INSPIRATION: A reinforcement learning-based human visual perception-driven image enhancement paradigm for underwater scenes
ENGINEERING APPLICATIONS OF ARTIFICIAL INTELLIGENCE(2024)
摘要
This paper delves into enhancing underwater images to improve human observation of underwater scenes. To this end, a reinforcement learning -based human visual perception -driven image enhancement paradigm for underwater scenes is proposed, referred to as INSPIRATION. This paradigm is so named because it constructs rewards for guiding model training by incorporating metrics inspired by human visual perception, eliminating the need for reference images. It overcomes the limitations of deep models, which often result in poor performance in dynamic and diverse underwater scenes due to the scarcity of underwater images with enhancement references. Specifically, a residual -enhancement network, composed of residual modules and a channel -attention module, is utilized to extract features as a state. An extensible collection encompassing diverse image enhancement algorithms is utilized to provide an image enhancement algorithm as an action. A multi -non -reference human visual perception metric increment is utilized as a reward, and proximal policy optimization (PPO) is utilized to conduct reinforcement learning. This paradigm treats underwater images as sole inputs during both training and implementation, learning and organizing a sequence of image enhancement algorithms to explicitly achieve a step -wise image enhancement process, aligning the enhanced images with human visual perception. Extensive qualitative and quantitative evaluations conclusively demonstrate that our paradigm outperforms nine state-of-the-art underwater image enhancement methods in terms of visual quality and achieves better performance on five underwater image quality assessment measures across three underwater image datasets. Additionally, the potential advantages of our paradigm as a preprocessing step in other underwater computer vision applications are demonstrated. The code has been released publicly for reproducibility and evaluations at https://gitee.com/wanghaoupc/uie_inspiration.
更多查看译文
关键词
Underwater scene,Image enhancement,Human visual perception,Reinforcement learning
AI 理解论文
溯源树
样例
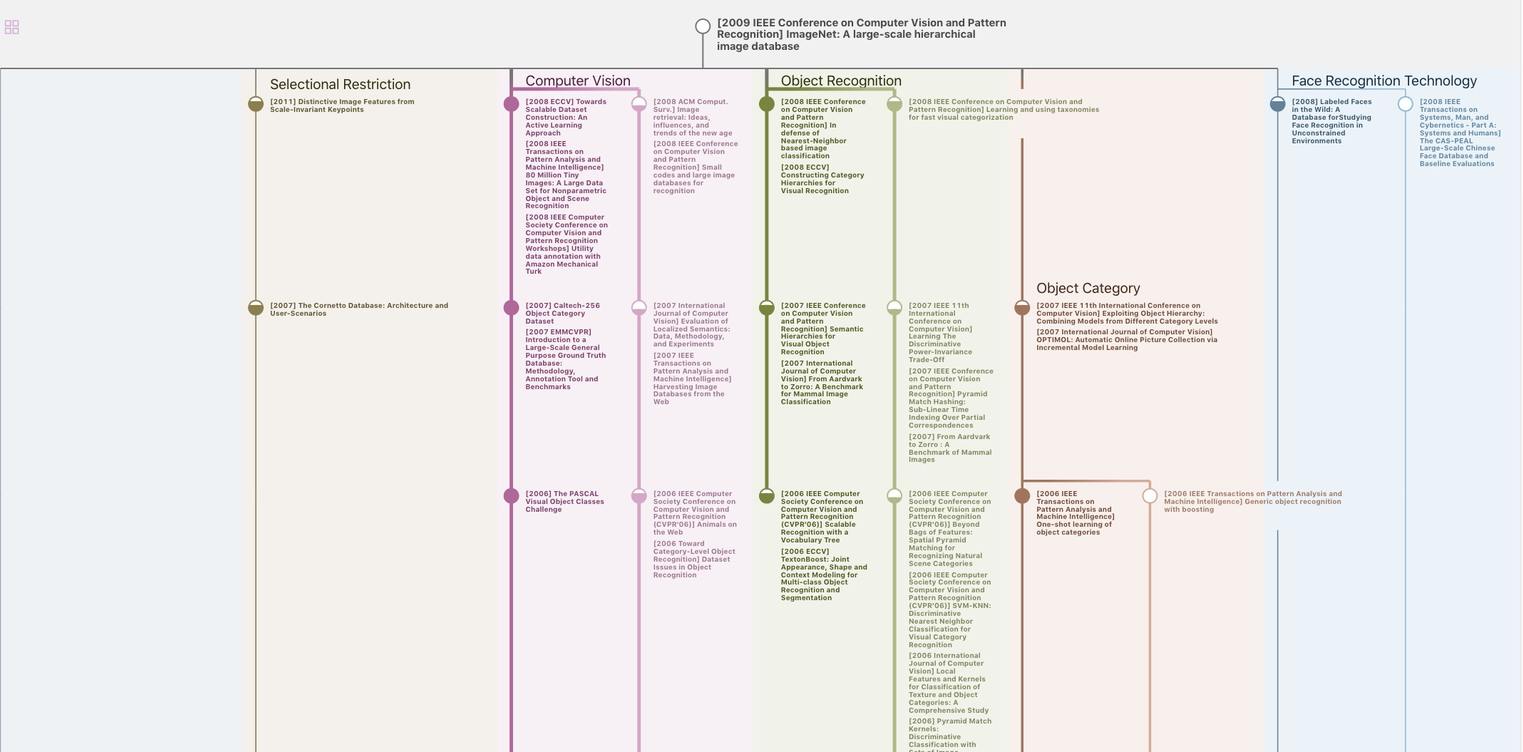
生成溯源树,研究论文发展脉络
Chat Paper
正在生成论文摘要