A deep reinforcement learning-driven multi-objective optimization and its applications on aero-engine maintenance strategy
Journal of Manufacturing Systems(2024)
摘要
Efficiently optimizing maintenance strategies is vital for the cost reduction of complex equipment. Most of the current research in this domain primarily focuses on single-objective optimization, often centered on cost-based approaches. However, this approach may not be sufficient for complex equipment like aero-engines, which operate in complex conditions and exhibit nonlinear degradation, posing significant reliability challenges. To tackle this issue, a multi-objective optimization problem (MOP) has been formulated and solved in this study, encompassing models for both maintenance cost and reliability. The optimization of multiple objectives involves employing a decomposition strategy that amalgamates these objectives into a unified single objective. Additionally, the training process is expedited through the utilization of a neighborhood-based parameter-transfer strategy. The approach represents the development of a maintenance framework that seamlessly integrates the decomposition strategy, the neighborhood-based parameter-transfer strategy, and deep reinforcement learning (DRL). Notably, this framework eliminates the need for predetermined maintenance thresholds and predefined weights for various objectives, effectively overcoming the overconfidence typically observed in DRL during single-objective optimization. Applying this framework to the optimization of aero-engine maintenance strategies that take economic dependence into account, cost reductions of up to 16.7% were achieved compared to traditional baseline maintenance strategies. It is proved that the proposed method can reduce maintenance costs while ensuring reliability, and can adapt to dynamic changes in the environment.
更多查看译文
关键词
Aero-engine,Maintenance strategy,Multi-objective optimization,Reliability,Deep reinforcement learning
AI 理解论文
溯源树
样例
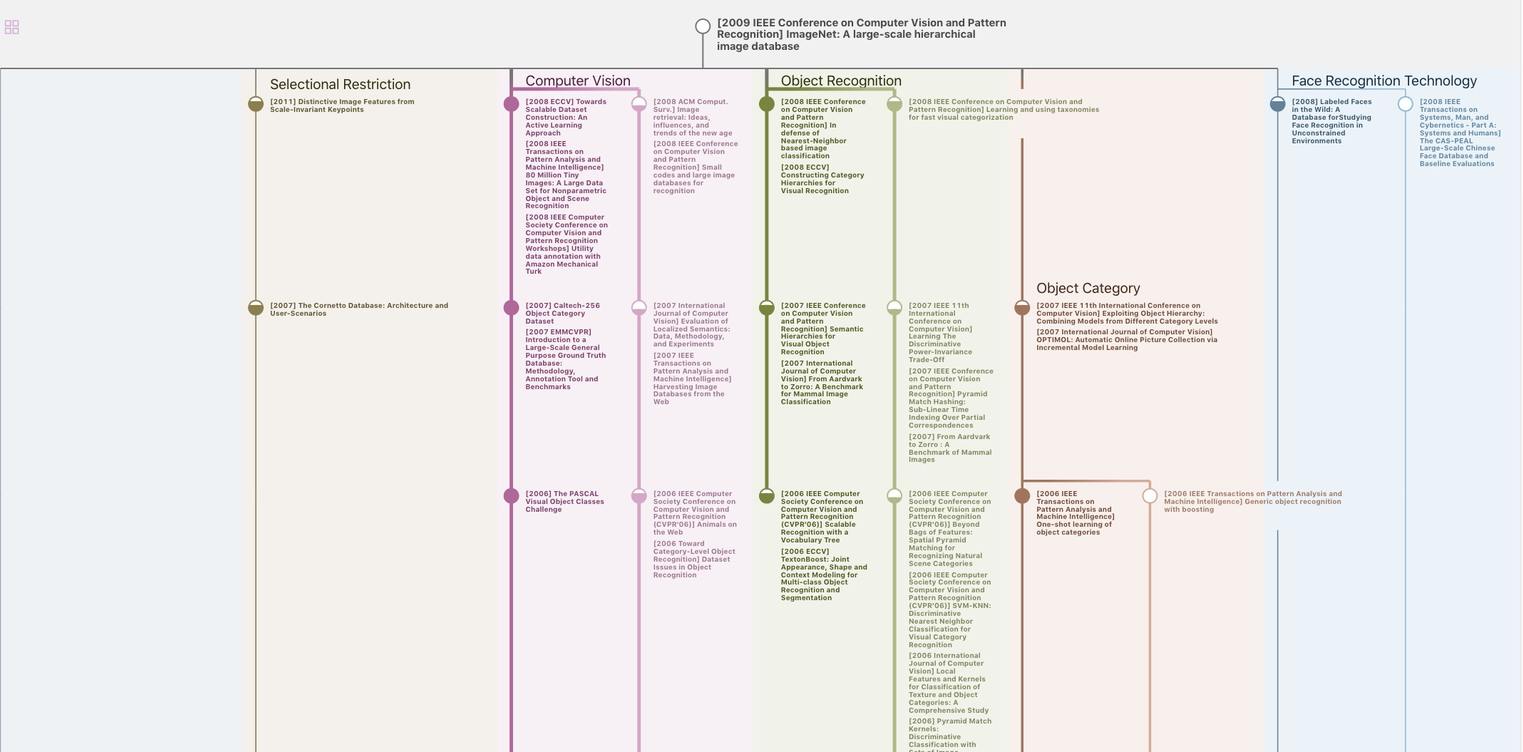
生成溯源树,研究论文发展脉络
Chat Paper
正在生成论文摘要