Exploring Probabilistic Models for Semi-supervised Learning
arxiv(2024)
摘要
This thesis studies advanced probabilistic models, including both their
theoretical foundations and practical applications, for different
semi-supervised learning (SSL) tasks. The proposed probabilistic methods are
able to improve the safety of AI systems in real applications by providing
reliable uncertainty estimates quickly, and at the same time, achieve
competitive performance compared to their deterministic counterparts. The
experimental results indicate that the methods proposed in the thesis have
great value in safety-critical areas, such as the autonomous driving or medical
imaging analysis domain, and pave the way for the future discovery of highly
effective and efficient probabilistic approaches in the SSL sector.
更多查看译文
AI 理解论文
溯源树
样例
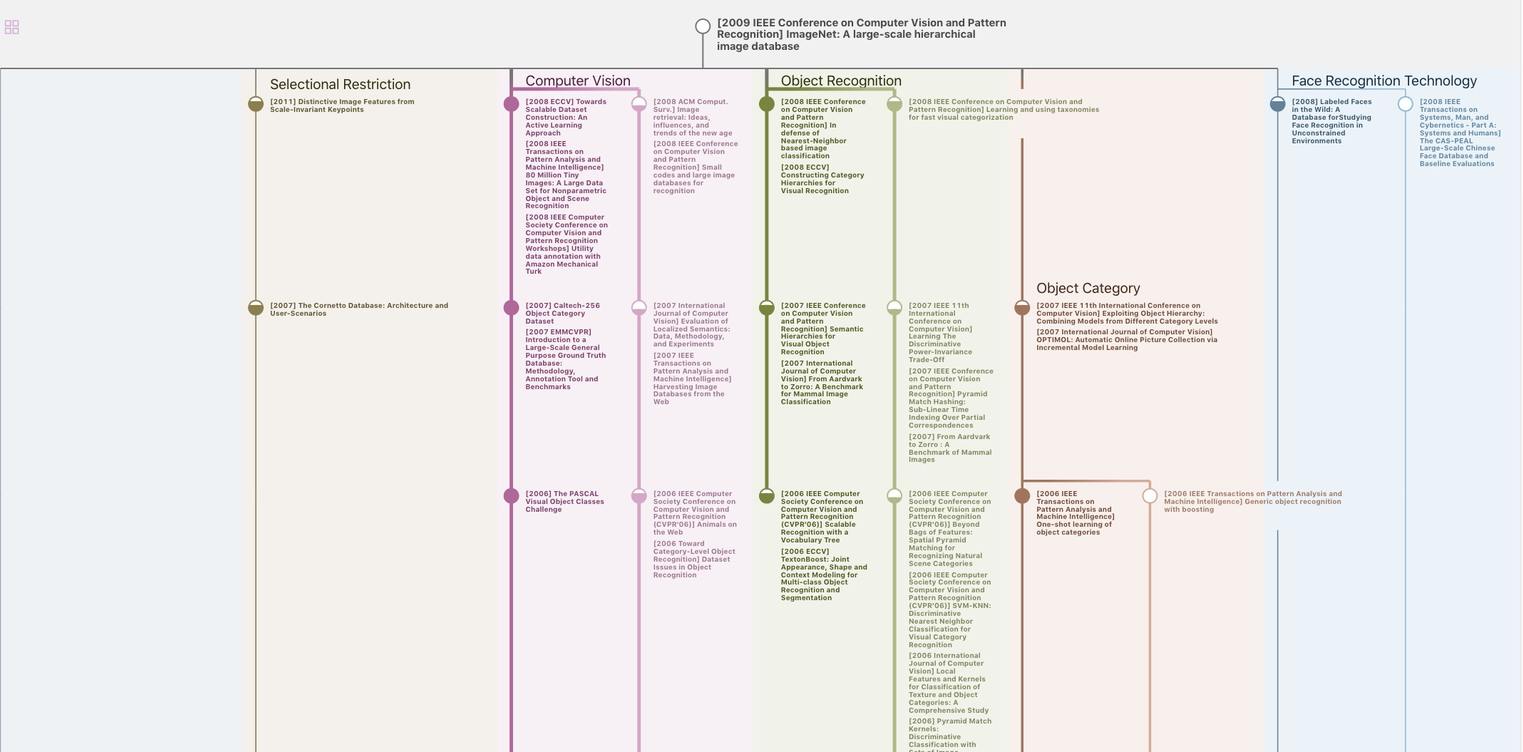
生成溯源树,研究论文发展脉络
Chat Paper
正在生成论文摘要