Automating property valuation at the macro scale of suburban level: A multi-step method based on spatial imputation techniques, machine learning and deep learning
HABITAT INTERNATIONAL(2024)
摘要
Property valuation research, evolving with Automated Valuation Models (AVMs) using Artificial Intelligence (AI) and Machine Learning (ML), encounters challenges in handling dynamic market conditions. While the market approach is a practical solution to complement the AVMs, it also suffers from different deficiencies, particularly in relying on subjective valuer judgment. In Australia 's diverse real estate market, complete and up-to-date market data derived from recent transactions of the different property types within various suburbs can be crucial for valuers. However, accessing such data often comes at a high cost, and the availability of transaction data is limited, mainly when market analysis necessitates the consideration of property valuation across various property types and bedroom counts. Accordingly, this paper presents a novel multi -step method to estimate the median prices of different property types considering their bedroom counts at the suburban level in the Melbourne Metropolitan area to benefit valuers when adopting the market approach. Nine distinct and ensembled spatially -based imputation techniques of K -Nearest Neighbors (KNN), Inverse Distance Weighted (IDW), Weighted KNN, Weighted IDW, Weighted KNN-IDW, Random Forest (RF), eXtreme Gradient Boosting (XGBoost), RF-IDW-KNN and XGBoost-IDW-KNN are first employed to impute missing data on six market -related parameters obtained from the Real Estate Institute of Victoria (REIV). These parameters include median price (with no consideration of bedroom counts), price change, median rent, rental yield, clearance rate and days on market for houses and units. Next, based on these parameters, three ML algorithms -RF, Support Vector Regression (SVR) and XGBoost -are developed to estimate the median prices. Subsequently, the Long Short -Term Memory (LSTM) technique is employed for Deep Learning (DL) -based spatiotemporal analysis, clustering suburbs based on property value fluctuations. Finally, these clusters are integrated into the ML models developed in the previous step as an auxiliary feature to assess their potential impact on enhancing price estimation accuracy. The results demonstrate promising accuracies for different property types based on different performance assessment metrics. The paper also underscores improved estimation accuracy by incorporating time series -based clustering as a supplementary parameter through transfer learning.
更多查看译文
关键词
Property valuation,Median price estimation,Machine learning,Deep learning,Spatial imputation techniques,Transfer learning
AI 理解论文
溯源树
样例
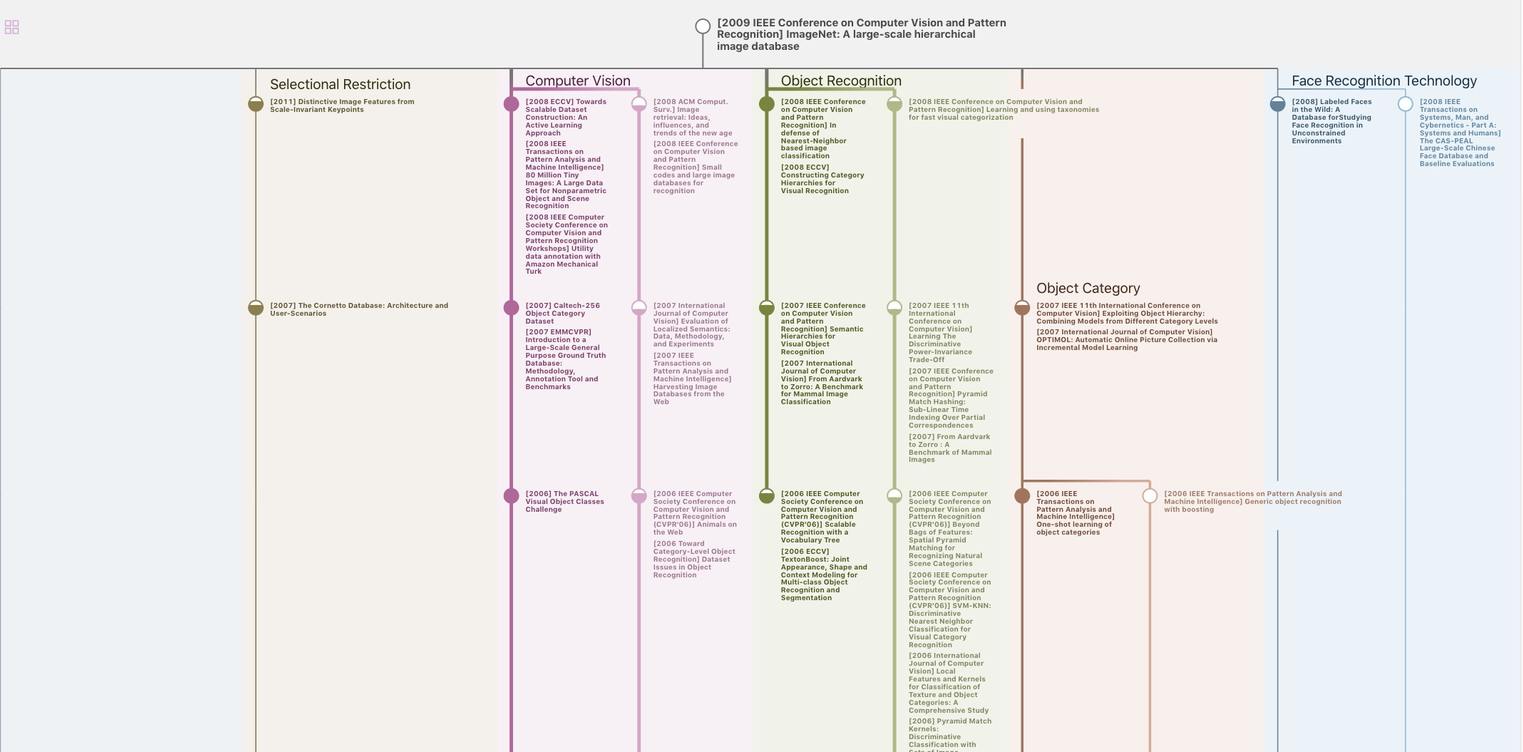
生成溯源树,研究论文发展脉络
Chat Paper
正在生成论文摘要