Rice Leaf Diseases Classification using Deep Learning Based on EfficientNetB3 Architecture with Transfer Learning
2023 International Conference on Technology, Engineering, and Computing Applications (ICTECA)(2023)
摘要
Rice is the most significant agricultural commodity and a staple among Indonesians. One of the primary issues encountered by rice farmers is the pests and diseases that attack their crops, including those that affect the leaves, ultimately decreasing the quality and quantity of rice productivity. In this research, we introduce deep learning, especially Convolutional Neural Networks, to classify rice leaf disease as a first-step approach to accelerating the determination of the appropriate treatment process. Convolutional Neural Networks (CNN) based on the EfficientNetB3 architecture can be used to directly diagnose and classify rice leaf disease image data. Because the CNN method is highly computational and uses many resources, the research proposed using the EfficientNetB3 architecture with transfer learning (pre-trained model). We use various transfer learning techniques to optimize our model’s accuracy and performance, including unfreezing a few last layers and unfreezing all layers, allowing the pre-trained model to learn from new data continually. This dataset contains 3355 healthy and three distinct varieties (brown spot, hispa, and leaf blast) of rice leaf diseases. Finally, model EfficientNetB3 with transfer learning unfreezes all layers and achieves the highest testing accuracy of 79.43% and train accuracy of 98.27% with an area under curve (AUC) of 0.9321.
更多查看译文
关键词
Image classification,Convolutional Neural Network,EfficientNetB3,rice leaf disease,transfer learning
AI 理解论文
溯源树
样例
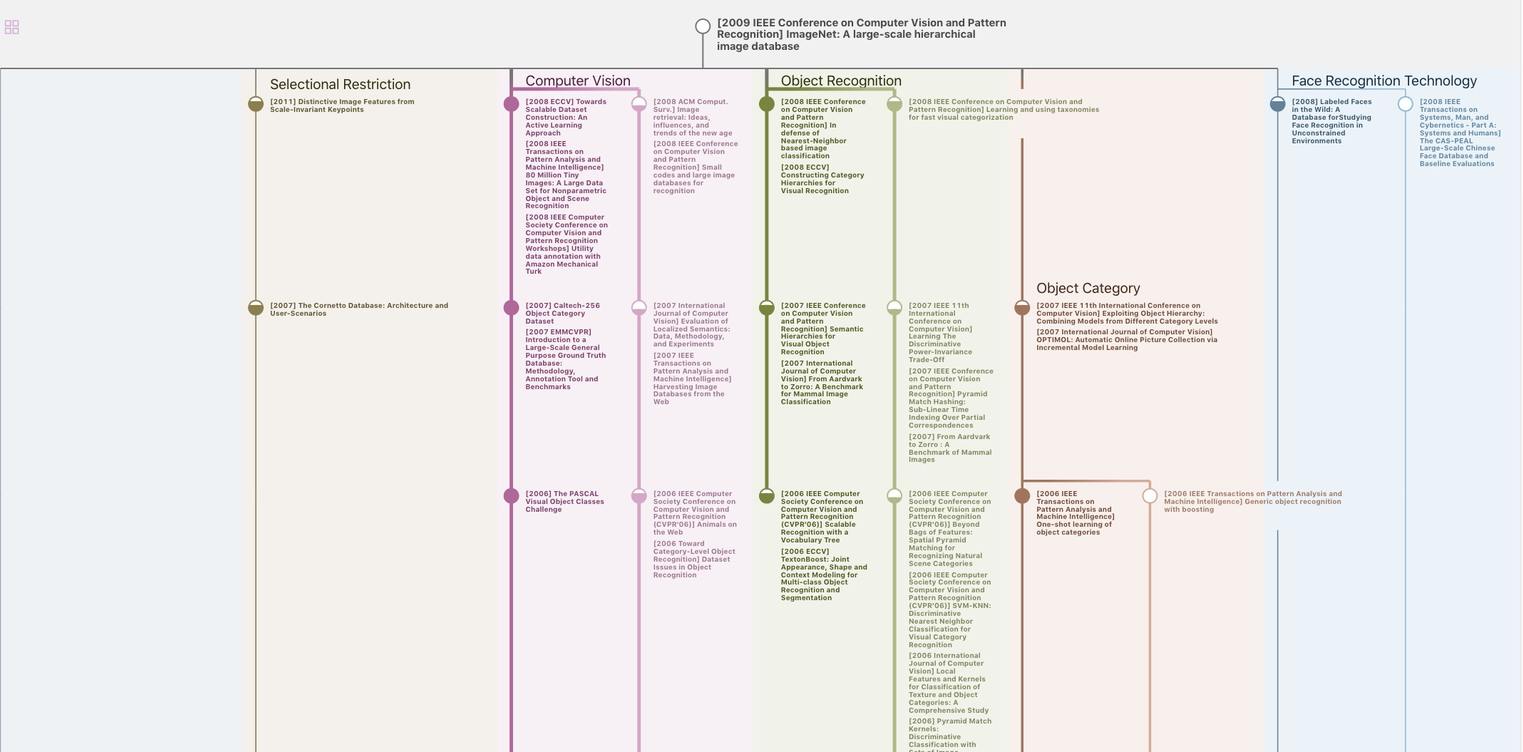
生成溯源树,研究论文发展脉络
Chat Paper
正在生成论文摘要