An Objective Space Constraint-Based Evolutionary Method for High-Dimensional Feature Selection
IEEE COMPUTATIONAL INTELLIGENCE MAGAZINE(2024)
摘要
Evolutionary algorithms (EAs) have shown their competitiveness in solving the problem of feature selection. However, limited by their encoding scheme, most of them face the challenge of "curse of dimensionality". To address the issue, in this paper, an objective space constraint-based evolutionary algorithm, named OSC-EA, is proposed for high-dimensional feature selection (HDFS). Although the decision space of EAs for HDFS is very huge, its objective space is the same as that of the low-dimensional feature selection. Based on this fact, in the proposed OSC-EA, the HDFS is firstly modeled as a constrained problem, where a constraint of the objective space is introduced and used to partition the whole objective space into the "feasible region" and the "infeasible region". To handle the constrained problem, a two-stage epsilon constraint-based evolutionary scheme is designed. In the first stage, the value of epsilon is set to be very small, which ensures that the search concentrates on the "feasible region", and the latent high-quality feature subsets can be found quickly. Then, in the second stage, the value of epsilon increases gradually, so that more solutions in the "infeasible region" are considered. Until the end of the scheme, epsilon -> infinity; all the solutions in the objective space are considered. By using the search in the second stage, the quality of the obtained feature subsets is further improved. The empirical results on different high-dimensional datasets demonstrate the effectiveness and efficiency of the proposed OSC-EA.
更多查看译文
关键词
Evolutionary computation,Encoding,Feature extraction,Constraint handling
AI 理解论文
溯源树
样例
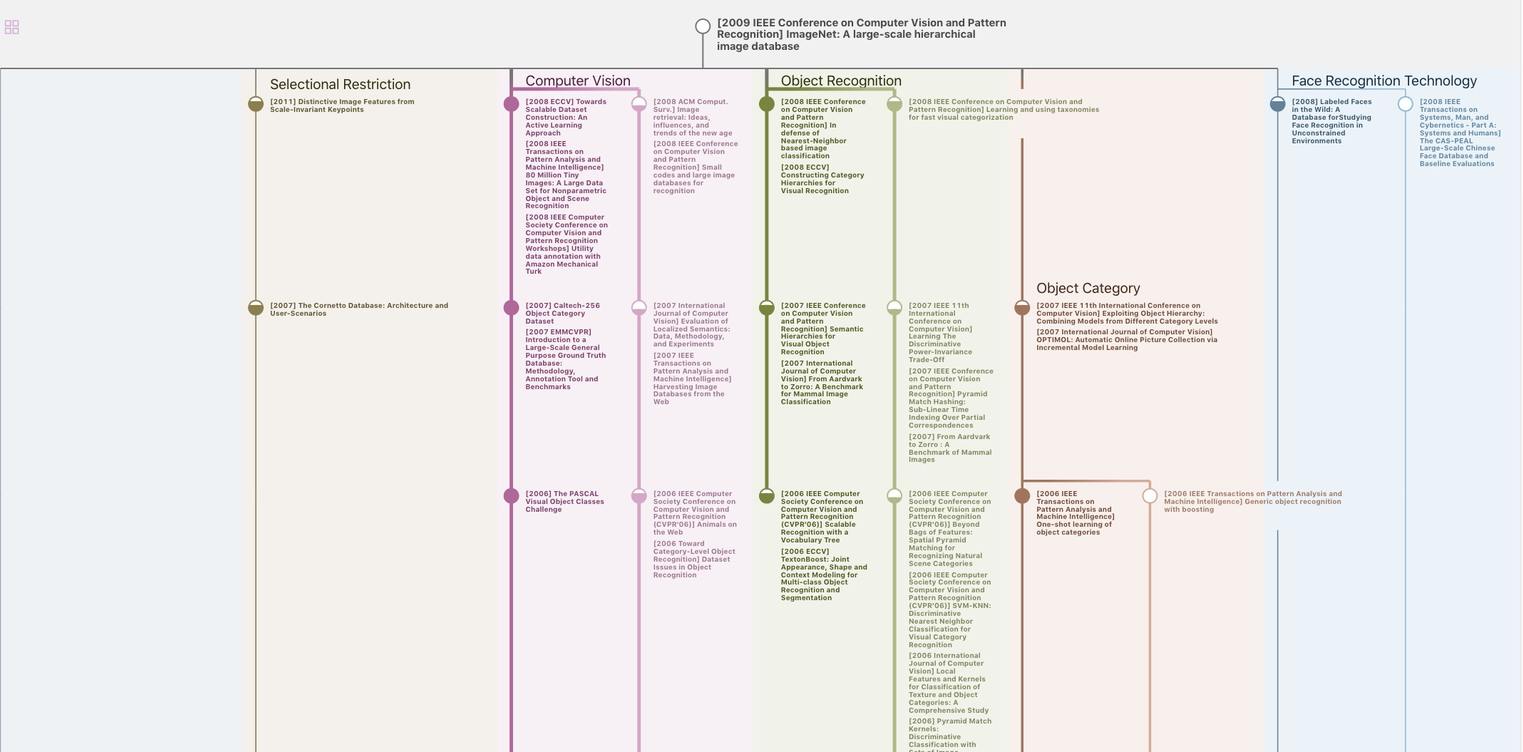
生成溯源树,研究论文发展脉络
Chat Paper
正在生成论文摘要