Dynamic Deep Learning Based Super-Resolution For The Shallow Water Equations
arxiv(2024)
摘要
Using the nonlinear shallow water equations as benchmark, we demonstrate that
a simulation with the ICON-O ocean model with a 20km resolution that is
frequently corrected by a U-net-type neural network can achieve discretization
errors of a simulation with 10km resolution. The network, originally developed
for image-based super-resolution in post-processing, is trained to compute the
difference between solutions on both meshes and is used to correct the coarse
mesh every 12h. Our setup is the Galewsky test case, modeling transition of a
barotropic instability into turbulent flow. We show that the ML-corrected
coarse resolution run correctly maintains a balance flow and captures the
transition to turbulence in line with the higher resolution simulation. After 8
day of simulation, the L_2-error of the corrected run is similar to a
simulation run on the finer mesh. While mass is conserved in the corrected
runs, we observe some spurious generation of kinetic energy.
更多查看译文
AI 理解论文
溯源树
样例
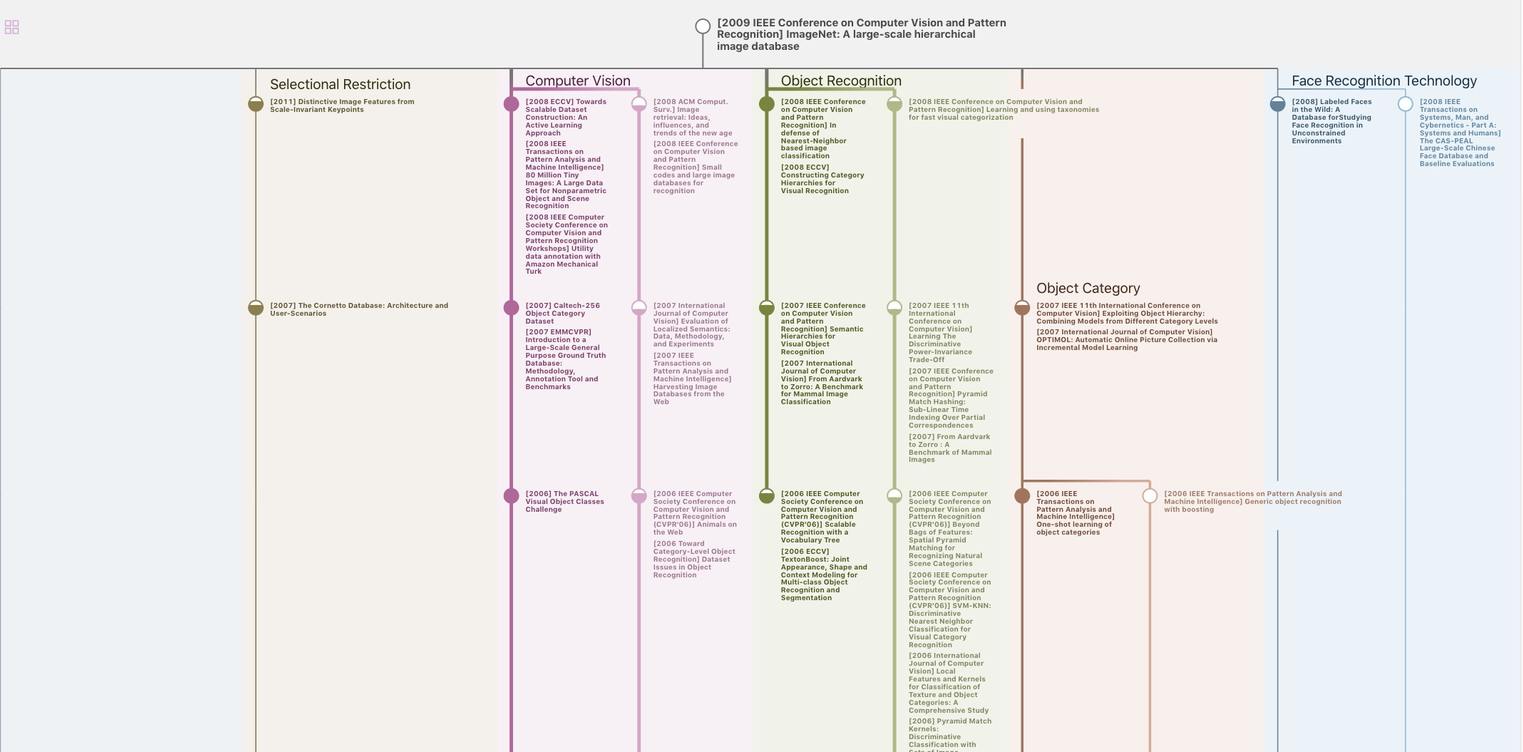
生成溯源树,研究论文发展脉络
Chat Paper
正在生成论文摘要