Training-free Content Injection using h-space in Diffusion Models.
IEEE/CVF Winter Conference on Applications of Computer Vision(2024)
Abstract
Diffusion models (DMs) synthesize high-quality images in various domains. However, controlling their generative process is still hazy because the intermediate variables in the process are not rigorously studied. Recently, the bottleneck feature of the U-Net, namely h-space, is found to convey the semantics of the resulting image. It enables StyleCLIP-like latent editing within DMs. In this paper, we explore further usage of h-space beyond attribute editing, and introduce a method to inject the content of one image into another image by combining their features in the generative processes. Briefly, given the original generative process of the other image, 1) we gradually blend the bottleneck feature of the content with proper normalization, and 2) we calibrate the skip connections to match the injected content. Unlike custom-diffusion approaches, our method does not require time-consuming optimization or fine-tuning. Instead, our method manipulates intermediate features within a feed-forward generative process. Furthermore, our method does not require supervision from external networks. Project page: https://curryjung.github.io/DiffStyle/
MoreTranslated text
Key words
Algorithms,Generative models for image,video,3D,etc.,Algorithms,Computational photography,image and video synthesis
AI Read Science
Must-Reading Tree
Example
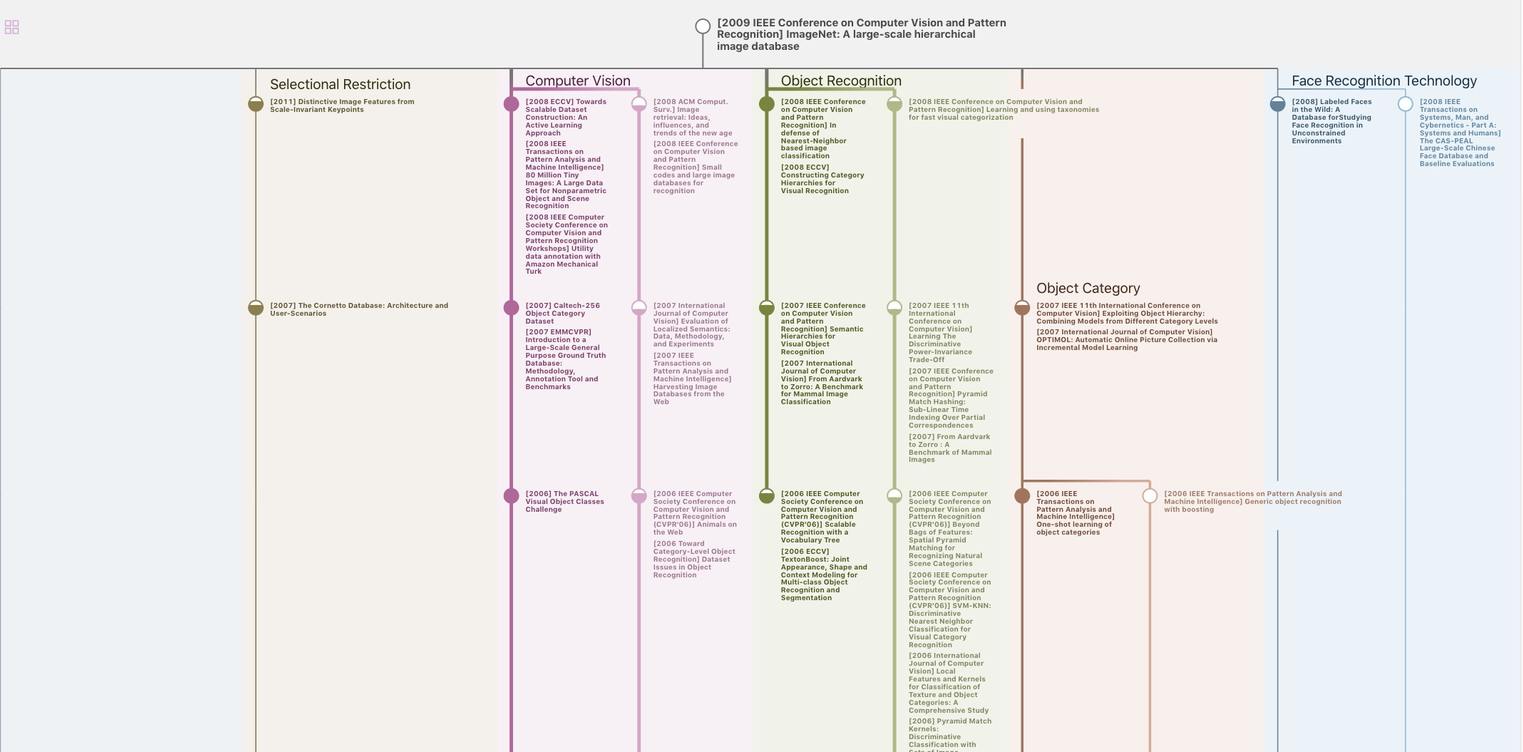
Generate MRT to find the research sequence of this paper
Chat Paper
Summary is being generated by the instructions you defined