What Fairness Metrics Can Really Tell You: A Case Study in the Educational Domain
FOURTEENTH INTERNATIONAL CONFERENCE ON LEARNING ANALYTICS & KNOWLEDGE, LAK 2024(2024)
摘要
Recently, discussions on fairness and algorithmic bias have gained prominence in the learning analytics and educational data mining communities. To quantify algorithmic bias, researchers and practitioners often use popular fairness metrics, e.g., demographic parity, without discussing their choices. This can be considered problematic, as the choices should strongly depend on the underlying data generation mechanism, the potential application, and normative beliefs. Likewise, whether and how one should deal with the indicated bias depends on these aspects. This paper presents and discusses several theoretical cases to highlight precisely this. By providing a set of examples, we hope to facilitate a practice where researchers discuss potential fairness concerns by default.
更多查看译文
关键词
fairness,education,causal models
AI 理解论文
溯源树
样例
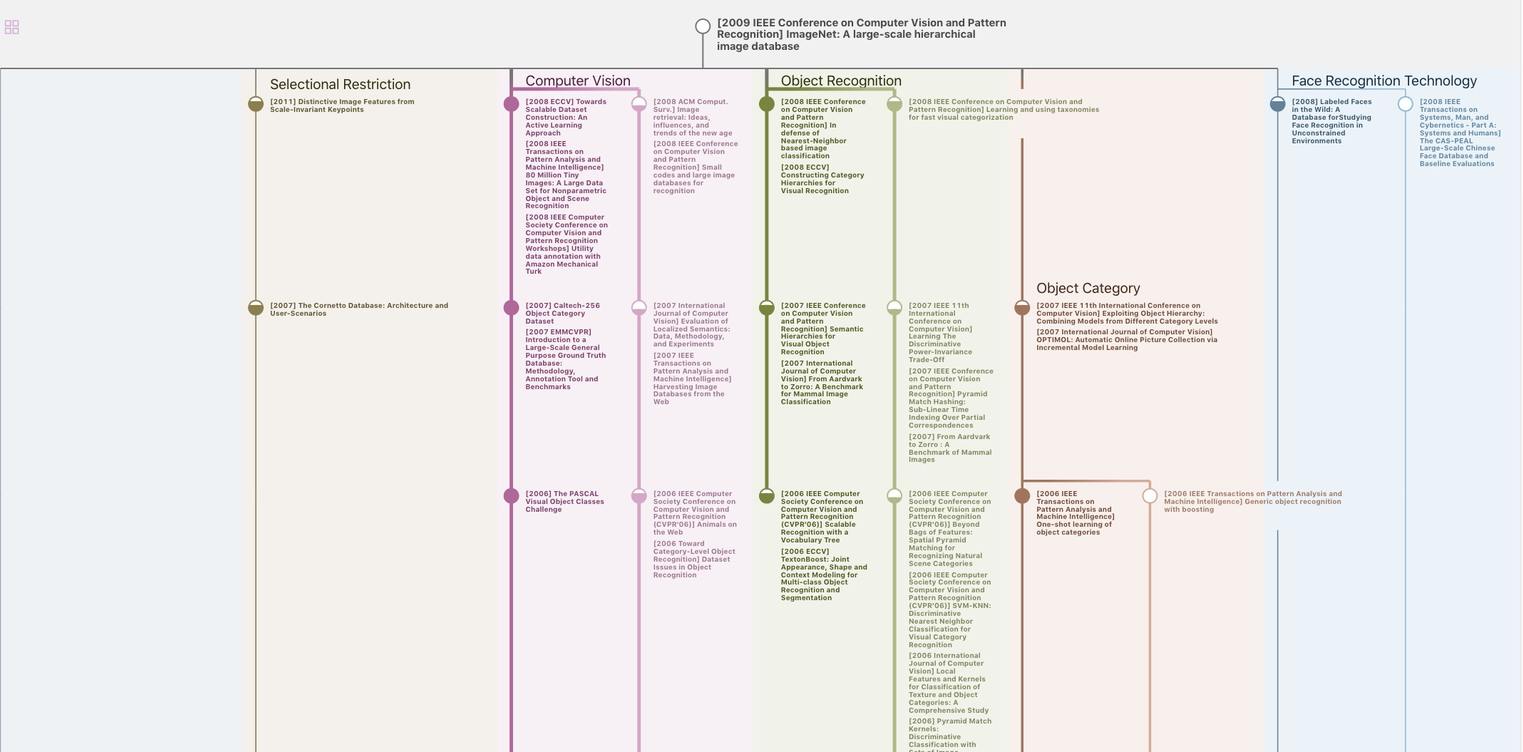
生成溯源树,研究论文发展脉络
Chat Paper
正在生成论文摘要