Graph Neural Network Meets Multi-Agent Reinforcement Learning: Fundamentals, Applications, and Future Directions
CoRR(2024)
摘要
Multi-agent reinforcement learning (MARL) has become a fundamental component
of next-generation wireless communication systems. Theoretically, although MARL
has the advantages of low computational complexity and fast convergence rate,
there exist several challenges including partial observability, non-stationary,
and scalability. In this article, we investigate a novel MARL with graph neural
network-aided communication (GNNComm-MARL) to address the aforementioned
challenges by making use of graph attention networks to effectively sample
neighborhoods and selectively aggregate messages. Furthermore, we thoroughly
study the architecture of GNNComm-MARL and present a systematic design
solution. We then present the typical applications of GNNComm-MARL from two
aspects: resource allocation and mobility management. The results obtained
unveil that GNNComm-MARL can achieve better performance with lower
communication overhead compared to conventional communication schemes. Finally,
several important research directions regarding GNNComm-MARL are presented to
facilitate further investigation.
更多查看译文
AI 理解论文
溯源树
样例
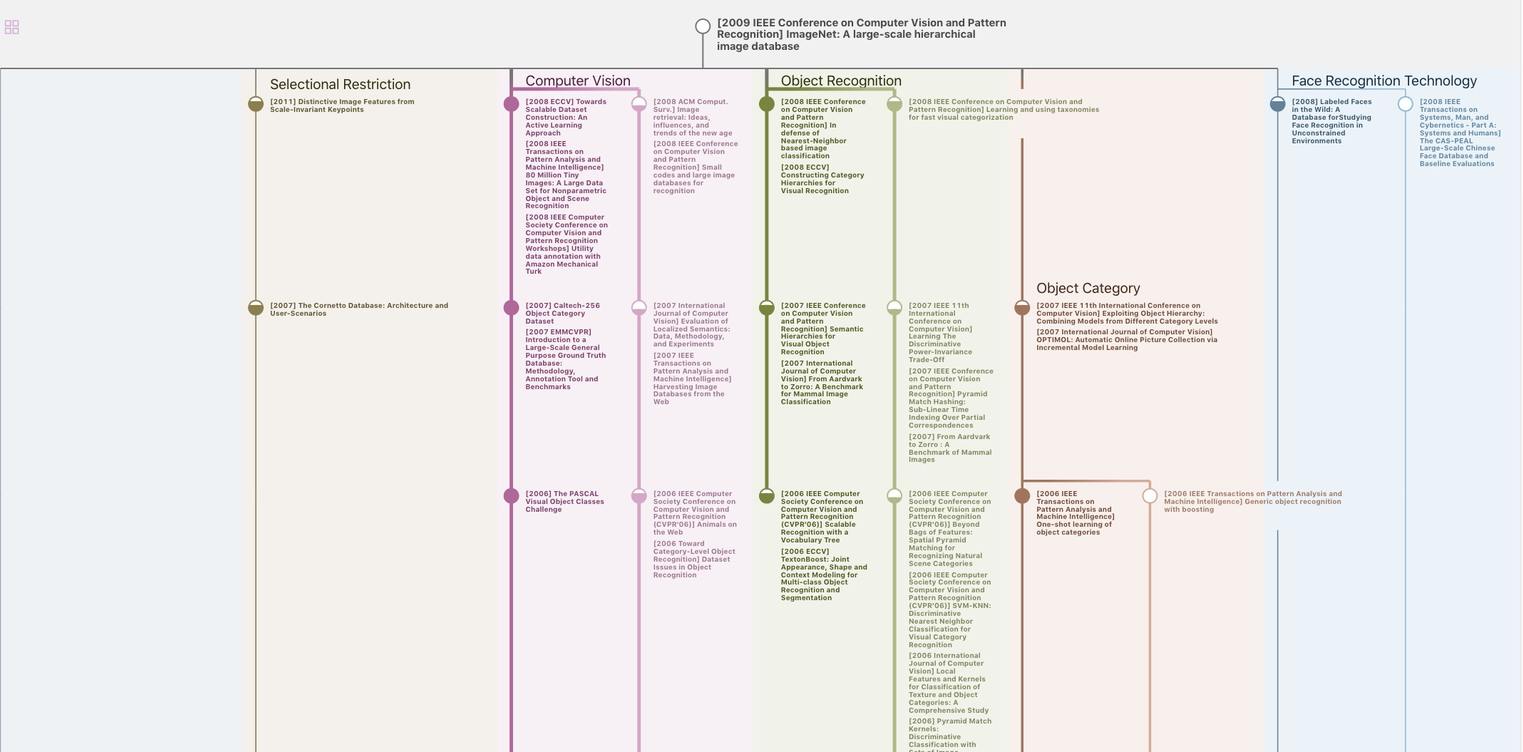
生成溯源树,研究论文发展脉络
Chat Paper
正在生成论文摘要