An Improved SAR Ship Classification Method Using Text-to-Image Generation-Based Data Augmentation and Squeeze and Excitation
REMOTE SENSING(2024)
Abstract
Synthetic aperture radar (SAR) plays a crucial role in maritime surveillance due to its capability for all-weather, all-day operation. However, SAR ship recognition faces challenges, primarily due to the imbalance and inadequacy of ship samples in publicly available datasets, along with the presence of numerous outliers. To address these issues, this paper proposes a SAR ship classification method based on text-generated images to tackle dataset imbalance. Firstly, an image generation module is introduced to augment SAR ship data. This method generates images from textual descriptions to overcome the problem of insufficient samples and the imbalance between ship categories. Secondly, given the limited information content in the black background of SAR ship images, the Tokens-to-Token Vision Transformer (T2T-ViT) is employed as the backbone network. This approach effectively combines local information on the basis of global modeling, facilitating the extraction of features from SAR images. Finally, a Squeeze-and-Excitation (SE) model is incorporated into the backbone network to enhance the network's focus on essential features, thereby improving the model's generalization ability. To assess the model's effectiveness, extensive experiments were conducted on the OpenSARShip2.0 and FUSAR-Ship datasets. The performance evaluation results indicate that the proposed method achieves higher classification accuracy in the context of imbalanced datasets compared to eight existing methods.
MoreTranslated text
Key words
SAR ship recognition,image generation,tokens-to-token vision transformers (T2T-ViT),diffusion model (DM)
AI Read Science
Must-Reading Tree
Example
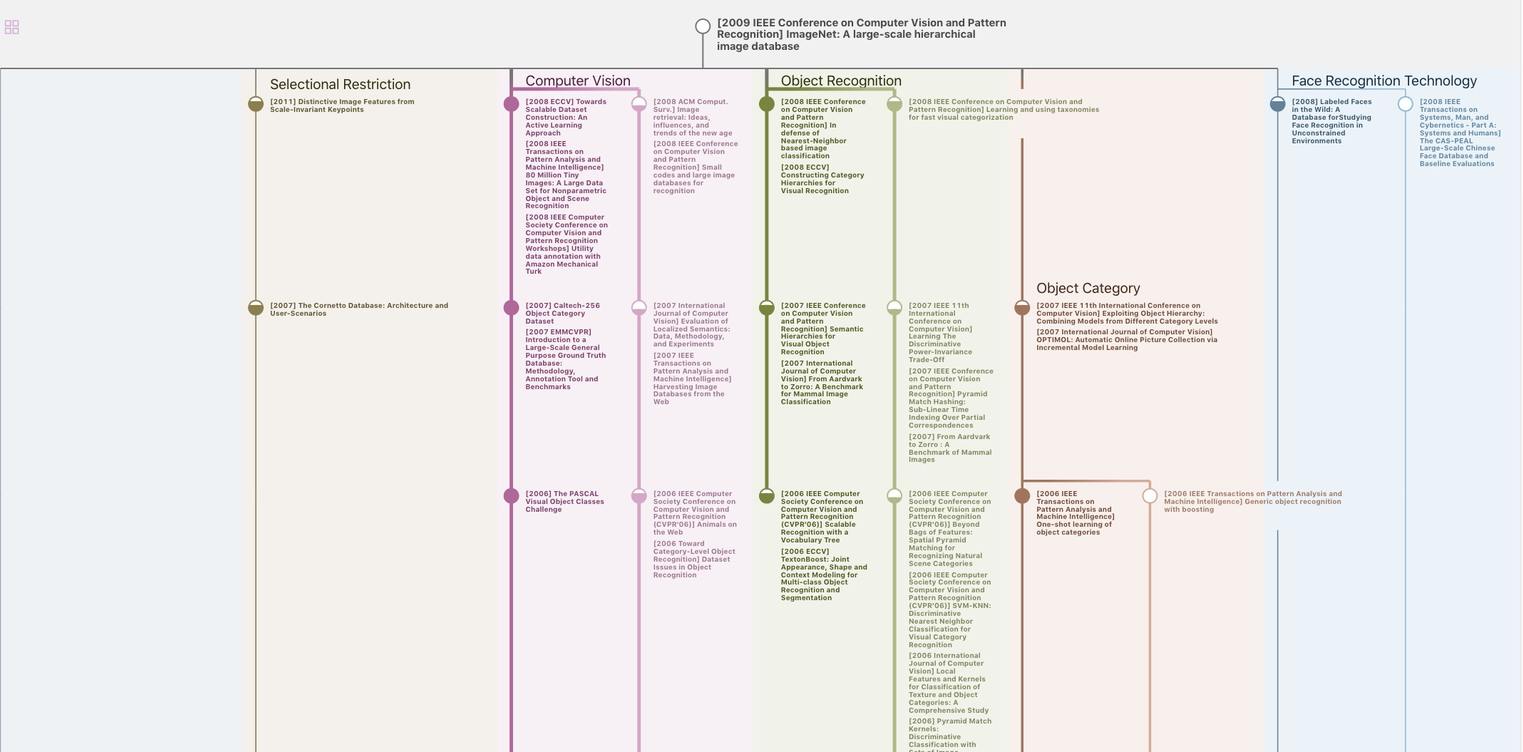
Generate MRT to find the research sequence of this paper
Chat Paper
Summary is being generated by the instructions you defined