A stacked architecture-based fuzzy classifier with data position transformation using fuzzy cognitive maps
JOURNAL OF INTELLIGENT & FUZZY SYSTEMS(2024)
摘要
The amalgamation of fuzzy model and deep learning has become one hot topic in today's fuzzy community. However, with the model goes deeper, a pivotal aspect for performance enhancement, the interpretability of the model will deteriorate. To enhance the classification accuracy of classifiers while ensuring interpretability, we propose a stacked architecture-based fuzzy classifier named PT-SAFC. Borrowing the hierarchically stacked thought originated from deep learning, the PT-SAFC is composed by stacking two distinct fuzzy systems, implemented by fuzzy neuro-networks. Here, we propose an improved Takagi-Sugeno-Kang (TSK) model (PTFS) for data transfer by incorporating fuzzy cognitive maps (FCM). It imparts the TSK model with the data processing capability akin to deep learning models, thereby mitigating the interpretability loss arising from an increase in model depth. Furthermore, the multi-prototypes fuzzy system for decision making (MPDFS) is constructed to map data onto classes. An enhanced gradient descent method with restriction mechanism of prototype position is designed for parameter optimization. The experiments underscore PT-SAFC's achievement of a harmonious equilibrium between interpretability and classification accuracy. And, PT-SAFC maintains an advantage in classification performance even compared to deep learning methods. Furthermore, experiments validate PT-SAFC's capability to manipulate data distribution to augment classification efforts.
更多查看译文
关键词
fuzzy classifier,fuzzy cognitive map,data position transformation,gradient descent method
AI 理解论文
溯源树
样例
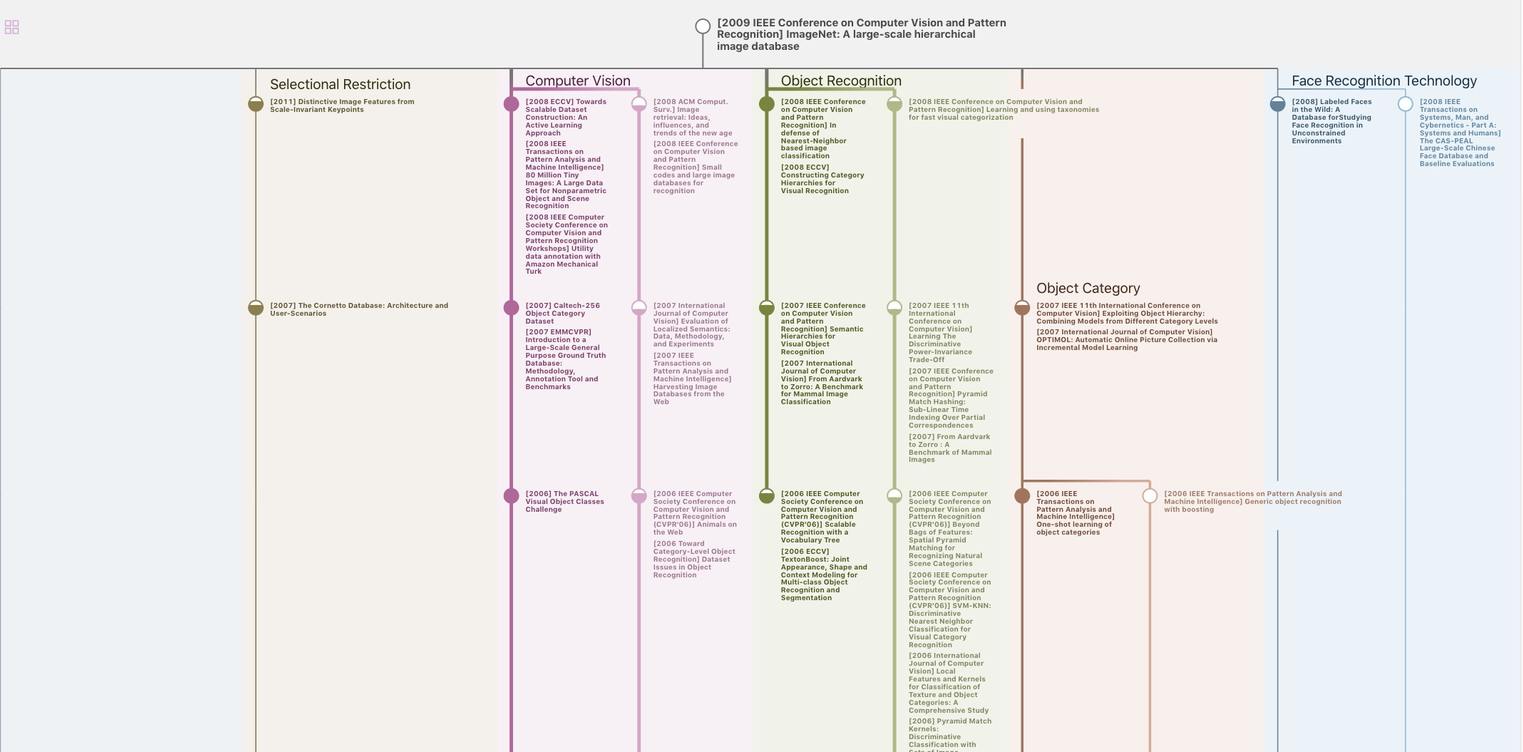
生成溯源树,研究论文发展脉络
Chat Paper
正在生成论文摘要