Diverse Structure-Aware Relation Representation in Cross-Lingual Entity Alignment
ACM TRANSACTIONS ON KNOWLEDGE DISCOVERY FROM DATA(2024)
摘要
Cross-lingual entity alignment (CLEA) aims to find equivalent entity pairs between knowledge graphs (KGs) in different languages. It is an important way to connect heterogeneous KGs and facilitate knowledge completion. Existing methods have found that incorporating relations into entities can effectively improve KG representation and benefit entity alignment, and these methods learn relation representation depending on entities, which cannot capture the diverse structures of relations. However, multiple relations in KG form diverse structures, such as adjacency structure and ring structure. This diversity of relation structures makes the relation representation challenging. Therefore, we propose to construct theweighted line graphs to model the diverse structures of relations and learn relation representation independently from entities. Especially, owing to the diversity of adjacency structures and ring structures, we propose to construct adjacency line graph and ring line graph, respectively, to model the structures of relations and to further improve entity representation. In addition, to alleviate the hubness problem in alignment, we introduce the optimal transport into alignment and compute the distance matrix in a different way. From a global perspective, we calculate the optimal 1-to-1 alignment bi-directionally to improve the alignment accuracy. Experimental results on two benchmark datasets show that our proposed method significantly outperforms state-of-the-art CLEA methods in both supervised and unsupervised manners.
更多查看译文
关键词
Cross-lingual entity alignment,knowledge graph,line graph,optimal transport
AI 理解论文
溯源树
样例
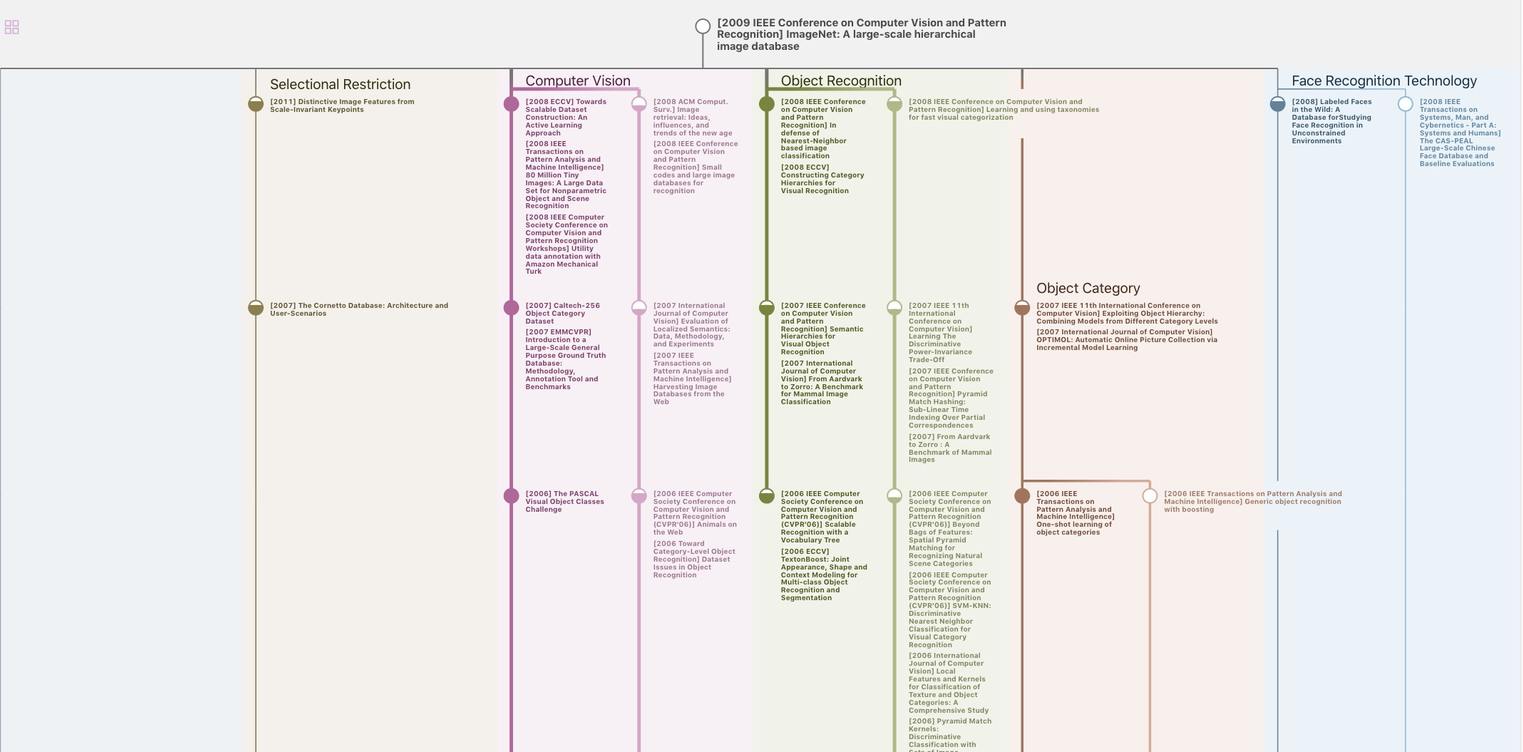
生成溯源树,研究论文发展脉络
Chat Paper
正在生成论文摘要