Evaluating feasibility of lower extremity joint moments predicted by an artificial intelligence model during walking in patients with cerebral palsy
crossref(2024)
摘要
Abstract Several studies have highlighted the advantages of employing artificial intelligence (AI) models in gait analysis. However, the practicality of AI models into clinical gait routines remains uncertain. In this study, we propose a three-step approach to evaluate the feasibility of a previously developed AI model. This model predicts joint moments during walking for 622 patients with cerebral palsy using joint kinematics as the input. Root mean square errors between lab-measured and predicted joint moments are labeled as Green (acceptable), Yellow (acceptable with caution), or Red (unacceptable). Kinematics are classified accordingly, and statistical analyses determine their impact on the AI model output. A linear discrimination analysis (LDA) model predicts labels for newly predicted joint moments based on kinematics. The knee moment showed the largest Green label population (73%), while the ankle moment has the smallest (34%). Gait profile score show significant differences between all labels except the Green vs Red for the ankle joint. The LDA model achieves75% accuracy for knee joint moment prediction, with a Green sensitivity of 94.7%. Severer patient’s condition leads to increase in Red population. While the AI model shows promise for predicting knee and hip moments, further development is necessary before its integration into clinical routines.
更多查看译文
AI 理解论文
溯源树
样例
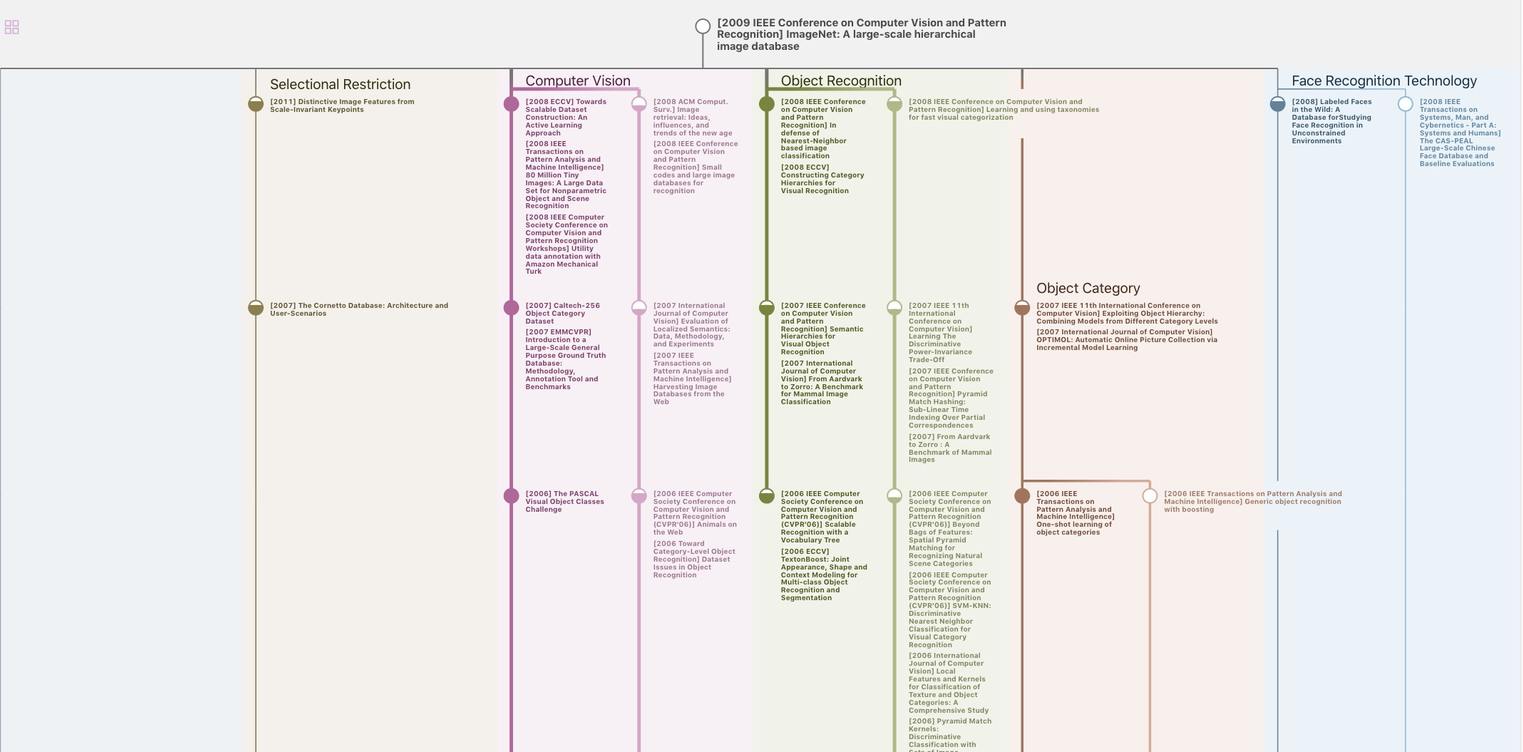
生成溯源树,研究论文发展脉络
Chat Paper
正在生成论文摘要