Multiple-instance Learning from Triplet Comparison Bags
ACM TRANSACTIONS ON KNOWLEDGE DISCOVERY FROM DATA(2024)
摘要
Multiple-instance learning (MIL) solves the problemwhere training instances are grouped in bags, and a binary (positive or negative) label is provided for each bag. Most of the existing MIL studies need fully labeled bags for training an effective classifier, while it could be quite hard to collect such data in many real-world scenarios, due to the high cost of data labeling process. Fortunately, unlike fully labeled data, triplet comparison data can be collected in a more accurate and human-friendly way. Therefore, in this article, we for the first time investigate MIL from only triplet comparison bags, where a triplet (Xa, Xb, Xc) contains the weak supervision information that bag Xa is more similar to Xb than to Xc. To solve this problem, we propose to train a bag-level classifier by the empirical risk minimization framework and theoretically provide a generalization error bound. We also show that a convex formulation can be obtained only when specific convex binary losses such as the square loss and the double hinge loss are used. Extensive experiments validate that our proposed method significantly outperforms other baselines.
更多查看译文
关键词
Multi-Instance Learning,Triplet Comparison,Empirical Risk Minimization
AI 理解论文
溯源树
样例
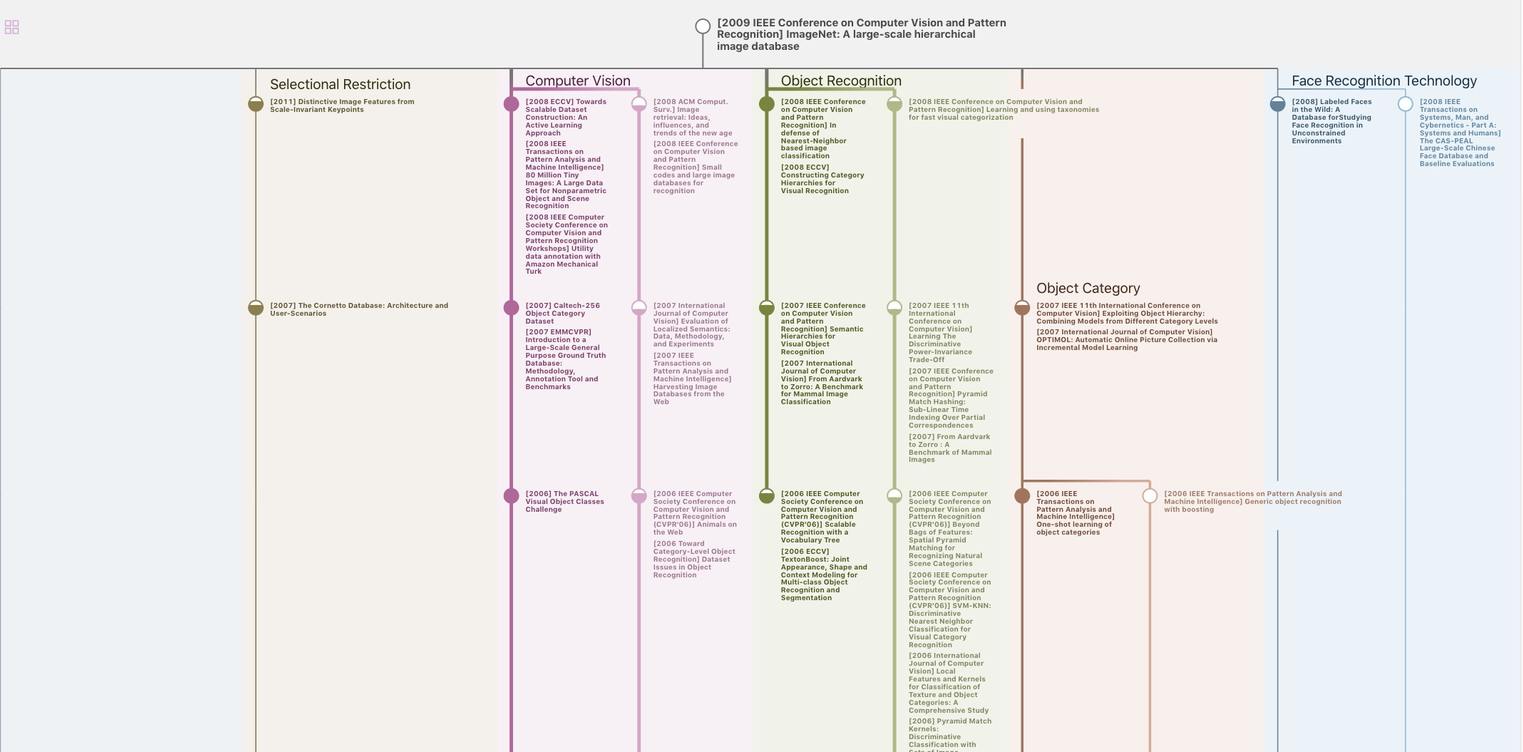
生成溯源树,研究论文发展脉络
Chat Paper
正在生成论文摘要