UNSPAT: Uncertainty-Guided SpatioTemporal Transformer for 3D Human Pose and Shape Estimation on Videos.
IEEE/CVF Winter Conference on Applications of Computer Vision(2024)
摘要
We propose an efficient framework for 3D human pose and shape estimation from a video, named Uncertainty-Guided SpatioTemporal Transformer (UNSPAT). Unlike previous video-based methods that consider temporal relationships with global average pooled features, our approach incorporates both spatial and temporal dimensions without compromising spatial information. We address the excessive complexity of spatiotemporal attention through two modules: Spatial Alignment Module (SAM) and Space2Batch. The modules align input features and compute temporal attention at every spatial position in a batch-wise manner. Furthermore, our uncertainty-guided attention re-weighting module improves performance by diminishing the impact of artifacts. We demonstrate the effectiveness of the UNSPAT on widely used benchmark datasets and achieve state-of-the-art performance. Our method is robust to challenging scenes, such as occlusion, and cluttered backgrounds, showing its potential for real-world applications.
更多查看译文
关键词
Algorithms,3D computer vision,Algorithms,Biometrics,face,gesture,body pose
AI 理解论文
溯源树
样例
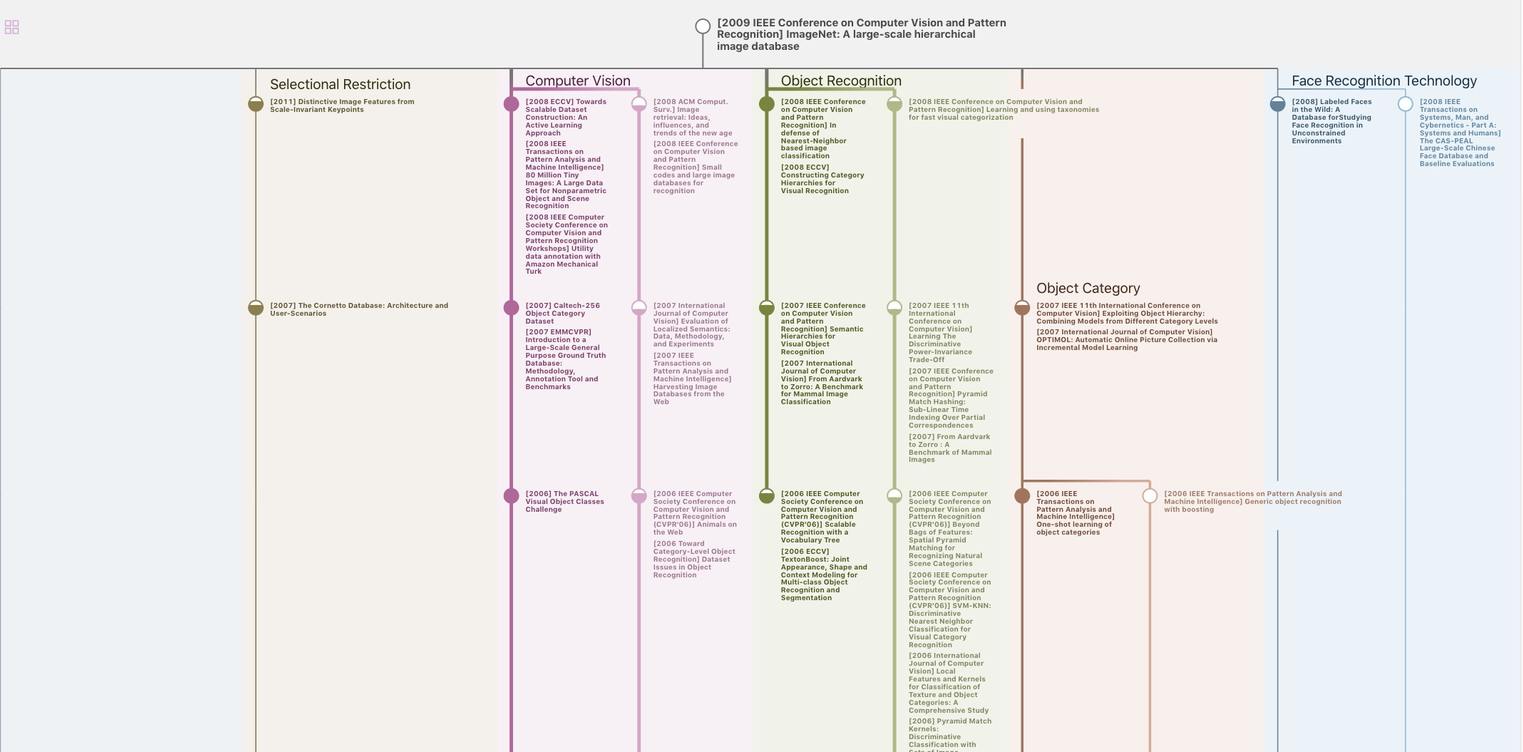
生成溯源树,研究论文发展脉络
Chat Paper
正在生成论文摘要