Using dynamic data augmentation and contrastive learning for iris verification
Journal of Measurement Science and Instrumentation(2024)
摘要
Iris verification has gained extensive attention because of its uniqueness, stability, and non-invasiveness. Deep learning techniques have made significant progress in the field of iris verification. By using convolutional neural networks (CNNs), features of iris images can be automatically extracted and learned, enabling high-precision identity verification. However, challenges such as intra-class variability and limited dataset size can compromise verification accuracy. To address these issues, we proposed an iris verification method based on dynamic data augmentation and contrastive learning. Four data augmentation strategies were carefully designed for online iris enhancement and dataset expansion, and the accuracy of iris verification was further improved by using data augmentation probability scheduler(DAPS). The MobileNetV3 was employed as the backbone network, which was optimized with contrastive learning for 3-channel iris pairs. The proposed method was evaluated on two benchmark iris databases, CASIA-V4-Interval and CASIA-V4-Thousand, achieving high accuracies of 99.85% and 98.82%, respectively. Experimental results demonstrate that the proposed method can achieve competitive performance with a small number of training samples.
更多查看译文
AI 理解论文
溯源树
样例
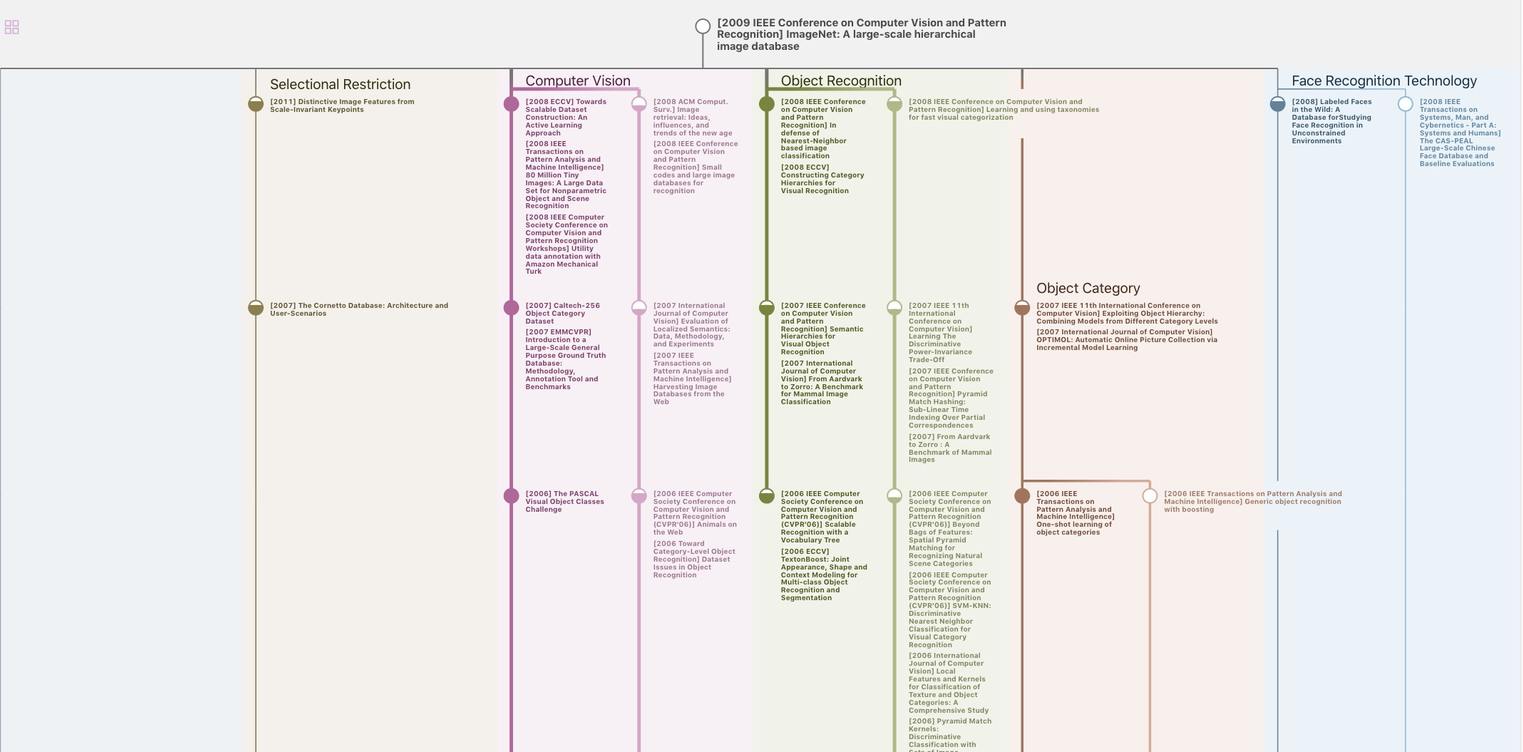
生成溯源树,研究论文发展脉络
Chat Paper
正在生成论文摘要