Robust maximum correlation entropy Kalman filtering algorithm based on S-functions under impulse noise
Signal, Image and Video Processing(2024)
Abstract
The impulse noise is heavy-tailed and distributed, which is common in many real-world scenarios of automatic control and target tracking. In order to improve the stability of Kalman filtering algorithms in the context of impulse noise, a number of Kalman filtering algorithms have been widely developed using the maximum correlation entropy instead of the minimum mean square error criterion. To better adapt to impulse noise environments. In this paper, we propose a new Kalman filtering algorithm called robust maximum correlation entropy Kalman filtering algorithm based on S-functions (HWSKF). The algorithm is based on a new cost function framework. On the one hand, this framework employs the properties of the Softplus function fused with the maximum correlation entropy to ensure that the algorithm is better adapted to the impulsive noise environment with faster convergence speed and stronger steady-state properties. On the other hand, the introduction of Huber regression weighting can effectively exclude the effect of outliers in the case of non-Gaussian noise. Under different impulse noise environments, we demonstrate through Monte Carlo simulation experiments that the algorithm proposed in this paper possesses high stationarity compared with the classical Kalman filtering algorithm (KF), the maximum correlation entropy Kalman filtering algorithm (MCKF), and the maximum correlation entropy Kalman filtering algorithm based on the student’s kernel (STTKF).
MoreTranslated text
Key words
Kalman filter algorithm,Huber regression,Impulse noise,Softplus function,Robust estimation
AI Read Science
Must-Reading Tree
Example
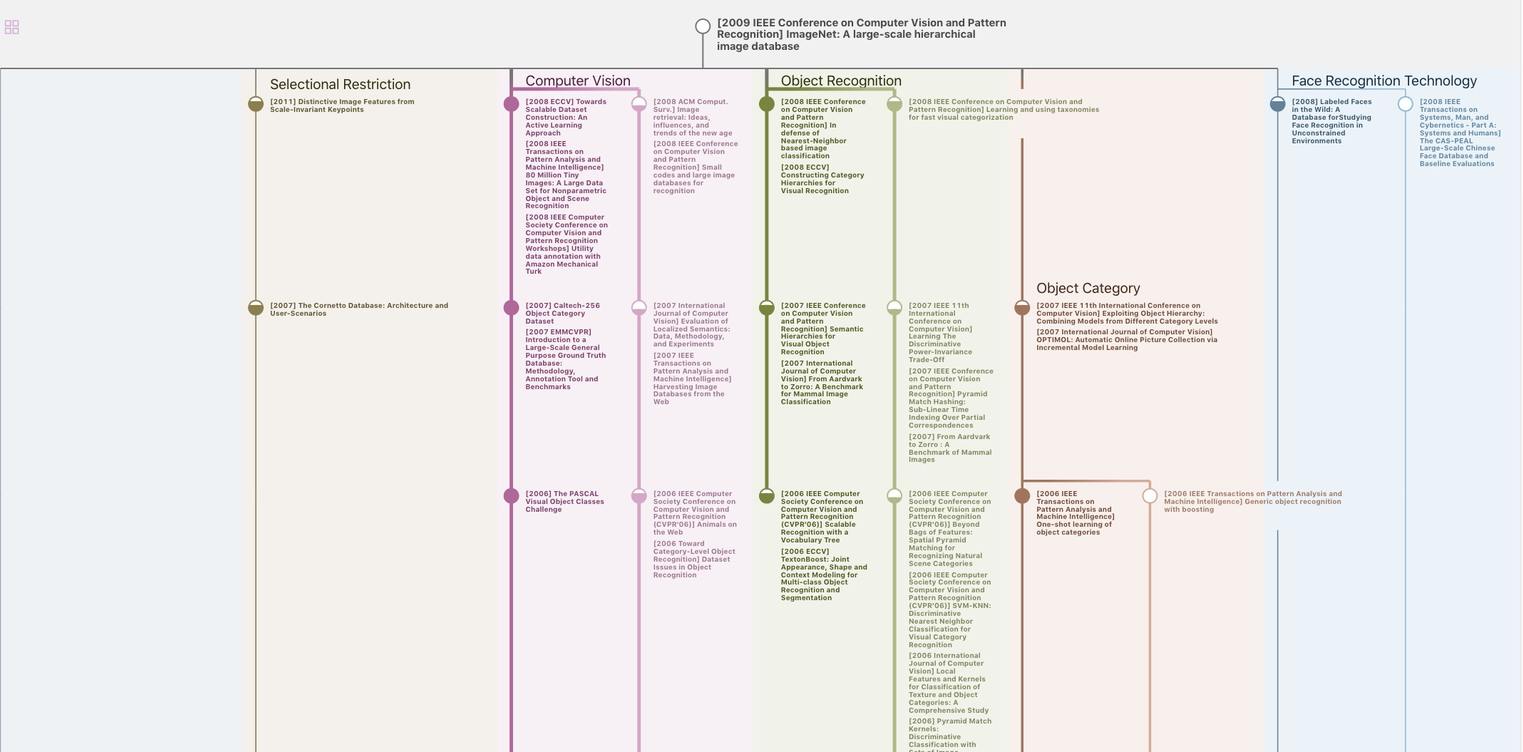
Generate MRT to find the research sequence of this paper
Chat Paper
Summary is being generated by the instructions you defined