SSP: Semi-signed prioritized neural fitting for surface reconstruction from unoriented point clouds.
IEEE/CVF Winter Conference on Applications of Computer Vision(2024)
摘要
Reconstructing 3D geometry from unoriented point clouds can benefit many downstream tasks. Recent shape modeling methods mostly adopt implicit neural representation to fit a signed distance field (SDF) and optimize the network by unsigned supervision. However, these methods occasionally have difficulty in finding the coarse shape for complicated objects, especially suffering from the "ghost" surfaces (i.e., fake surfaces that should not exist). To guide the network quickly fit the coarse shape, we propose to utilize the signed supervision in regions that are obviously outside the object and can be easily determined, resulting in our semi-signed supervision. To better recover high-fidelity details, a novel loss-based region sampling strategy and a progressive positional encoding (PE) method are applied to prioritize the optimization towards underfitting and complicated regions. Specifically, we voxelize and partition the object space into sign-known and sign-uncertain regions, in which different supervisions are applied. Besides, we adaptively adjust the sampling rate of each voxel according to the tracked reconstruction loss, so that the network can focus more on the complicated under-fitting regions. We conduct extensive experiments to demonstrate that our method achieves state-of-the-art performance compared to the existing fitting-based methods and comparable performance to learning-based methods on multiple datasets. The code is publicly available at https://github.com/Runsong123/SSP.
更多查看译文
关键词
Algorithms,3D computer vision,Applications,Visualization
AI 理解论文
溯源树
样例
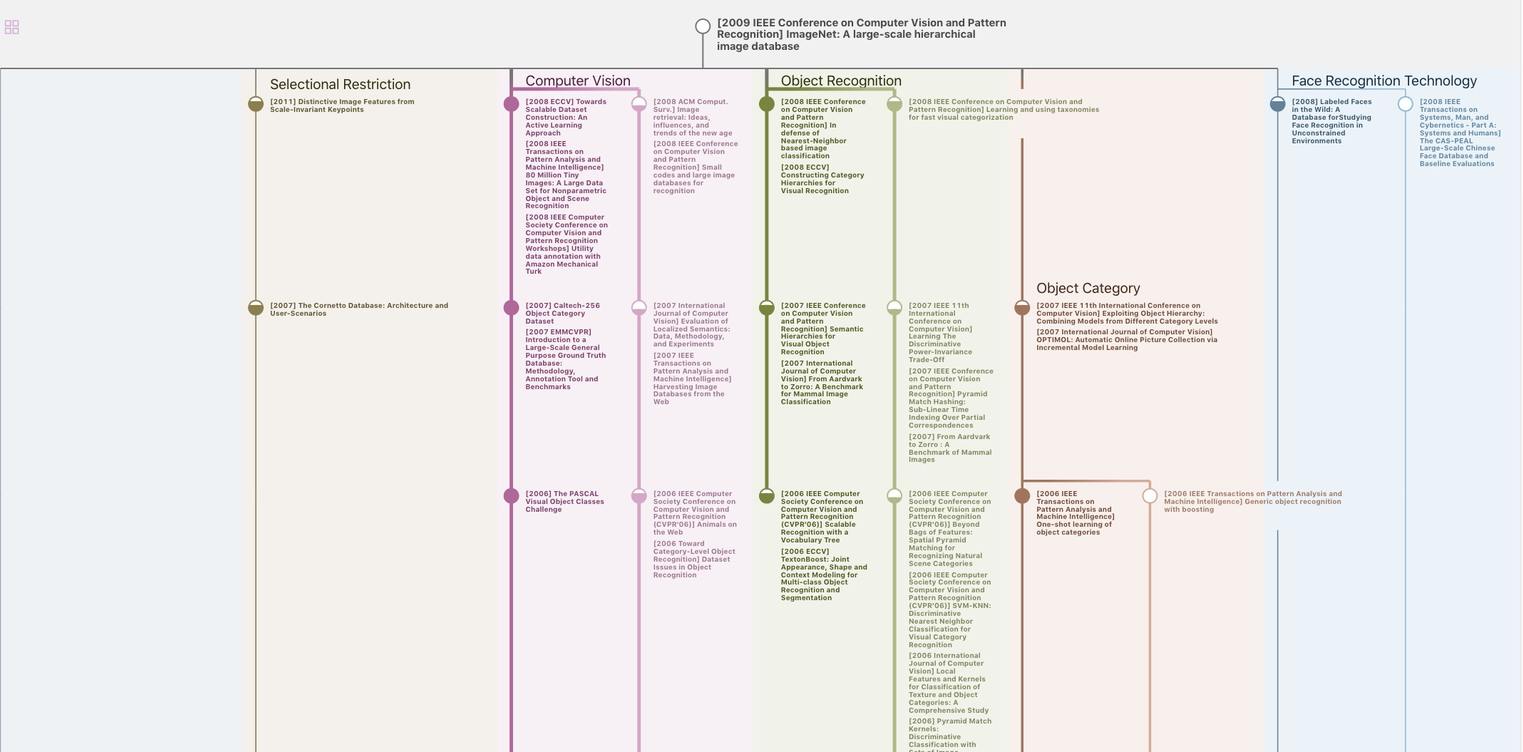
生成溯源树,研究论文发展脉络
Chat Paper
正在生成论文摘要