GA-RCNN:Graph self-attention feature extraction for 3D object detection
JOURNAL OF INTELLIGENT & FUZZY SYSTEMS(2024)
摘要
In recent years, 3D object detection based on LiDAR point clouds is a key component of autonomous driving. In pursuit of enhancing the accuracy of 3D point cloud feature extraction and point cloud detection, this paper introduces a novel 3D object detection model, termed as Graph Self-Attention-RCNN (GA-RCNN). This model is designed to integrate voxel information and point location information, enhancing the quality of 3D object proposals while maintaining contextual accuracy. The first stage rectifies the previous approach that relied on local features for preselected boxes, overlooking crucial global contextual information. An improved method is suggested in this work, utilizing BEV to capture long-range dependencies via a cross-attention mechanism. The second stage addresses the overreliance on local neighborhood point feature extraction. The Graph Self-Attention Pooling method is proposed, characterized by its dynamic computation of contribution weights for inputs. This enhances the model's flexibility and generalization performance. Extensive evaluations on KITTI andWaymo datasets demonstrate GA-RCNN's superior accuracy compared to other methods, affirming its efficacy in 3D object detection.
更多查看译文
关键词
3D object detection,Point clouds,deep learning
AI 理解论文
溯源树
样例
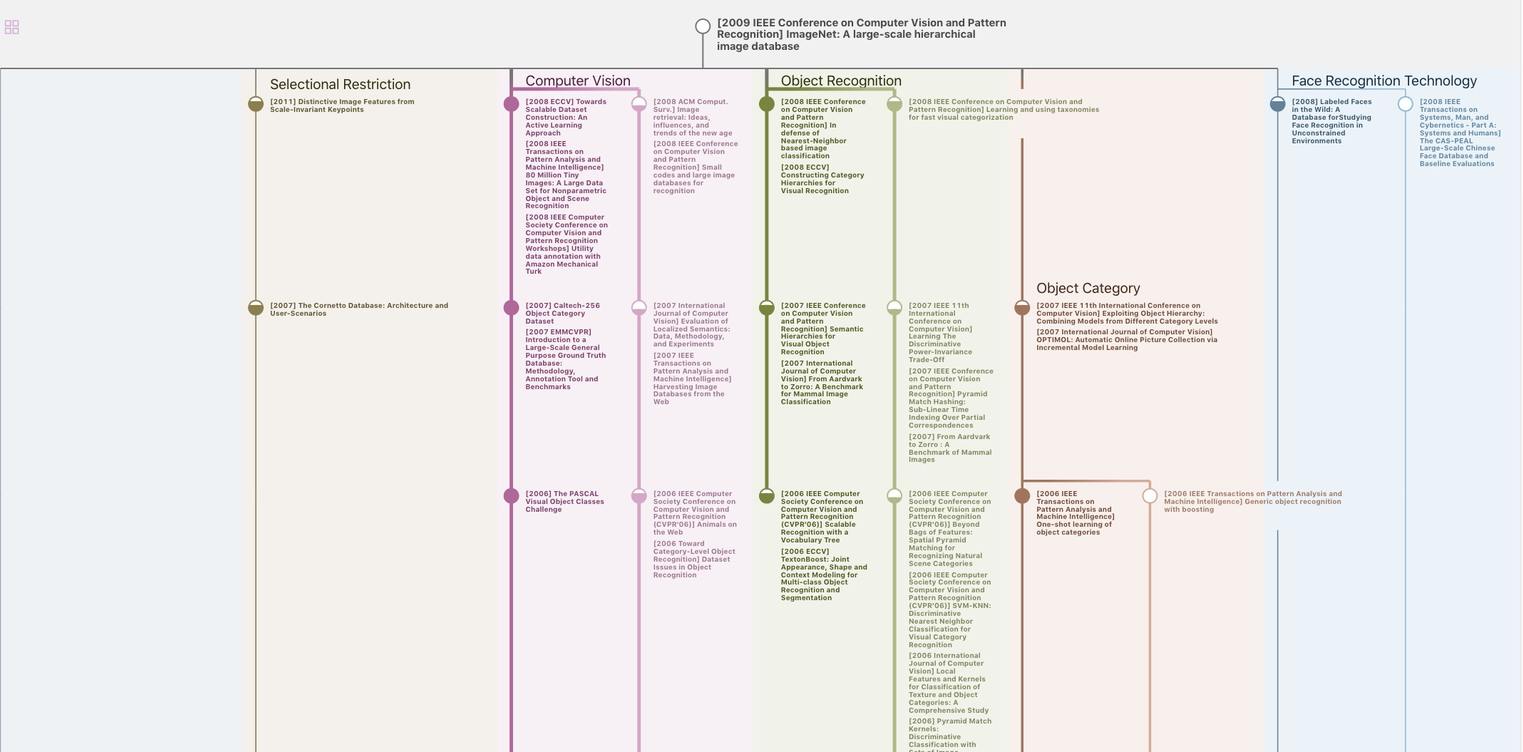
生成溯源树,研究论文发展脉络
Chat Paper
正在生成论文摘要