A Novel Data-Driven LSTM-SAF Model for Power Systems Transient Stability Assessment
IEEE TRANSACTIONS ON INDUSTRIAL INFORMATICS(2024)
摘要
Transient stability is an important metric for assessing the operational state of a power system. However, due to the inherent complexity of the power systems, it is difficult to achieve stable and precise transient stability assessment (TSA). This article proposes a novel data-driven long short-term memory with self-attention mechanism and focal loss function (LSTM-SAF) model to achieve a rapid and reliable TSA scheme. First, an improved wrapper approach involving a genetic algorithm is established to obtain concise and effective input features, which can enhance model performance and efficiency. Then, an LSTM network combined with a self-attention mechanism is developed to learn reliable TSA paradigms, in which the self-attention mechanism can further explore the information relationships of temporal features extracted from the LSTM, thereby significantly improving TSA accuracy. In addition, to resolve the lack of insufficient training related to sample imbalance, a new focal loss function is designed to guide model training. This article provides a complete TSA scheme (including offline training and online execution) that considers both assessment performance and response speed. The effectiveness of the proposed model is verified by the numerical testing results on IEEE 39 bus system, NPCC 140 bus system, IEEE 145 bus system and IEEE 300 bus system.
更多查看译文
关键词
Power system stability,Feature extraction,Long short term memory,Data models,Numerical stability,Stability criteria,Numerical models,Focal loss,long short-term memory (LSTM) network,self-attention,transient stability,wrapper approach
AI 理解论文
溯源树
样例
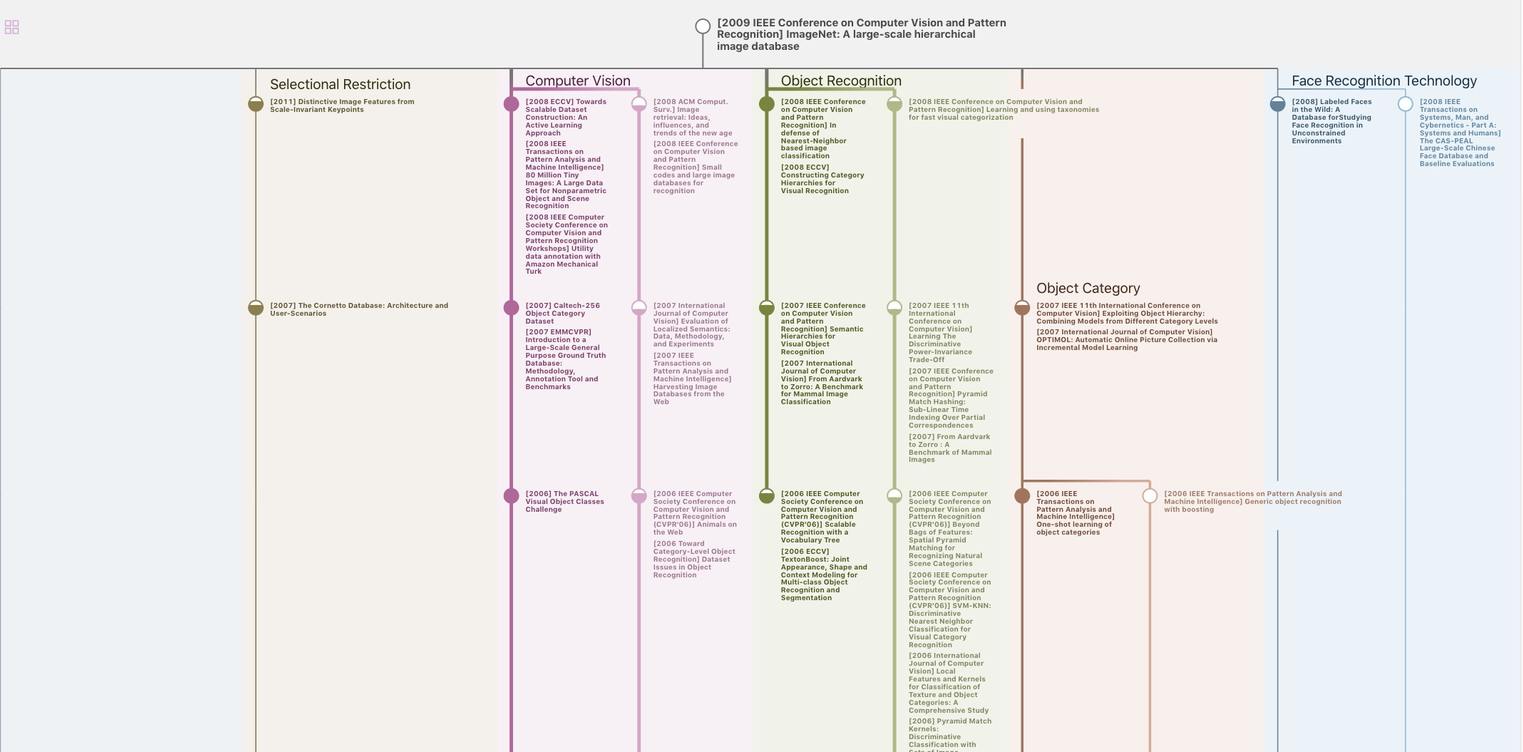
生成溯源树,研究论文发展脉络
Chat Paper
正在生成论文摘要