Revolutionize the Oceanic Drone RGB Imagery with Pioneering Sun Glint Detection and Removal Techniques
IEEE/CVF Winter Conference on Applications of Computer Vision(2024)
Abstract
The issue of sun glint poses a significant challenge for ocean remote sensing with high-resolution ocean drone imagery, as it contaminates images and obstructs crucial features in shallow-waters, leading to inaccurate benthic substrates identification. While various physics-based statistical solutions have been proposed to address this optical issue in remote sensing, there is a lack of sun glint detection and removal methods specifically designed for high-resolution consumer-grade drone RGB imagery. In this paper, we present a pioneering pipeline for sun glint detection and removal in high-resolution drone RGB images, aiming to restore the real features that are hindered by sun glint. Our approach involves the development of a Foreground Attention-based Semantic Segmentation Network (FANet) for accurate and precise sun glint detection, while effective sun glint removal is achieved through pixel propagation using an optical flow field. Experimental results demonstrate the effectiveness of our FANet in identifying sun glint, achieving IoU accuracy of 81.34% for sun glint pixels and 99.52% for non-sun glint background pixels. Furthermore, the quantitative evaluation of sun glint removal using two well-known metrics show that our method outperforms the GAN-based image restoration method (DeepFillv2) and the conventional image interpolation method (Fast Marching Method, hereafter referred to as FMM). Thus, our pipeline lays the foundation for accurate and precise marine coastal ecological monitoring and seafloor topographic mapping using consumer-grade drone at a low cost.
MoreTranslated text
Key words
Applications,Remote Sensing,Algorithms,Image recognition and understanding,Applications,Environmental monitoring / climate change / ecology
AI Read Science
Must-Reading Tree
Example
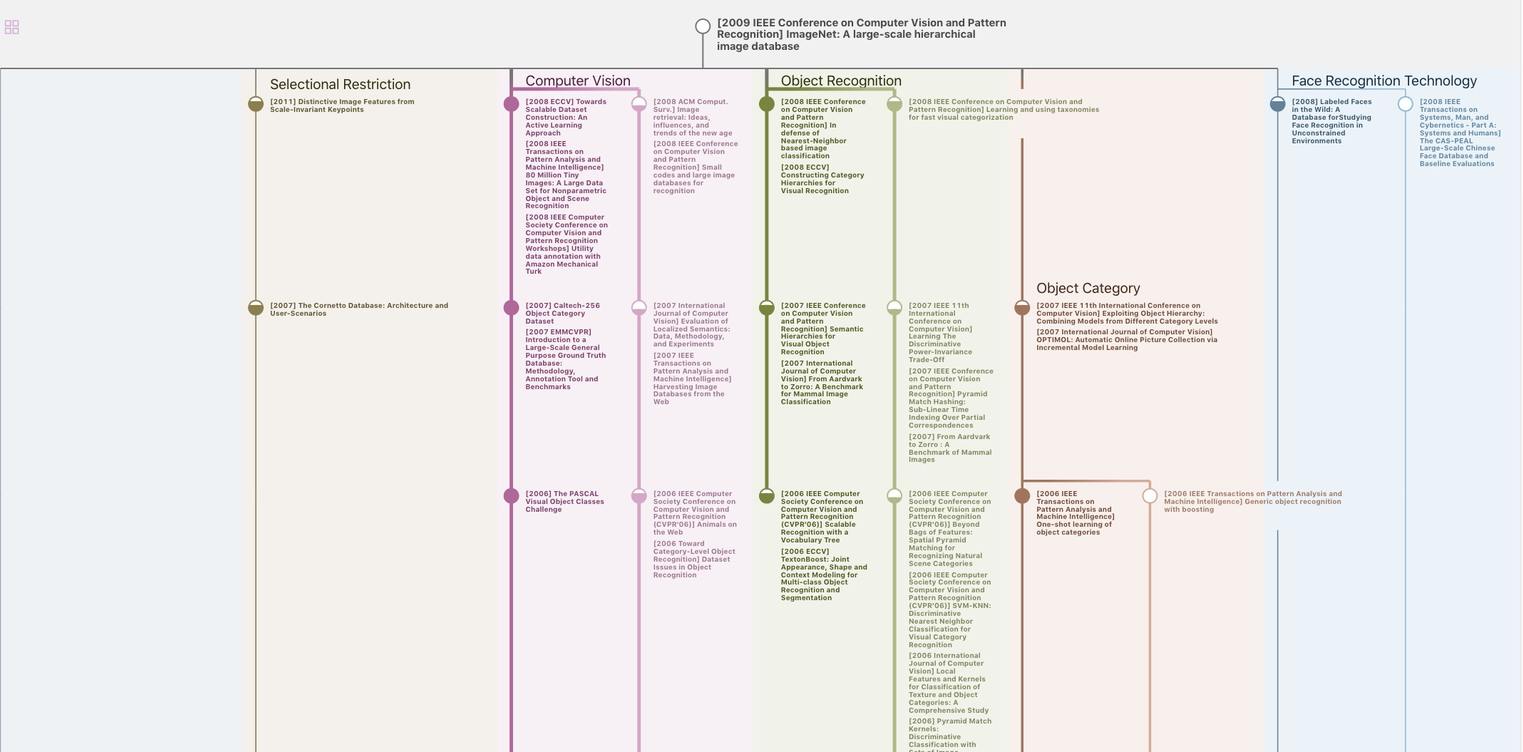
Generate MRT to find the research sequence of this paper
Chat Paper
Summary is being generated by the instructions you defined