A Feature-Level Mask Self-Supervised Assisted Learning Approach Based on Transformer for Remaining Useful Life Prediction.
Intelligent data analysis(2024)
Abstract
Nowadays, the massive industrial data has effectively improved the performance of the data-driven deep learning Remaining Useful Life (RUL) prediction method. However, there are still problems of assigning fixed weights to features and only coarse-grained consideration at the sequence level. This paper proposes a Transformer-based end-to-end feature-level mask self-supervised learning method for RUL prediction. First, by proposing a fine-grained feature-level mask self-supervised learning method, the data at different time points under all features in a time window is sent to two parallel learning streams with and without random masks. The model can learn more fine-grained degradation information by comparing the information extracted by the two parallel streams. Instead of assigning fixed weights to different features, the abstract information extracted through the above process is invariable correlations between features, which has a good generalization to various situations under different working conditions. Then, the extracted information is encoded and decoded again using an asymmetric structure, and a fully connected network is used to build a mapping between the extracted information and the RUL. We conduct experiments on the public C-MAPSS datasets and show that the proposed method outperforms the other methods, and its advantages are more obvious in complex multi-working conditions.
MoreTranslated text
Key words
Remaining useful life prediction,multi-working conditions,self-supervised learning,transformer,feature-level mask
AI Read Science
Must-Reading Tree
Example
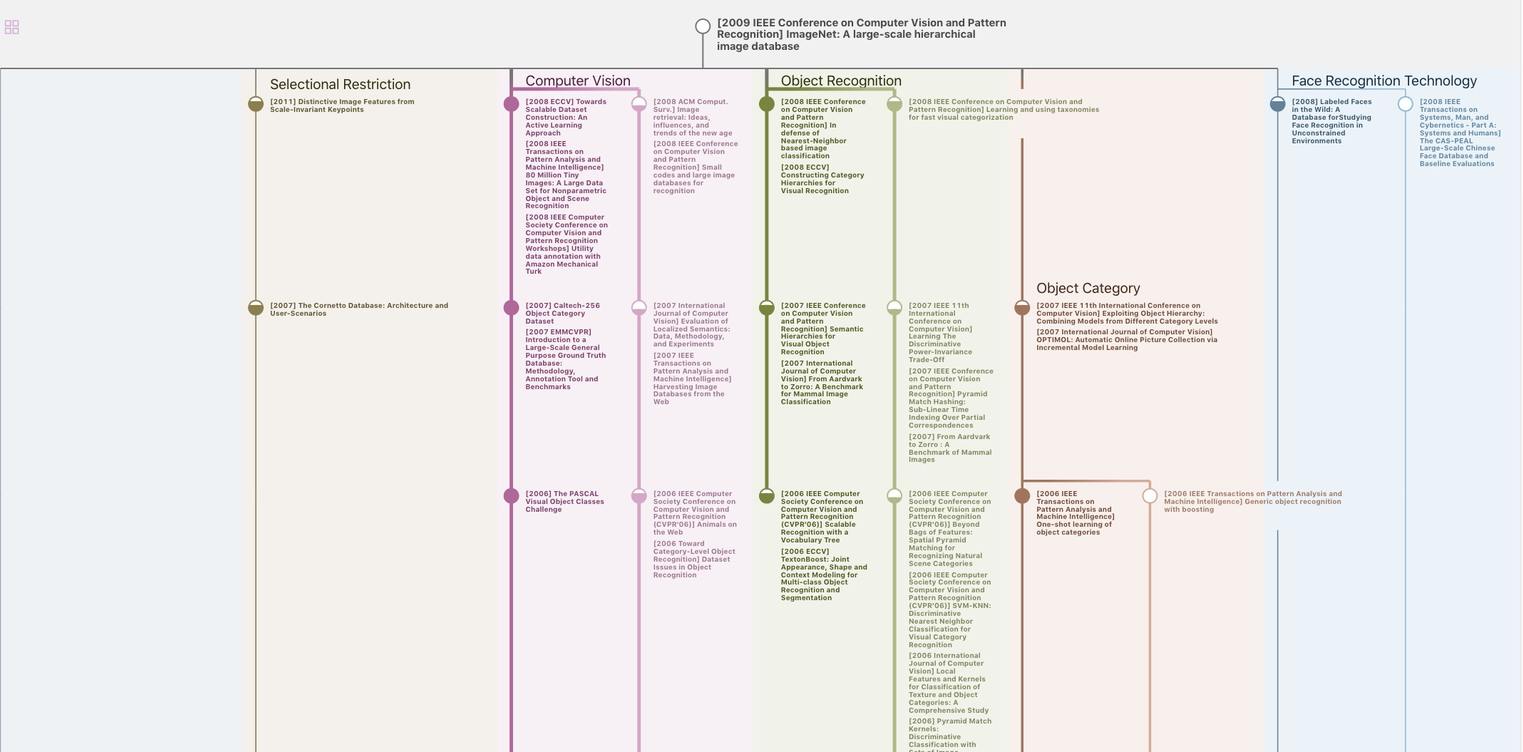
Generate MRT to find the research sequence of this paper
Chat Paper
Summary is being generated by the instructions you defined