Estimation of soil erodability parameters based on different machine algorithms integrated with remote sensing techniques
International Journal of Environmental Science and Technology(2024)
Abstract
Erosion causes significant damage to life and nature every year; therefore, controlling erosion is of great importance. Therefore, maintaining the balance between soil, plants, and water plays a vital role in controlling erosion. Aim of this study was to estimate some erodability parameters (structural stability index—SSI, aggregate stability—AS, and erosion ratio—ER) with indices and reflectance obtained via TripleSat satellite imagery using machine learning algorithms (support vector regression—SVR, artificial neural network—ANN, and K-nearest neighbors—KNN) in Samsun Province, Vezirköprü, Türkiye. Various interpolation methods (inverse distance weighting—IDW, radial basis function—RBF, and kriging) were also used to create spatial distribution maps of the study area for observed and predicted values. Estimates were made using NDVI, SAVI, and ASVI indices obtained from satellite images and NIR reflectance. Accordingly, the ANN algorithm yielded the lowest MAE (2.86
MoreTranslated text
Key words
Soil properties,Erodibility factors,Machine-learning algorithm,Remote sensing,Kriging
AI Read Science
Must-Reading Tree
Example
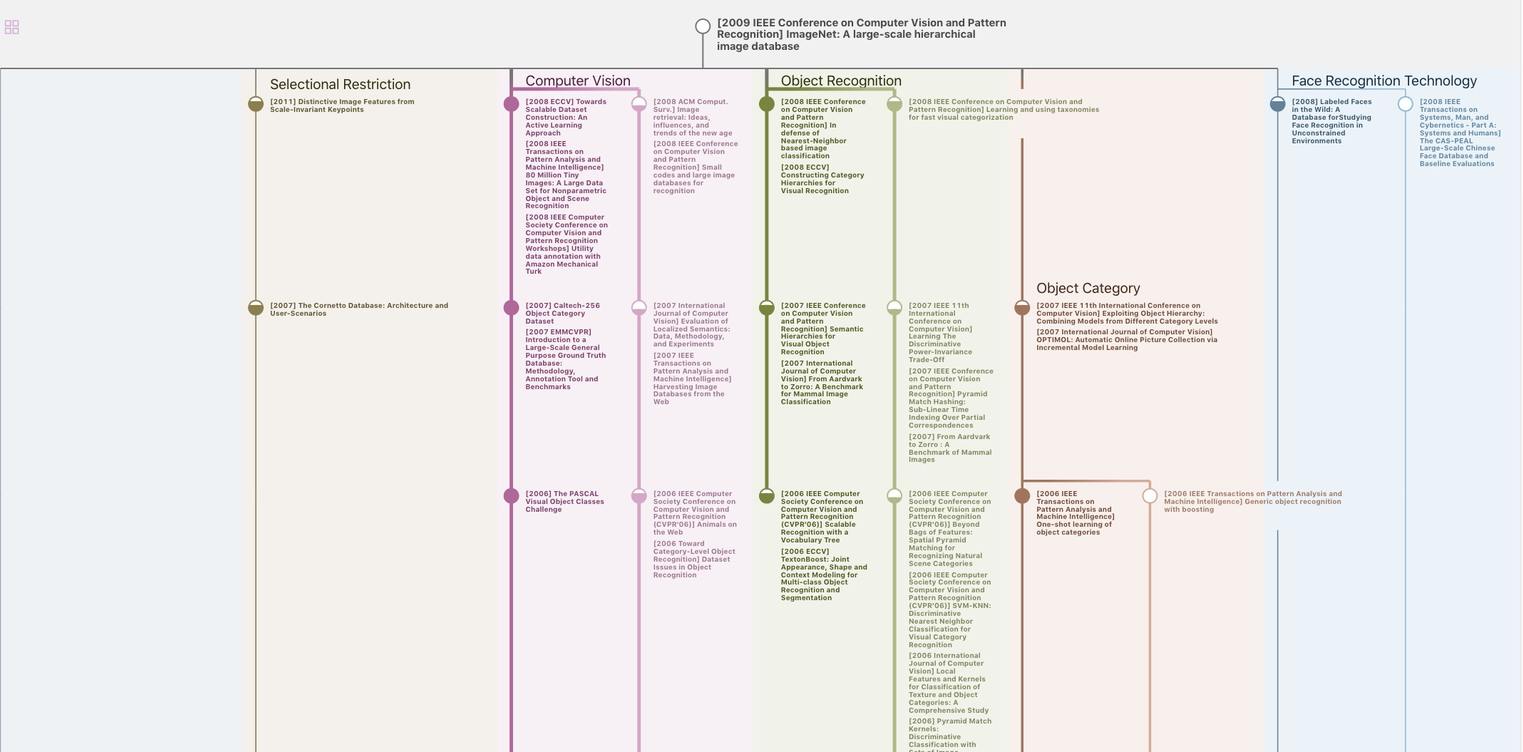
Generate MRT to find the research sequence of this paper
Chat Paper
Summary is being generated by the instructions you defined