Optimality of Decentralized Symmetric Policies for Stochastic Teams with Mean-Field Information Sharing
arxiv(2024)
摘要
We study a class of stochastic exchangeable teams comprising a finite number
of decision makers (DMs) as well as their mean-field limits involving infinite
numbers of DMs. In the finite population regime, we study exchangeable teams
under the centralized information structure (IS). For the infinite population
setting, we study exchangeable teams under the decentralized mean-field
information sharing. The paper makes the following main contributions: i) For
finite population exchangeable teams, we establish the existence of a
randomized optimal policy that is exchangeable (permutation invariant) and
Markovian. This optimal policy is obtained via value iterations for an
equivalent measure-valued controlled Markov decision problem (MDP); ii) We show
that a sequence of exchangeable optimal policies for a finite population
setting converges to a conditionally symmetric (identical), independent, and
decentralized randomized policy for the infinite population problem. This
result establishes the existence of a symmetric, independent, decentralized
optimal randomized policy for the infinite population problem. Additionally, it
proves the optimality of the limiting measure-valued MDP for the representative
DM; iii) Finally, we show that symmetric, independent, decentralized optimal
randomized policies are approximately optimal for the corresponding
finite-population team with a large number of DMs under the centralized IS.
更多查看译文
AI 理解论文
溯源树
样例
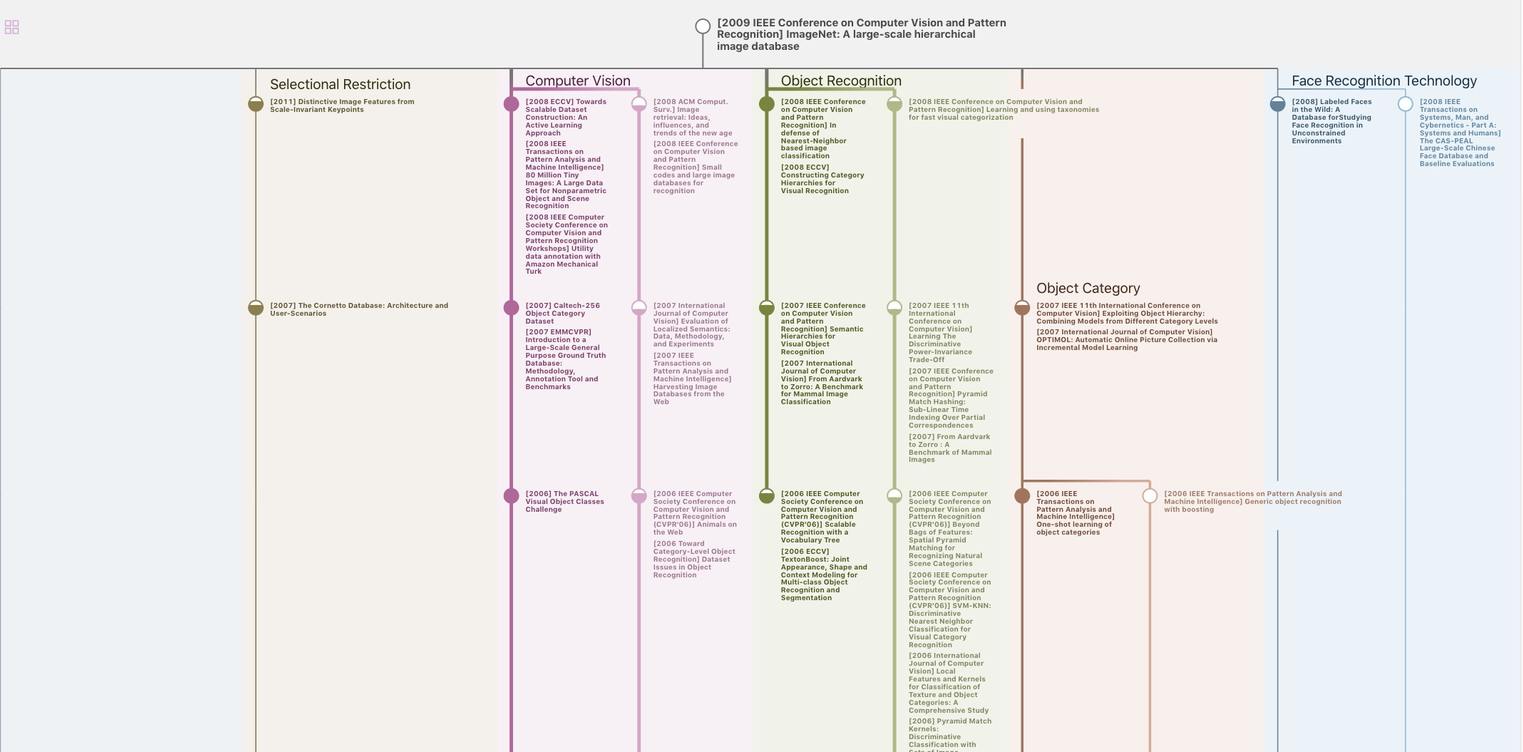
生成溯源树,研究论文发展脉络
Chat Paper
正在生成论文摘要