U2D2PCB: Uncertainty-aware Unsupervised Defect Detection on PCB Images Using Reconstructive and Discriminative Models
IEEE Transactions on Instrumentation and Measurement(2024)
摘要
The defect detection of printed circuit board (PCB) images face challenges such as limited sample number, imbalanced sample types, and varying detection reliability. To address these issues, this paper proposes an uncertainty-aware unsupervised detection model on PCB images, short for U
2
D
2
PCB. The proposed method utilizes two U-Net networks to serve as the reconstructive sub-network and the discriminative sub-network, respectively. The former one reconstructs defect-free PCB images from defective PCB images, while the latter segments the defects and evaluates the defects uncertainty with the concatenated inputs of the defective and reconstructed images. The U
2
D
2
PCB model is trained in an unsupervised manner with only defectfree images embedding with multi-scale artificial defects. Experimental results on the public PCB defect dataset and DeepPCB dataset demonstrate the effectiveness of the proposed method. The mean average precision (mAP) is 99.29% on the PCB defect dataset, while it reaches 95.78% on the DeepPCB dataset. These results are competitive to those of state-of-the-art fully supervised methods. The findings of U
2
D
2
PCB highlight the potential significance of employing unsupervised learning techniques for PCB defect detection.
更多查看译文
关键词
PCB defect detection,unsupervised learning,artificial defect,uncertainty,deep ensemble
AI 理解论文
溯源树
样例
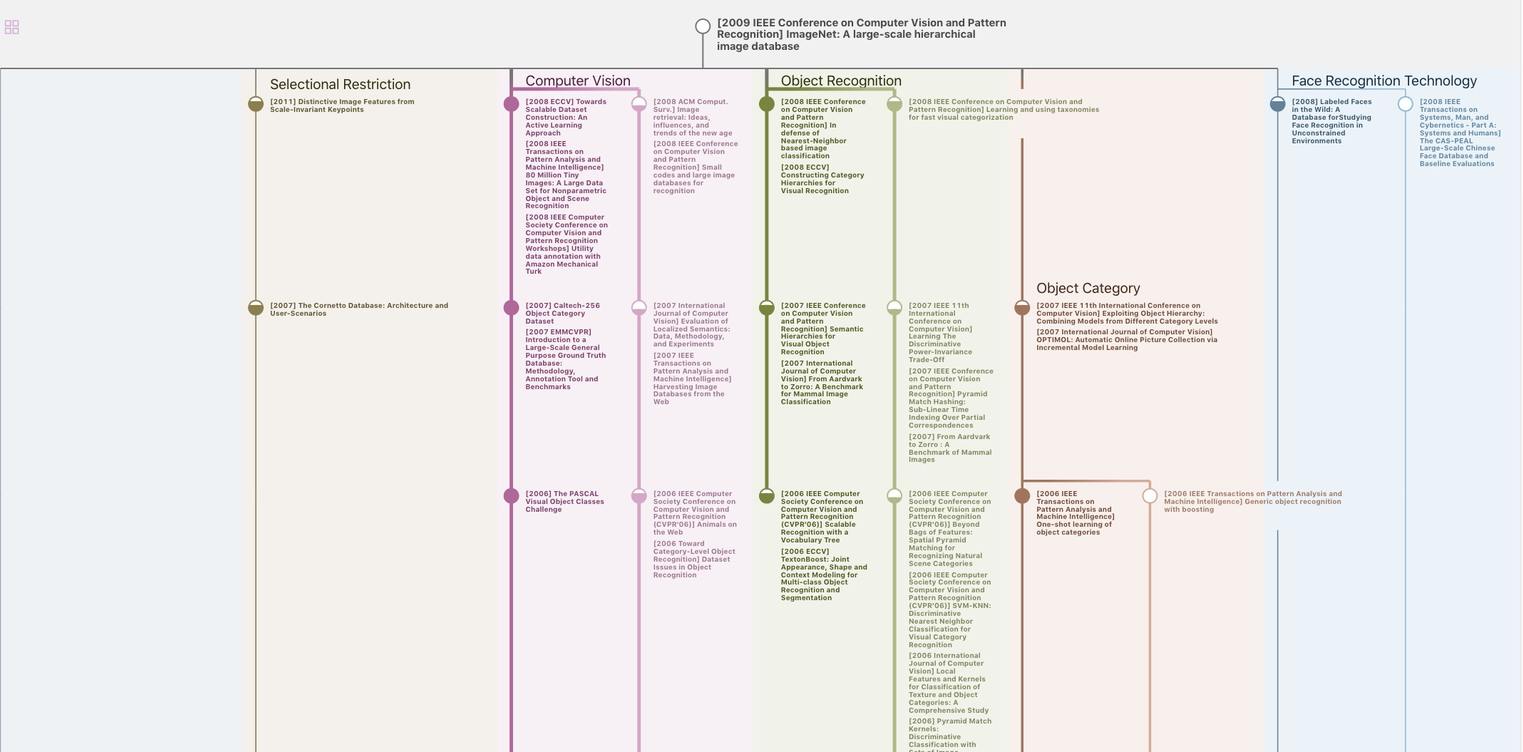
生成溯源树,研究论文发展脉络
Chat Paper
正在生成论文摘要