Unsupervised machine learning for supercooled liquids
arxiv(2024)
摘要
Unraveling dynamic heterogeneity in supercooled liquids from structural
information is one of the grand challenges of physics. In this work, we
introduce an unsupervised machine learning approach based on a time-lagged
autoencoder (TAE) to elucidate the effect of structural features on the
long-term dynamics of supercooled liquids. The TAE uses an autoencoder to
reconstruct features at time t + Δ t from input features at time t for
individual particles, and the resulting latent space variables are considered
as order parameters. In the Kob-Andersen system, with a Δ t about a
thousand times smaller than the relaxation time, the TAE order parameter
exhibits a remarkable correlation with the long-time propensity. We find that
short-range radial features correlate with the short-time dynamics, and
medium-range radial features correlate with the long-time dynamics. This shows
that fluctuations of medium-range structural features contain sufficient
information about the long-time dynamic heterogeneity, consistent with some
theoretical predictions.
更多查看译文
AI 理解论文
溯源树
样例
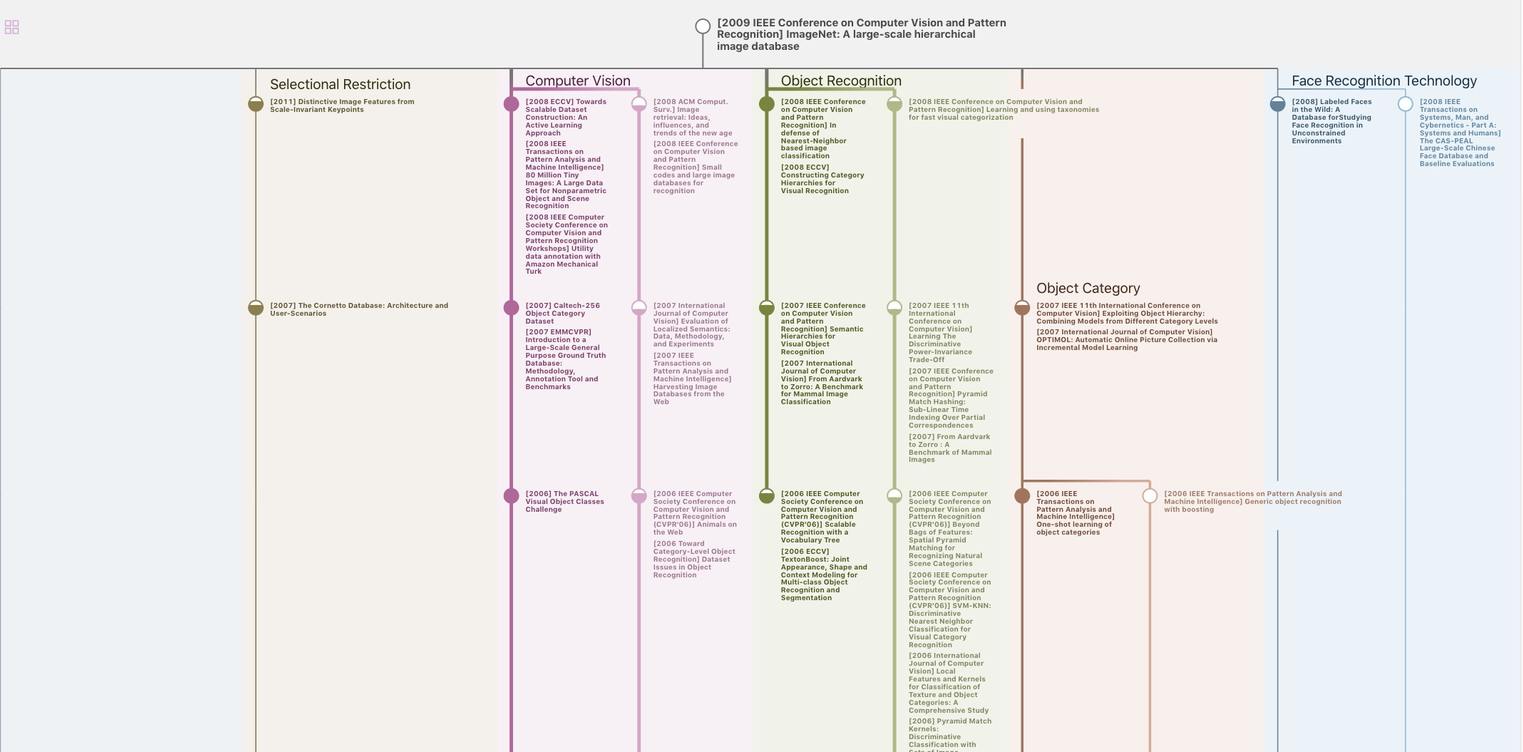
生成溯源树,研究论文发展脉络
Chat Paper
正在生成论文摘要