Gray-Box Nonlinear Feedback Optimization
arxiv(2024)
摘要
Feedback optimization enables autonomous optimality seeking of a dynamical
system through its closed-loop interconnection with iterative optimization
algorithms. Among various iteration structures, model-based approaches require
the input-output sensitivity of the system to construct gradients, whereas
model-free approaches bypass this need by estimating gradients from real-time
evaluations of the objective. These approaches own complementary benefits in
sample efficiency and accuracy against model mismatch, i.e., errors of
sensitivities. To achieve the best of both worlds, we propose gray-box feedback
optimization controllers, featuring systematic incorporation of approximate
sensitivities into model-free updates via adaptive convex combination. We
quantify conditions on the accuracy of the sensitivities that render the
gray-box approach preferable. We elucidate how the closed-loop performance is
determined by the number of iterations, the problem dimension, and the
cumulative effect of inaccurate sensitivities. The proposed controller
contributes to a balanced closed-loop behavior, which retains provable sample
efficiency and optimality guarantees for nonconvex problems. We further develop
a running gray-box controller to handle constrained time-varying problems with
changing objectives and steady-state maps.
更多查看译文
AI 理解论文
溯源树
样例
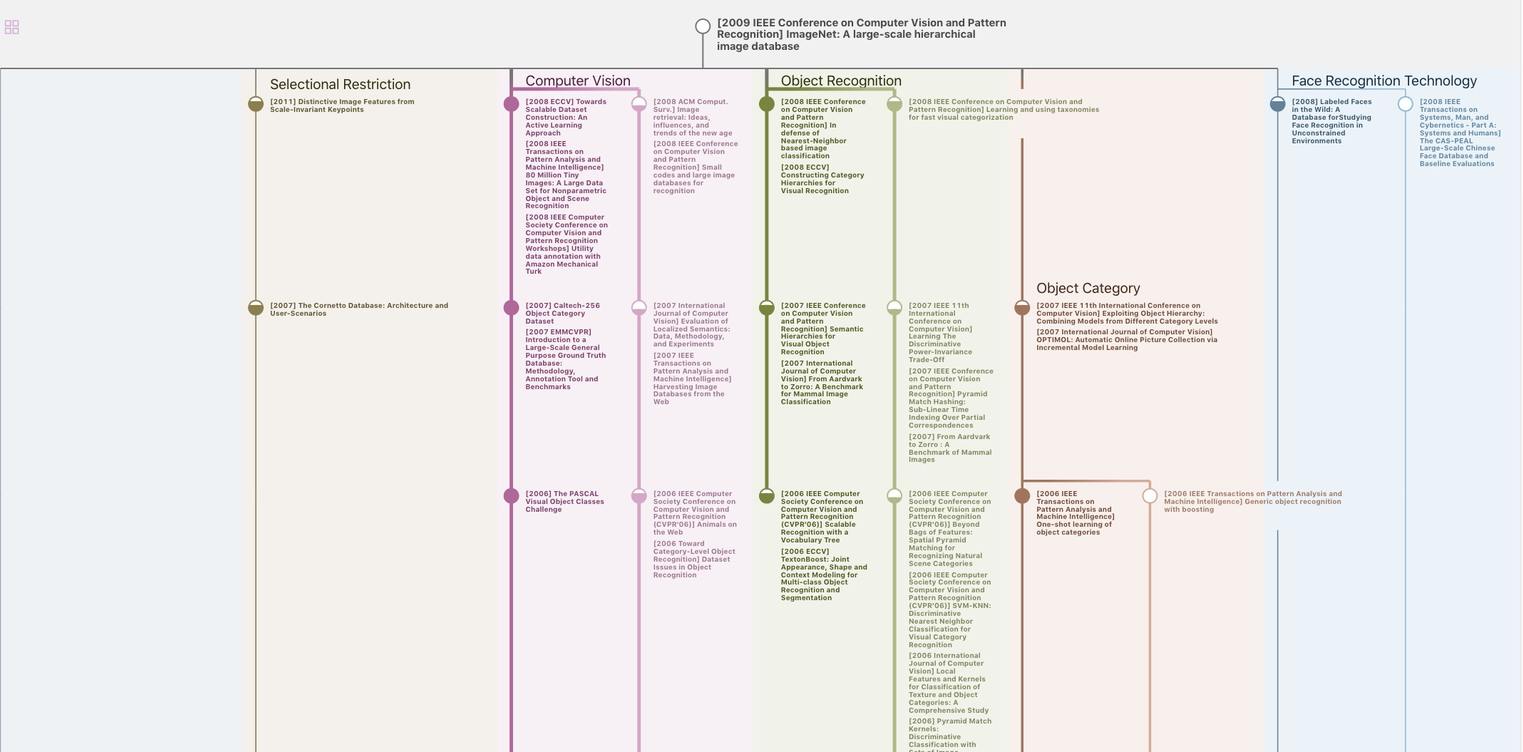
生成溯源树,研究论文发展脉络
Chat Paper
正在生成论文摘要