From irregular to continuous: The deep Koopman model for time series forecasting of energy equipment
APPLIED ENERGY(2024)
Abstract
The data driven time series forecasting is a key concern for the digital modeling and health management of energy equipment. However, due to various issues, e.g., sensor failures in the data collection of energy equipment, the missing of data or the outliers often exist, thus producing irregular time series data. This limits the modeling capabilities of conventional sequence models like recurrent neural networks (RNN). To this end, we propose a continuous dynamic model based on the Koopman theory and deep learning. This model attempts to learn the latent linear and continuous Koopman space over irregular time series for performing highprecision multi -step -ahead prediction. In order to assess the performance, numerous numerical experiments have been conducted on the condition assessment of gas turbine and the spatio-temporal forecasting of multiphase flow fields in the supercritical water fluidized bed. The comparison to state-of-the-art sequence models validates the efficacy and superiority of the proposed model in handling irregular time series data from energy equipment.
MoreTranslated text
Key words
Irregular time series,Koopman operator,Recurrent neural networks,Multi-step-ahead prediction,Energy equipment
AI Read Science
Must-Reading Tree
Example
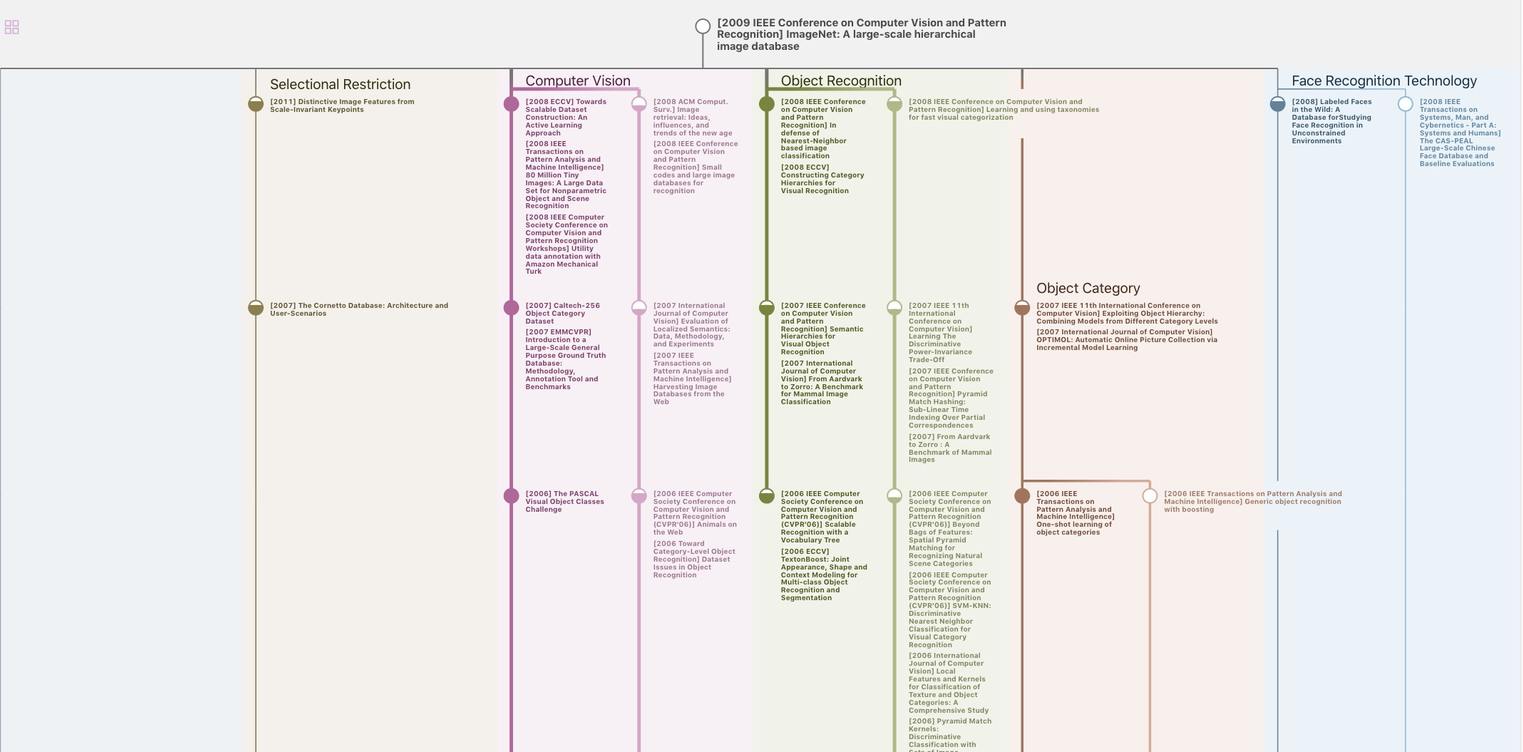
Generate MRT to find the research sequence of this paper
Chat Paper
Summary is being generated by the instructions you defined