Performance Evaluation of Federated Learning for Anomaly Network Detection
2023 Congress in Computer Science, Computer Engineering, & Applied Computing (CSCE)(2023)
摘要
Network anomaly detection is crucial for ensuring the security and dependability of modern networked systems. Traditional machine learning methods face scalability, data security, and adaptability challenges. This paper explores federated learning, a collaborative learning technique, for network anomaly detection. The federated learning approach allows multiple computing agents to train a model on their local data without sharing sensitive information. Using a real-world dataset of network traffic, the effectiveness of federated learning is evaluated and compared with rule-based and machine-learning-based methods. The results show that the federated learning-based approach outperforms traditional methods in terms of accuracy, precision, and recall. Achieving an accuracy of 97%, precision of 93%, and recall of 91%, it surpasses the best rule-based method (accuracy: 85%, precision: 71%, recall: 62%) and the best machine learning-based method (accuracy: 93%, precision: 83%, recall: 79%).
更多查看译文
关键词
Network anomaly detection,Federated learning,Data privacy,Anomaly detection,Network security
AI 理解论文
溯源树
样例
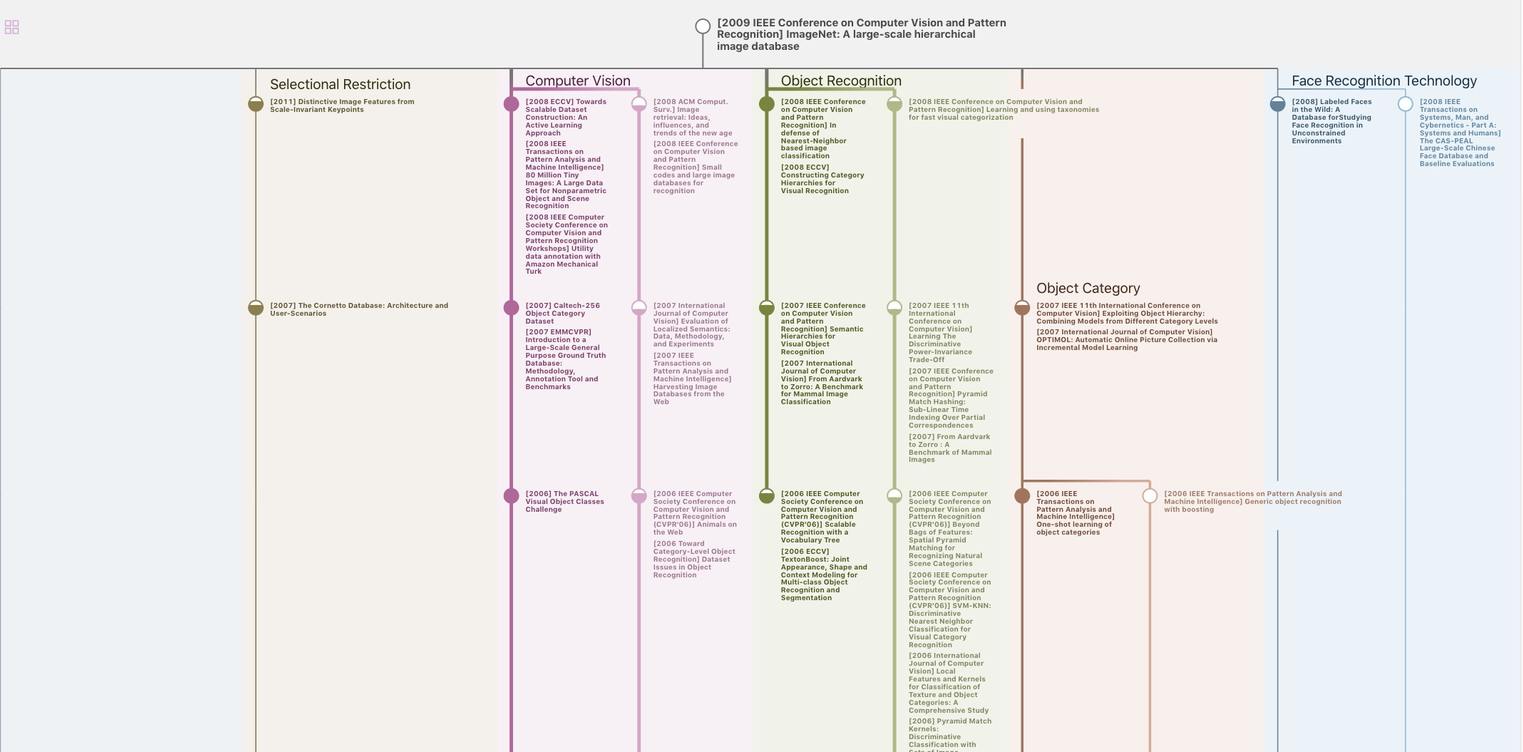
生成溯源树,研究论文发展脉络
Chat Paper
正在生成论文摘要