Predicting time‐at‐depth weighted biodiversity patterns for sharks of the North Pacific
Ecography(2024)
摘要
Depth is a fundamental and universal driver of ocean biogeography but it is unclear how the biodiversity patterns of larger, more mobile organisms change as a function of depth. Here, we developed a predictive biogeography model to explore how information of mobile species' depth preferences influence biodiversity patterns. We employed a literature review to collate shark biotelemetry studies and used open‐access tools to extract 283 total records from 119 studies of 1133 sharks from 35 species. We then matched field guide reported depth ranges and IUCN habitat associations for each shark species to use as covariates in a hurdle variant of ensemble random forests. We successfully fit this model (R2 = 0.63) to the noisy time‐at‐depth observations and used it to predict the time budgets of the northeast Pacific shark regional pool (n = 52). We then assessed how occurrence diversity patterns, informed by minimum and maximum depth of occurrence, compared to time‐at‐depth weighted diversity patterns. Time‐at‐depth weighted richness was highest between 0 and 25 m and at the upper part of the mesopelagic zone, 250–300 m; resulting in little similarity to common depth or elevational biodiversity patterns while the occurrence‐weighted richness pattern was similar to the ‘low‐plateau' pattern. In the phylogenetic and functional dimensions of biodiversity and over three different distance metrics, we found strong but haphazard differences between the occurrence‐ and time‐at‐depth weighted biodiversity patterns. The strong influence of time budgets on biodiversity led us to conclude that occurrence data alone are likely insufficient or even misleading in terms of the depth‐driven biogeographic patterns in the open ocean. Utilizing the increasing amount of time‐at‐depth information from biotelemetry studies in predictive biogeographic models may be critical for capturing the preferences of pelagic, mobile species occupying the largest biome on the planet.
更多查看译文
AI 理解论文
溯源树
样例
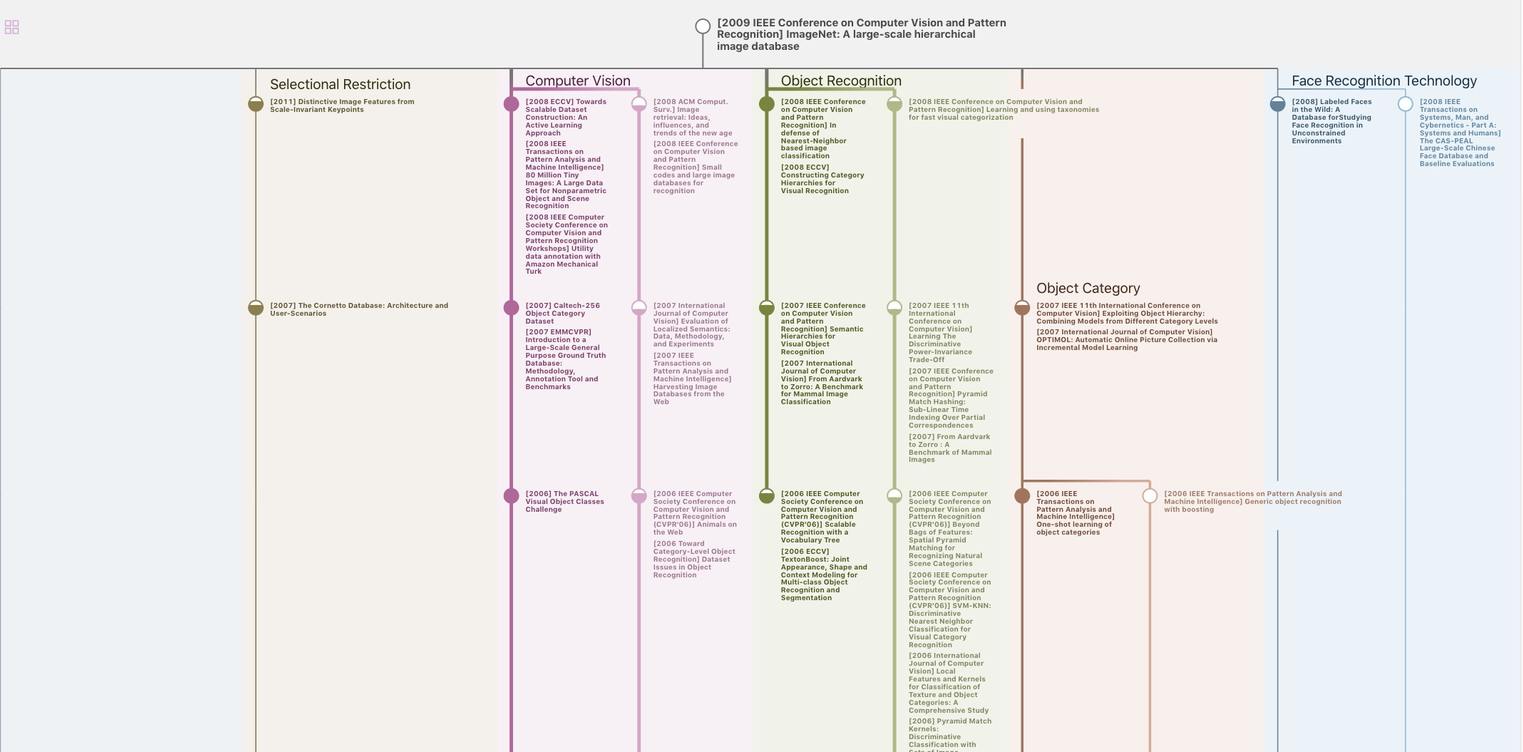
生成溯源树,研究论文发展脉络
Chat Paper
正在生成论文摘要