MeFiNet: Modeling multi-semantic convolution-based feature interactions for CTR prediction
INTELLIGENT DATA ANALYSIS(2024)
摘要
Extracting more information from feature interactions is essential to improve click-through rate (CTR) prediction accuracy. Although deep learning technology can help capture high-order feature interactions, the combination of features lacks interpretability. In this paper, we propose a multi-semantic feature interaction learning network (MeFiNet), which utilizes convolution operations to map feature interactions to multi-semantic spaces to improve their expressive ability and uses an improved Squeeze & Excitation method based on SENet to learn the importance of these interactions in different semantic spaces. The Squeeze operation helps to obtain the global importance distribution of semantic spaces, and the Excitation operation helps to dynamically re-assign the weights of semantic features so that both semantic diversity and feature diversity are considered in the model. The generated multi-semantic feature interactions are concatenated with the original feature embeddings and input into a deep learning network. Experiments on three public datasets demonstrate the effectiveness of the proposed model. Compared with state-of-the-art methods, the model achieves excellent performance (+0.18% in AUC and -0.34% in LogLoss VS DeepFM; +0.19% in AUC and -0.33% in LogLoss VS FiBiNet).
更多查看译文
关键词
Click-through rate prediction,multi-semantic,feature interaction,convolution,squeeze-excitation network
AI 理解论文
溯源树
样例
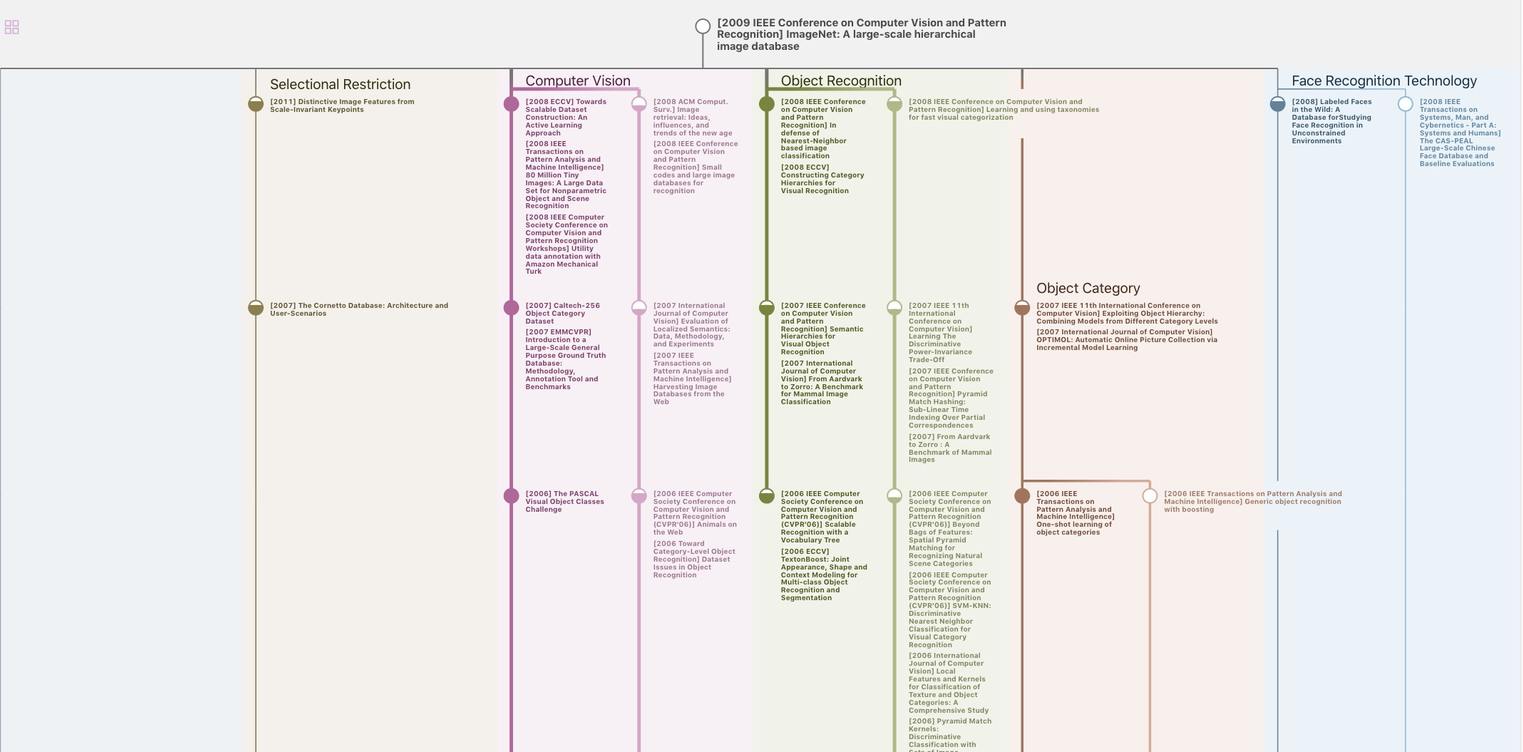
生成溯源树,研究论文发展脉络
Chat Paper
正在生成论文摘要