Empowering Unsupervised Domain Adaptation with Large-scale Pre-trained Vision-Language Models.
IEEE/CVF Winter Conference on Applications of Computer Vision(2024)
摘要
Unsupervised Domain Adaptation (UDA) aims to leverage the labeled source domain to solve the tasks on the unlabeled target domain. Traditional UDA methods face the challenge of the tradeoff between domain alignment and semantic class discriminability, especially when a large domain gap exists between the source and target domains. The efforts of applying large-scale pre-training to bridge the domain gaps remain limited. In this work, we propose that Vision-Language Models (VLMs) can empower UDA tasks due to their training pattern with language alignment and their large-scale pre-trained datasets. For example, CLIP and GLIP have shown promising zero-shot generalization in classification and detection tasks. However, directly fine-tuning these VLMs into downstream tasks may be computationally expensive and not scalable if we have multiple domains that need to be adapted. Therefore, in this work, we first study an efficient adaption of VLMs to preserve the original knowledge while maximizing its flexibility for learning new knowledge. Then, we design a domain-aware pseudo-labeling scheme tailored to VLMs for domain disentanglement. We show the superiority of the proposed methods in four UDA-classification and two UDA-detection benchmarks, with a significant improvement (+9.9%) on DomainNet.
更多查看译文
关键词
Algorithms,Machine learning architectures,formulations,and algorithms,Algorithms,Vision + language and/or other modalities
AI 理解论文
溯源树
样例
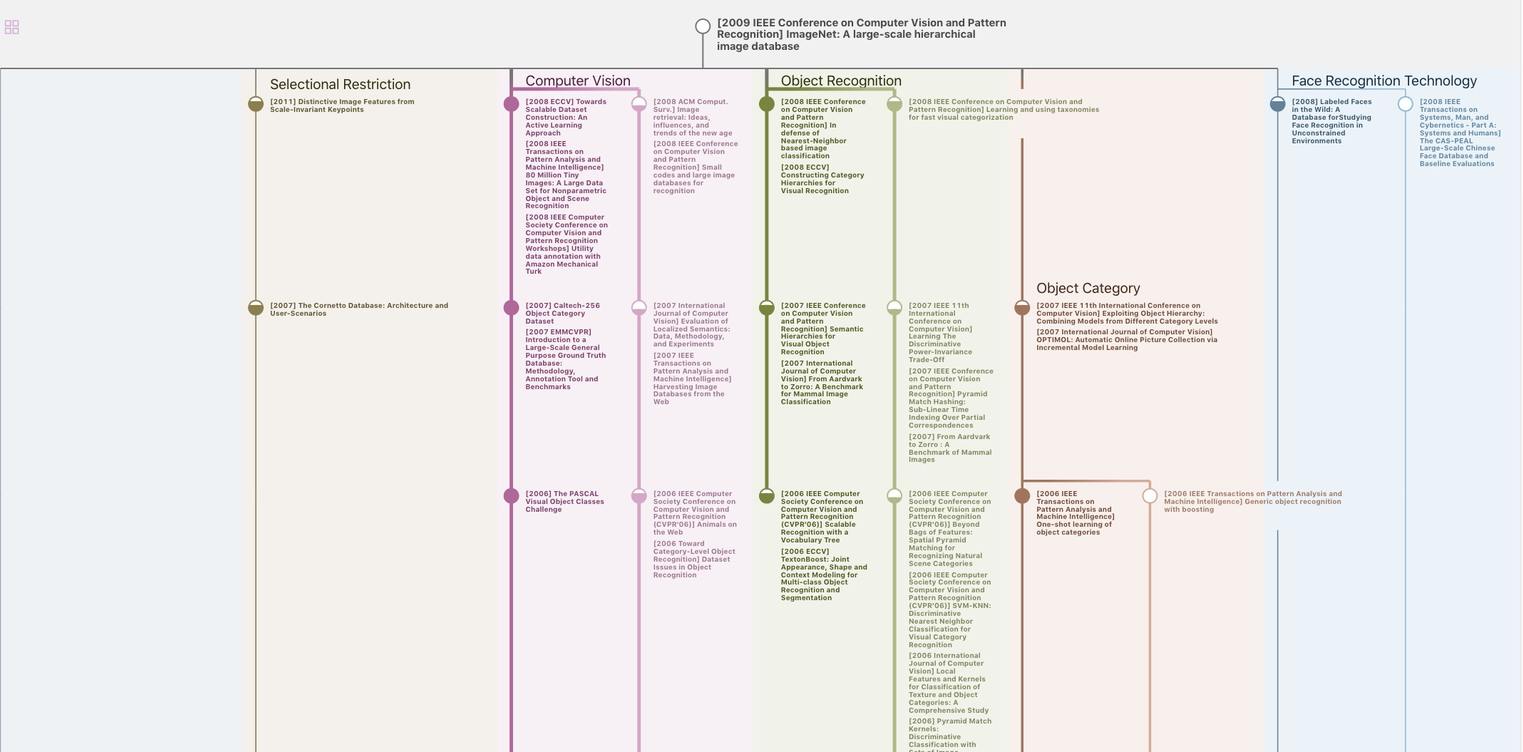
生成溯源树,研究论文发展脉络
Chat Paper
正在生成论文摘要