FPL+: Filtered Pseudo Label-based Unsupervised Cross-Modality Adaptation for 3D Medical Image Segmentation
IEEE transactions on medical imaging(2024)
摘要
Adapting a medical image segmentation model to a new domain is important forimproving its cross-domain transferability, and due to the expensive annotationprocess, Unsupervised Domain Adaptation (UDA) is appealing where only unlabeledimages are needed for the adaptation. Existing UDA methods are mainly based onimage or feature alignment with adversarial training for regularization, andthey are limited by insufficient supervision in the target domain. In thispaper, we propose an enhanced Filtered Pseudo Label (FPL+)-based UDA method for3D medical image segmentation. It first uses cross-domain data augmentation totranslate labeled images in the source domain to a dual-domain training setconsisting of a pseudo source-domain set and a pseudo target-domain set. Toleverage the dual-domain augmented images to train a pseudo label generator,domain-specific batch normalization layers are used to deal with the domainshift while learning the domain-invariant structure features, generatinghigh-quality pseudo labels for target-domain images. We then combine labeledsource-domain images and target-domain images with pseudo labels to train afinal segmentor, where image-level weighting based on uncertainty estimationand pixel-level weighting based on dual-domain consensus are proposed tomitigate the adverse effect of noisy pseudo labels. Experiments on three publicmulti-modal datasets for Vestibular Schwannoma, brain tumor and whole heartsegmentation show that our method surpassed ten state-of-the-art UDA methods,and it even achieved better results than fully supervised learning in thetarget domain in some cases.
更多查看译文
关键词
Domain adaption,image translation,uncertainty,brain tumor,pseudo labels
AI 理解论文
溯源树
样例
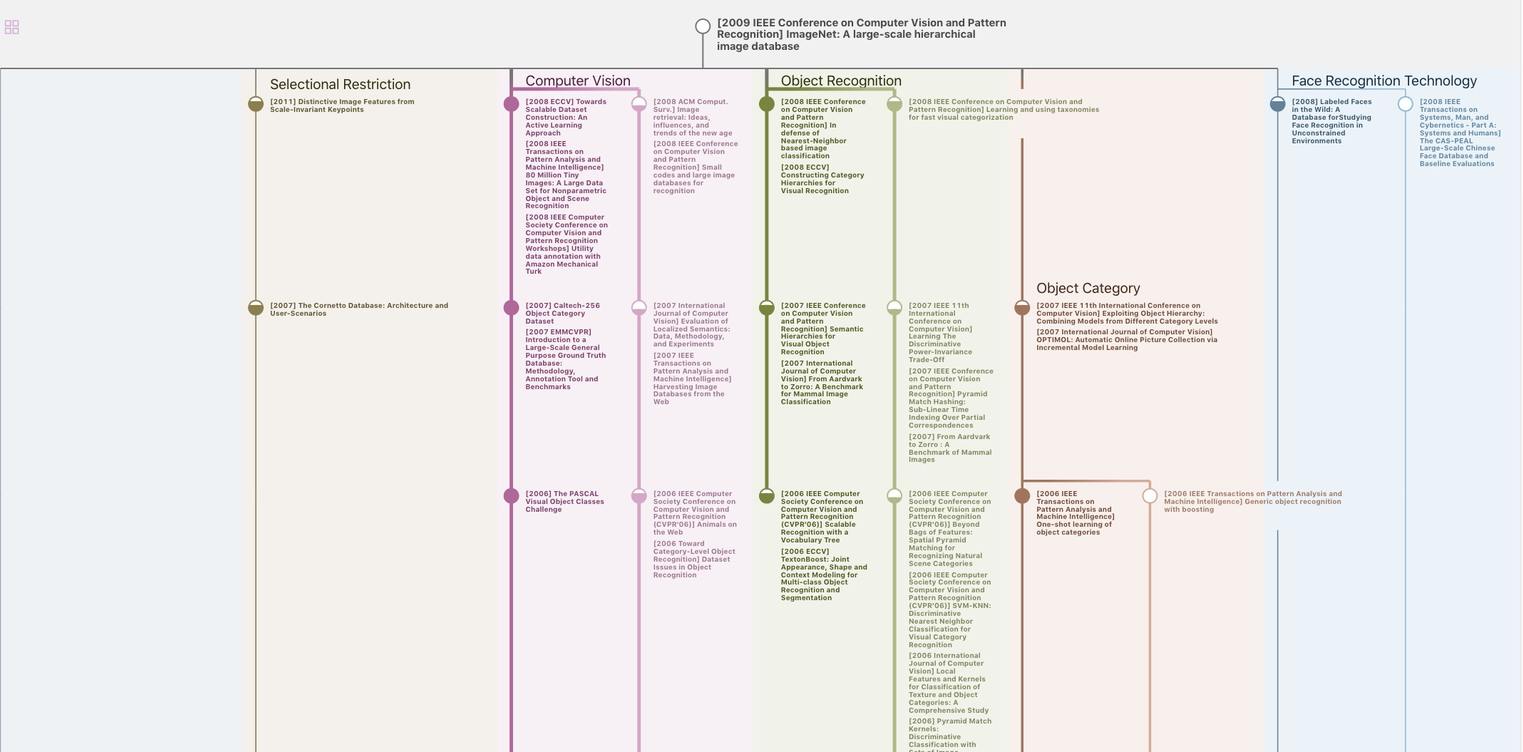
生成溯源树,研究论文发展脉络
Chat Paper
正在生成论文摘要