A Framework of Decentralized Federated Learning with Soft Clustering and 1-Bit Compressed Sensing for Vehicular Networks
IEEE INTERNET OF THINGS JOURNAL(2024)
Key words
1-bit compressed sensing,communication overhead,federated learning (FL),Internet of Vehicles (IoV),soft clustering
AI Read Science
Must-Reading Tree
Example
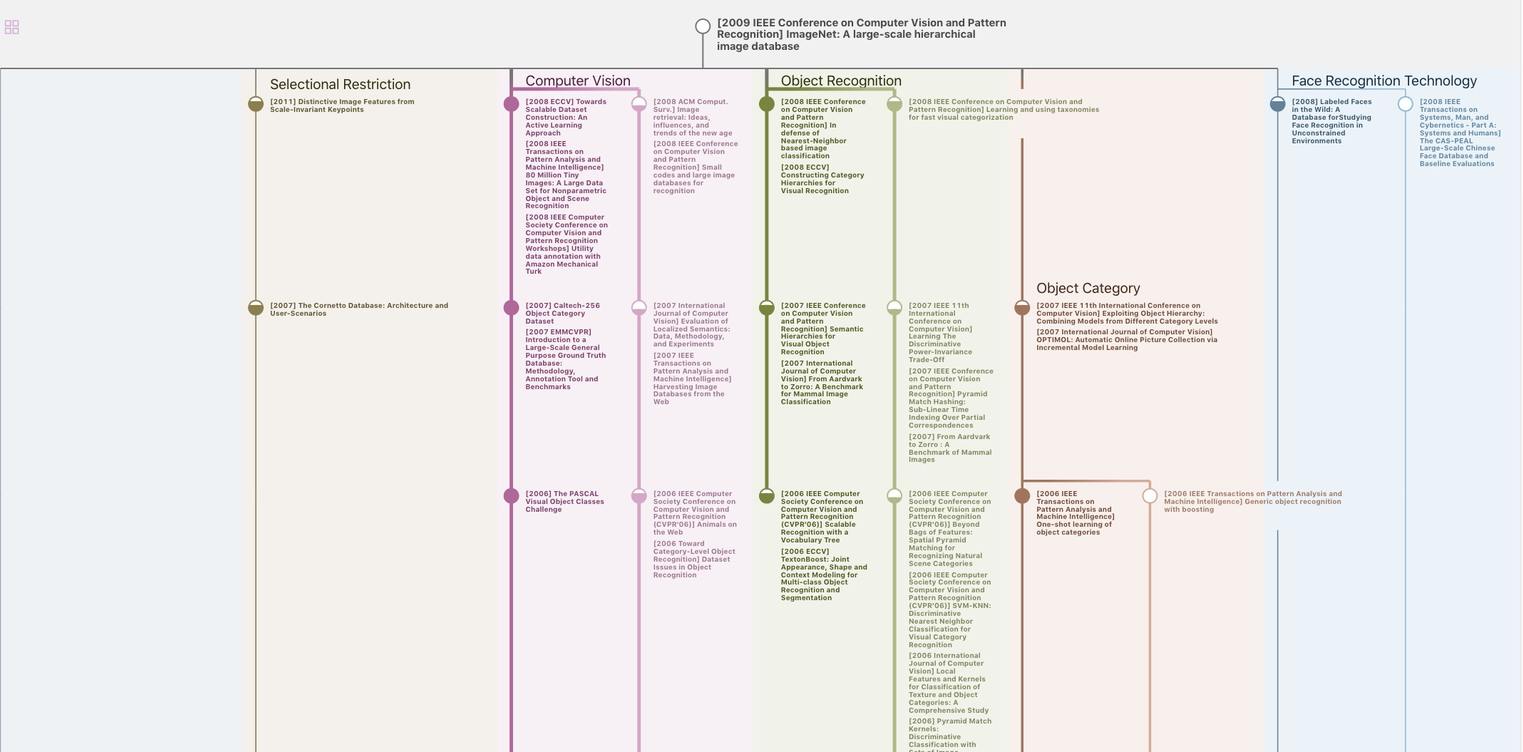
Generate MRT to find the research sequence of this paper
Chat Paper
Summary is being generated by the instructions you defined