Bearing Fault Diagnosis Using Multichannel Broad Learning System Based on Positive-Negative Weighted Voting Mechanism
IEEE TRANSACTIONS ON INSTRUMENTATION AND MEASUREMENT(2024)
摘要
Bearing fault diagnosis is essential for improving the efficiency of industrial operations. The inherently multimodal nature of bearing vibration signals presents significant challenges in bearing fault diagnosis. To address this issue, we propose a novel multichannel broad learning system (MCBLS). MCBLS comprises multiple broad learning systems (BLSs) and incorporates a positive-negative weighted voting mechanism (PNWV). MCBLS first employs empirical mode decomposition to break down the vibration signal into multiple intrinsic mode functions (IMFs) and subsequently transforms these IMFs into concise spectrogram distributions (CSDs). Following this, MCBLS employs multiple BLSs, with each one being trained based on a CSD associated with an IMF. The final diagnosis result is obtained by aggregating all BLSs' outcomes using the PNWV. To validate MCBLS, we employ three bearing fault datasets collected from Guangdong University of Petrochemical Technology (GDUPT), Maoming, China, Case Western Reserve University (CWRU), Cleveland, OH, USA, and Southeast University (SEU), Nanjing, China. The accuracies of MCBLS in three datasets can reach up to 98.11%, 100%, and 94.33%, respectively, demonstrating its superior classification performance and applicable generalization for bearing fault diagnosis.
更多查看译文
关键词
Fault diagnosis,Learning systems,Mathematical models,Vibrations,Time-frequency analysis,Spectrogram,Feature extraction,Bearing fault diagnosis,concise spectrogram distribution (CSD),multichannel broad learning system (MCBLS),neural network,positive-negative weighted voting mechanism (PNWV)
AI 理解论文
溯源树
样例
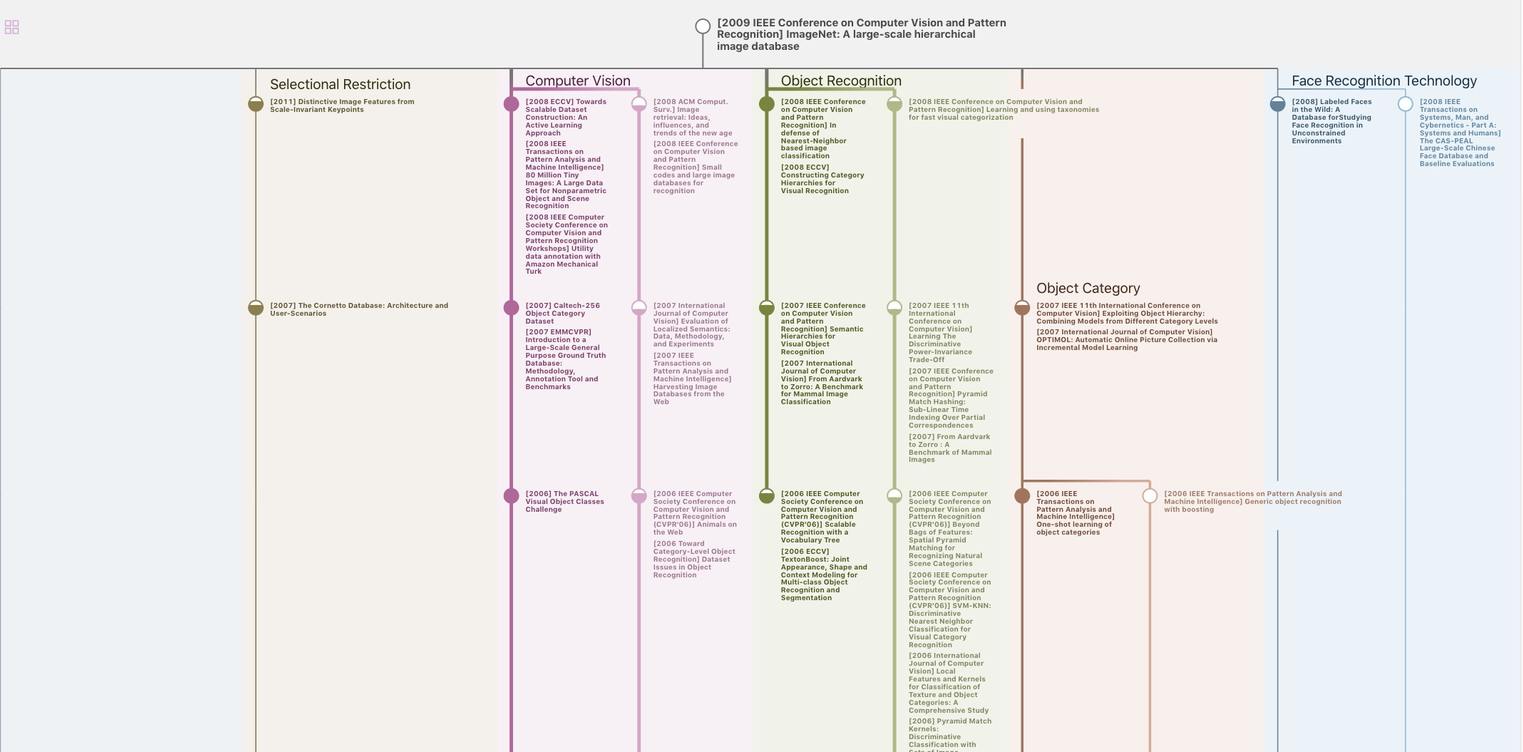
生成溯源树,研究论文发展脉络
Chat Paper
正在生成论文摘要