Federated learning-based edge computing for automatic train operation in communication-based train control systems
The Journal of Supercomputing(2024)
Abstract
Automatic train operation (ATO) is a critical component of automatic train control (ATC) systems. The ATO automatically adjusts the speed of trains, ensuring the safety of trains and increasing the passenger capacity of urban railway networks. The traditional ATO models employ a linear approximation approach to fit the nonlinear control model of the train, such as proportional–integral–derivative (PID) or model predictive control (MPC). However, due to the complexity of actual train operations, these methods fail to accurately fit train models. Thus, they are unable to shorten the operational intervals of trains and meet the demand for improving system efficiency of ATC. In recent years, machine learning-based methods have been employed for deriving more accurate train control models. However, these methods require trains to offload massive amounts of data to central servers for centralized training, which is challenging in CBTC systems due to limited wireless bandwidth. In this paper, we propose using federated learning (FL)-based edge computing to train the ATO model without transmitting a large amount of data. The onboard computing devices are used to perform local federated learning when the train is idle. Additionally, we present a Tab-Transformer-based machine learning model for ATO policy to improve the prediction accuracy of federally trained models. Our extensive simulation results demonstrate that the proposed federated learning scheme improves the efficiency of model training, and the Tab-Transformer-based ATO model achieves better driving performance.
MoreTranslated text
Key words
ATO,CBTC,Federated learning,Edge computing,Tab-Transformer
AI Read Science
Must-Reading Tree
Example
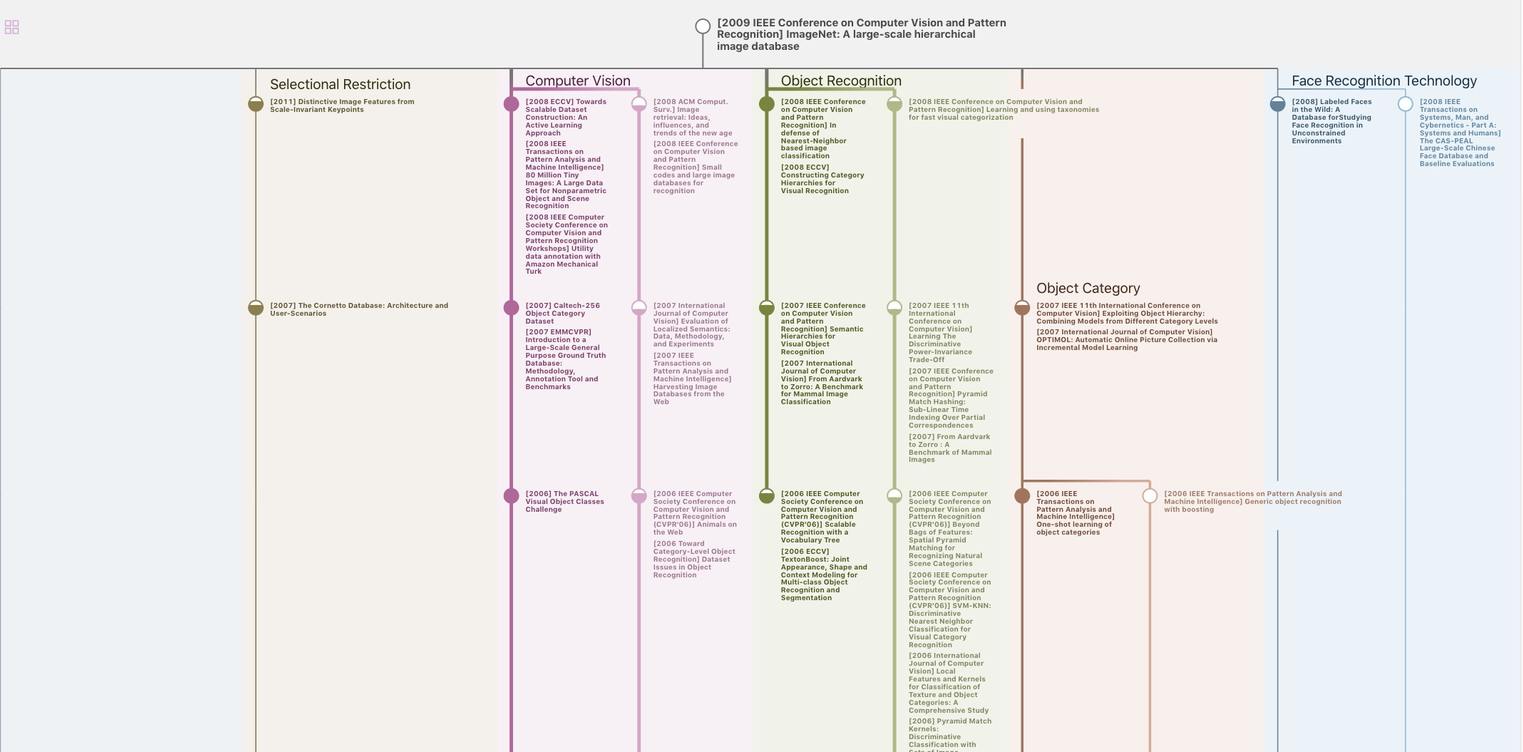
Generate MRT to find the research sequence of this paper
Chat Paper
Summary is being generated by the instructions you defined