SSDT: Scale-Separation Semantic Decoupled Transformer for Semantic Segmentation of Remote Sensing Images
IEEE Journal of Selected Topics in Applied Earth Observations and Remote Sensing(2024)
摘要
As we all know, semantic segmentation of remote sensing (RS) images is to classify the images pixel by pixel to realize the semantic decoupling of the images. Most traditional semantic decoupling methods only decouple and do not perform scale-separation operations, which leads to serious problems. In the semantic decoupling process, if the feature extractor is too large, it will ignore the small-scale targets; if the feature extractor is too small, it will lead to the separation of large-scale target objects and reduce the segmentation accuracy. To address this concern, we propose a Scale-separated Semantic Decoupled Transformer(SSDT), which first performs scale-separation in the semantic decoupling process and uses the obtained scale information-rich semantic features to guide the Transformer to extract features. The network consists of five modules, Scale-separated Patch Extraction (SPE), Semantic Decoupled Transformer (SDT), Scale-separated Feature Extraction (SFE), Semantic Decoupling(SD), and Multi-view Feature Fusion Decoder (MFFD). In particular, SPE turns the original image into a linear embedding sequence of three scales; SD divides pixels into different semantic clusters by K-means, and further obtains scale information-rich semantic features; SDT improves the intra-class compactness and inter-class looseness by calculating the similarity between semantic features and image features, the core of which is Decouped Attention. Finally, MFFD is proposed to fuse salient features from different perspectives to further enhance the feature representation. Our experiments on two large-scale fine-resolution RS image datasets (Vaihingen and Potsdam) demonstrate the effectiveness of the proposed SSDT strategy in RS image semantic segmentation tasks.
更多查看译文
关键词
Article submission,IEEE,IEEEtran,journal,paper,template,typesetting,LaTeX
AI 理解论文
溯源树
样例
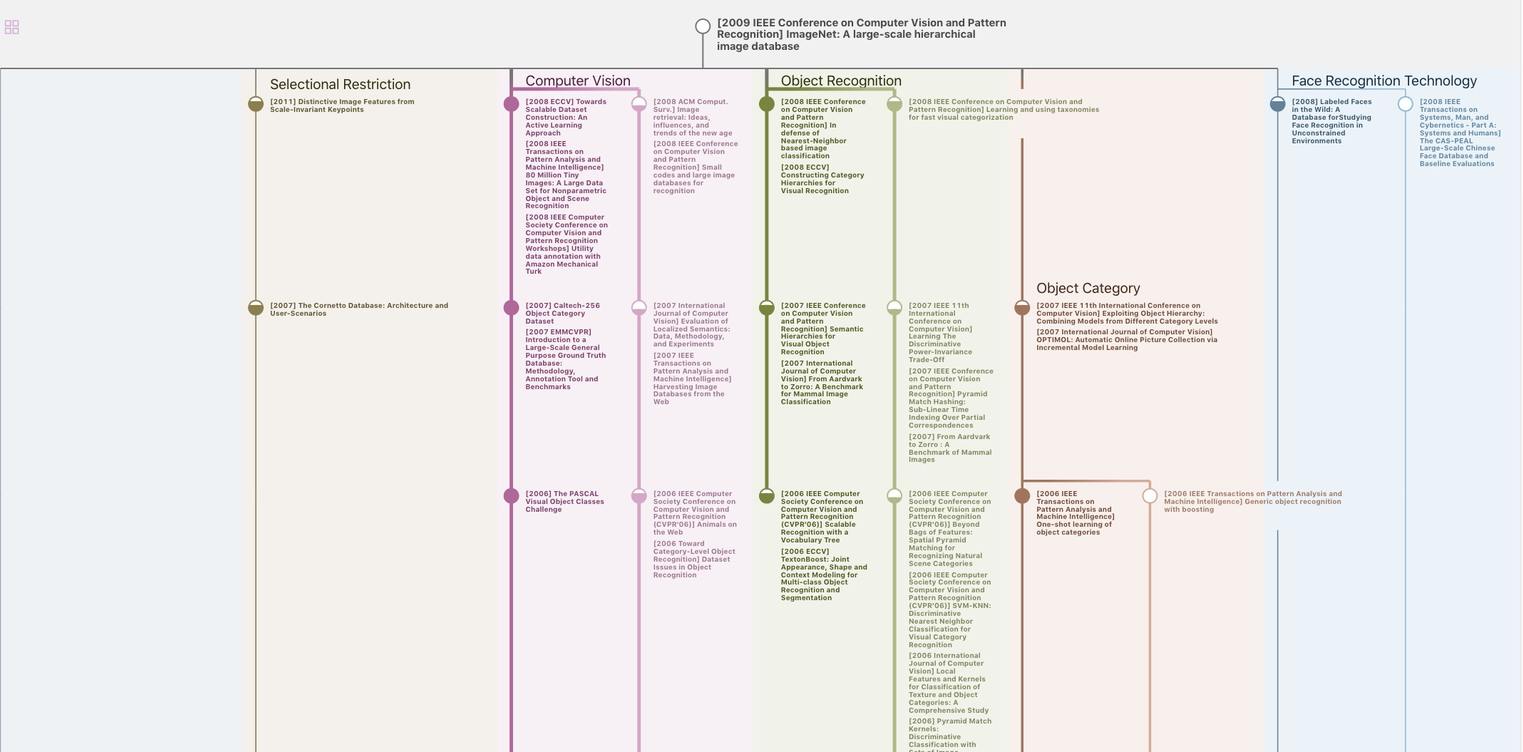
生成溯源树,研究论文发展脉络
Chat Paper
正在生成论文摘要