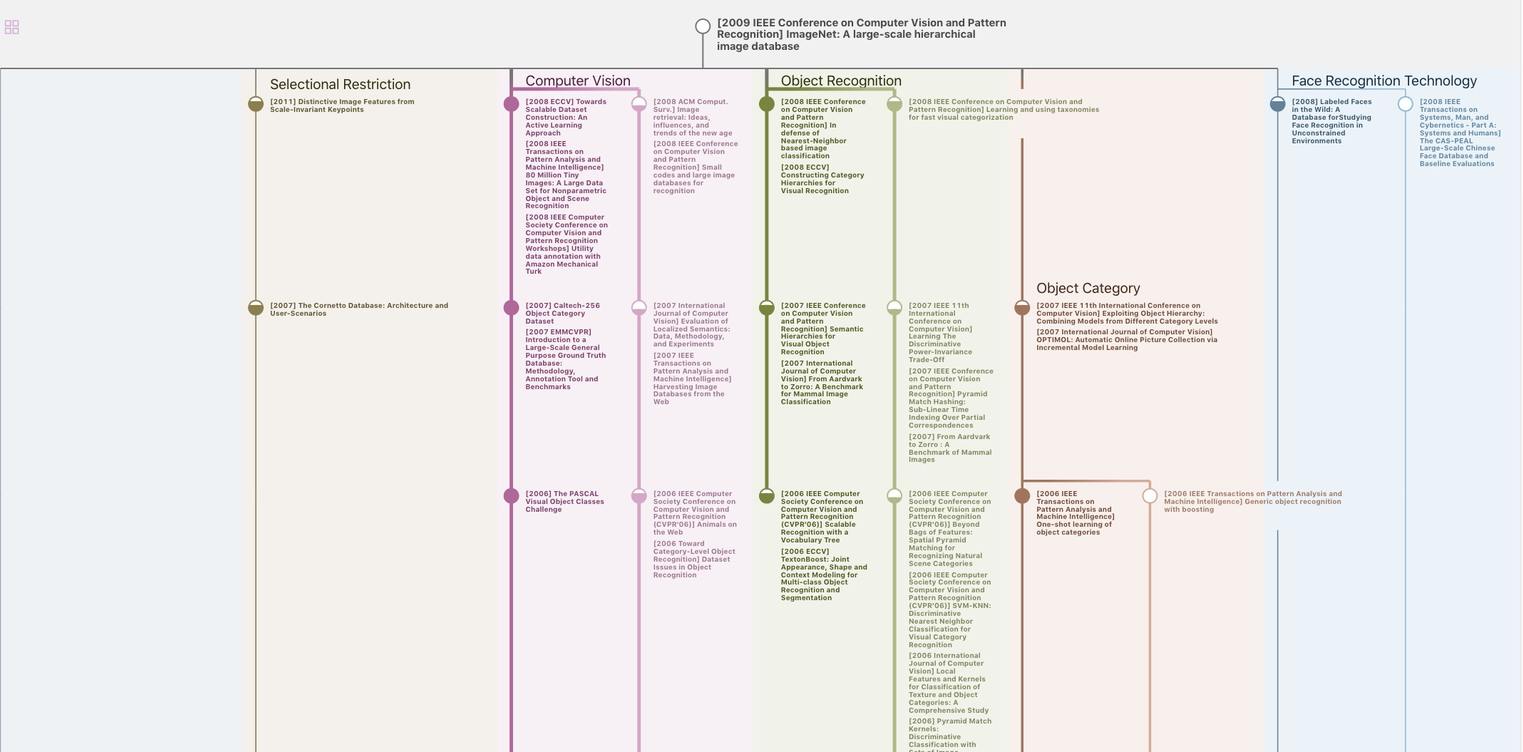
生成溯源树,研究论文发展脉络
Chat Paper
正在生成论文摘要