Research on micro parallax adversarial sample generation method based on texture sensitive region
JOURNAL OF INTELLIGENT & FUZZY SYSTEMS(2024)
摘要
Deep neural networks have been extensively applied in fields such as image classification, object detection, and face recognition. However, research has shown that adversarial samples with subtle perturbations can effectively deceive these networks. Existing methods for generating such adversarial images often lack stealth and robustness. In this study, we present an enhanced attack strategy based on traditional Generative Adversarial Networks (GANs). We integrate image texture into the unsupervised training scheme, guiding the model to focus perturbations in high-texture areas. We also introduce a dynamic equilibrium training strategy that employs Differential Evolution algorithms to adaptively adjust both network weight parameters and the training ratio between the generator and discriminator, achieving a self-balancing training process. Further, we propose an image local optimization algorithm to eliminate perturbations in non-sensitive areas through weighted filtering. The model is validated using benchmark datasets such as MNIST, ImageNet and SVHN. Through extensive experimental evaluations, our approach shows a 4.93% improvement in attack success rate against conventional models and a 10.23% increase against defense models compared to state-of-the-art attack methods.
更多查看译文
关键词
Adversarial samples,texture sensitive region,GAN networks,micro parallax,optimization algorithm
AI 理解论文
溯源树
样例
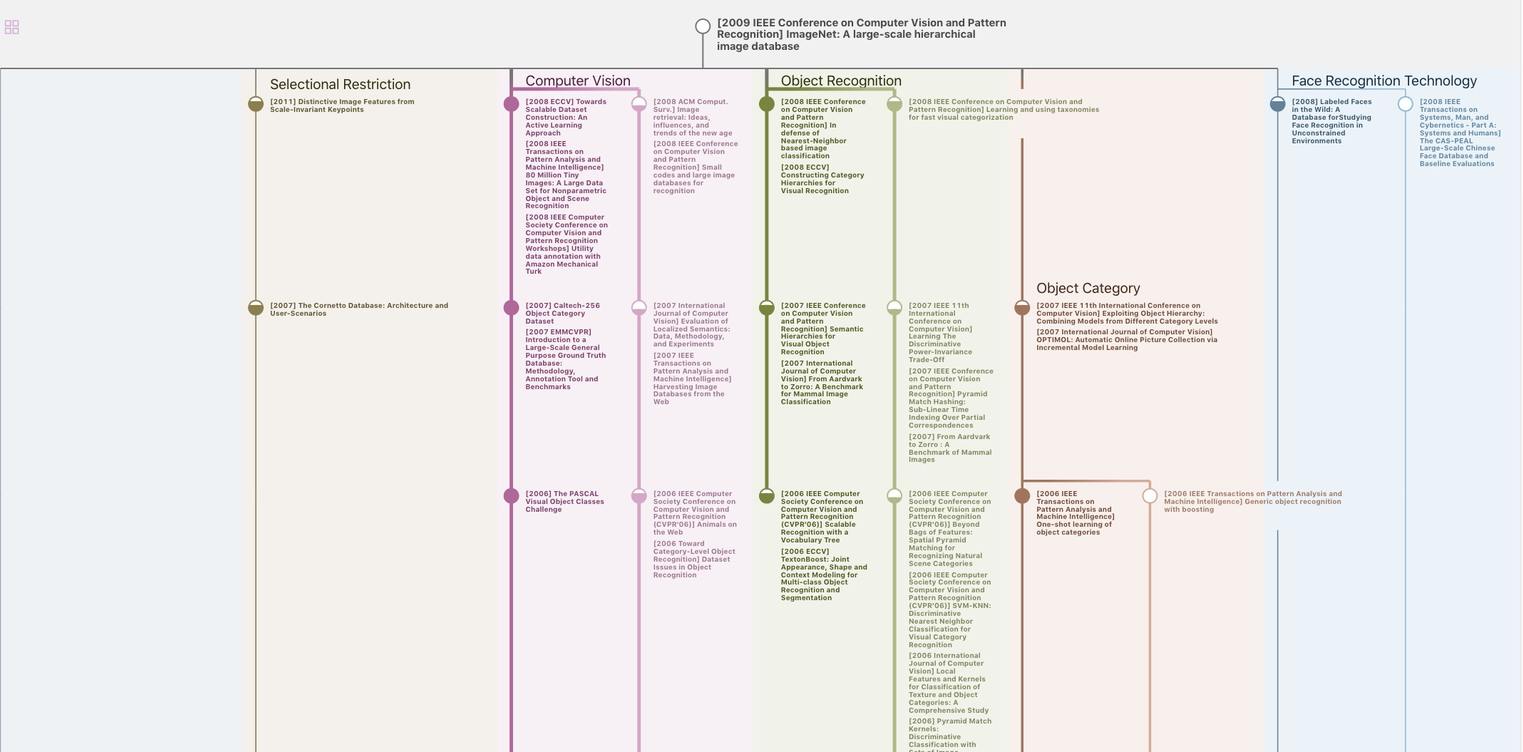
生成溯源树,研究论文发展脉络
Chat Paper
正在生成论文摘要