Estimating potato above-ground biomass based on vegetation indices and texture features constructed from sensitive bands of UAV hyperspectral imagery
Computers and Electronics in Agriculture(2024)
摘要
Above-ground biomass (AGB) estimation is critical for monitoring crop growth and assessing yields. Unmanned aerial vehicle (UAV) optical remote sensing technology offers robust support for crop AGB estimation through vegetation indices (VIs). However, under conditions of high nitrogen or high AGB, most VIs lose their response to the presence of a dense plant canopy. To address the inaccuracy of estimating crop multistage AGB based on VIs formed in two bands, a UAV imaging hyperspectral experiment was conducted on potato test plots with different varieties, planting densities, and fertilizer application gradients. The ratio vegetation index (RVI) and normalized difference vegetation index (NDVI) were initially modified using a lambda-by-lambda optimization algorithm, which yielded the optimized RVI (Opt-RVI) and NDVI (Opt-NDVI). Their performances were compared with published VIs. Then, the corresponding bands from optimized VIs were used to extract textural features and their estimation performances were evaluated. Finally, based on published VIs, Opt-VIs, textures of sensitive bands, and Opt-VIs combined with the textures, the potato multistage AGB was estimated by optimized random forest (RF) regression. The RF estimation model was simplified according to variable importance scores. Results showed the spectral regions sensitive to AGB were mainly located in the red-edge range. The wavelengths of Opt-RVI and Opt-NDVI that exhibited the strongest correlation with AGB were 734 and 742 nm. Except for the normalized red-edge index formed by two red-edge bands, the published VIs (R2 = 0.07–0.28) demonstrated saturation in the context of high AGB. The optimized Opt-RVI and Opt-NDVI suppressed this phenomenon, such that R2 values for both reached 0.44 for estimating AGB. The R2 values of textural features relative to AGB for both bands ranged from 0.06 and 0.31, and CON734, COR734, DIS742, ENT742, SEC742, and COR742 exhibited the strongest correlations with AGB (R2 > 0.2). The performance of estimating potato AGB by a single indicator was sensitive texture features, Opt-VIs and published VIs from high to low and the estimation capacities of these models were limited. The combination of Opt-VIs and textural features of sensitive bands demonstrated the greatest estimation accuracy (R2 = 0.62 and RMSE = 293.08 kg/hm2). Based on variable importance scores, the accuracy of the simplified RF estimation model (four variables: Opt-RVI, CON734, ENT742, COR742) slightly decreased (R2 = 0.59 and RMSE = 301.01 kg/hm2), but model complexity was reduced and computational efficiency was improved. The simplified model accuracy was mainly affected by potato variety, fertilizer gradient, and growth stage, but not by planting density. The results of this study can be used as a reference for potato growth monitoring in the field.
更多查看译文
关键词
UAV,Potato,AGB,Hyperspectral images,RF
AI 理解论文
溯源树
样例
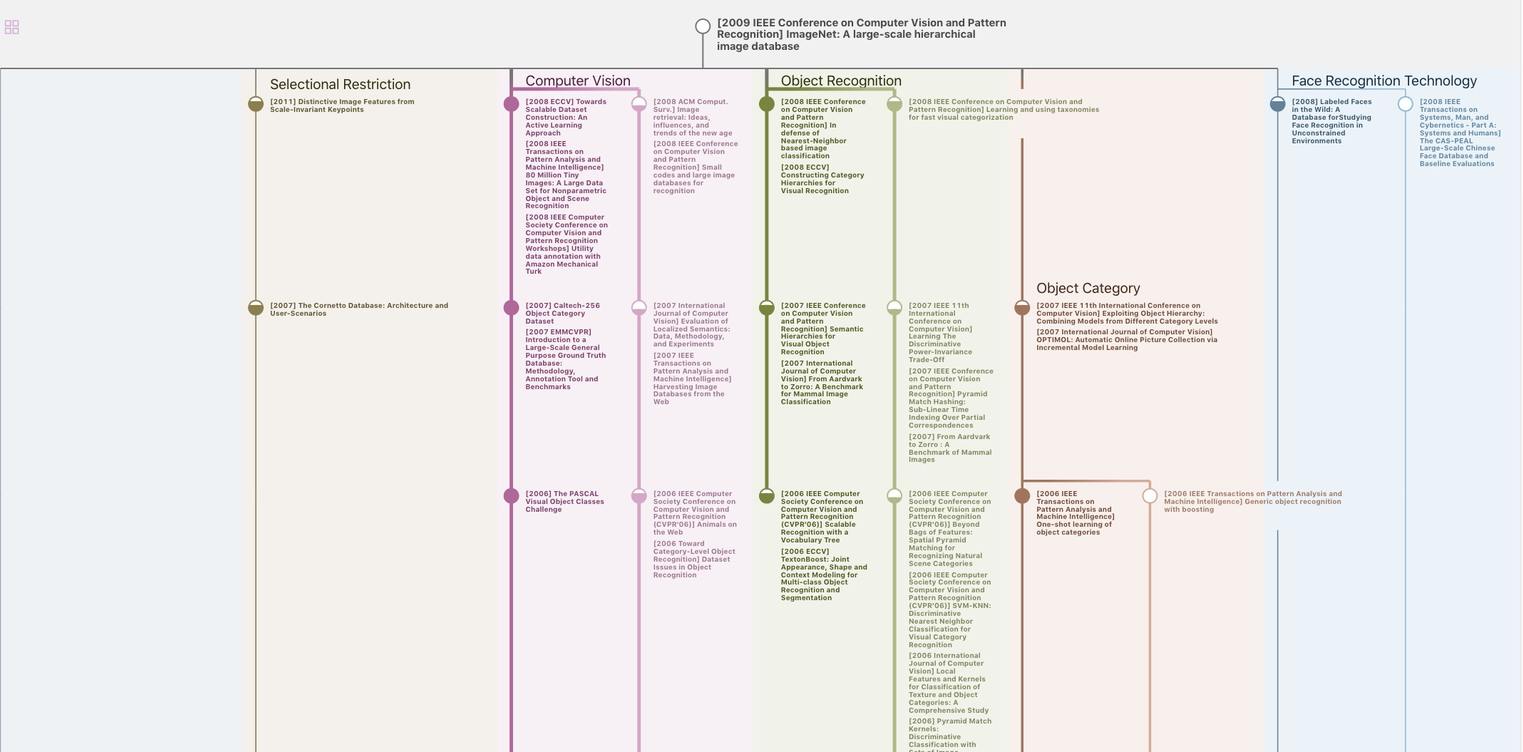
生成溯源树,研究论文发展脉络
Chat Paper
正在生成论文摘要