Adversarially robust and real-time DDoS detection and classification framework using AutoML
INFORMATION SECURITY JOURNAL(2024)
摘要
Denial of Service (DoS) attacks target the availability part of the CIA triad (Confidentiality, Availability, and Integrity). A special category of these attacks is the Distributed DoS (DDoS) attack, where the attacker uses a network of compromised systems called a botnet to flood a target server with requests and refuses to serve legitimate users. DDoS attacks can cost an organization millions of dollars in terms of lost revenue, remediation costs, and damage to brand reputation. Hence, all organizations need speedy real-time detection of DDoS attacks. This work presents a DDoS detection and classification framework using the flow-based approach for feature engineering and the AutoML technique. Our detection system is trained on the latest DDoS datasets - CIC-DDoS 2019 and CIC-IDS 2017, which contain various categories of DDoS attacks. We use various tools to perform adversarial attacks on our trained model. We retrain our models using adversarially crafted network packet captures and then test our models for robustness against practical adversarial attacks that an attacker might use to evade detection. Finally, we deploy our model in real-time using a GUI-based tool. Our model achieves a validation accuracy of 99.9% and a low false positive rate of 0.05%.
更多查看译文
关键词
Adversarial attack,adversarial retraining,AutoML,DDoS attack detection,flow based analysis
AI 理解论文
溯源树
样例
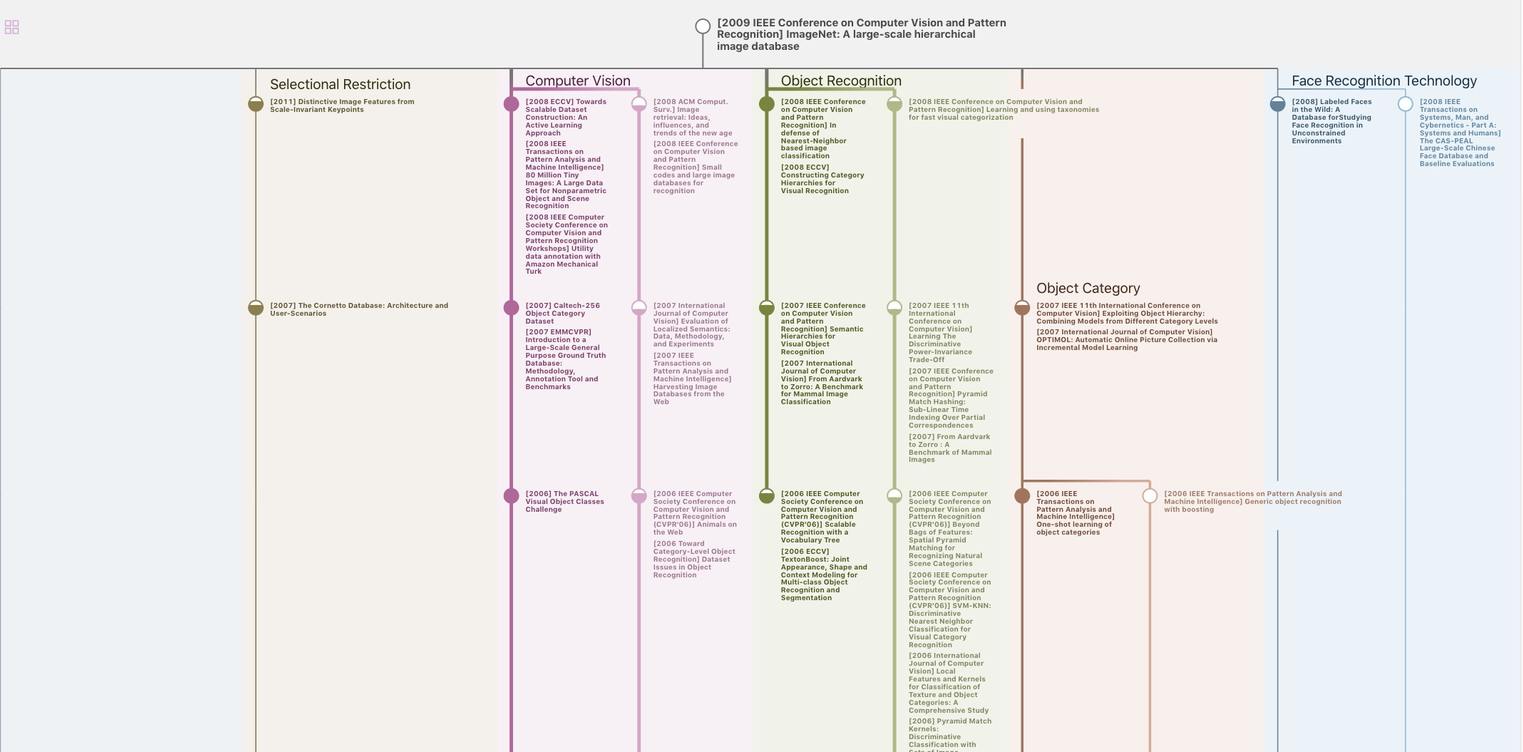
生成溯源树,研究论文发展脉络
Chat Paper
正在生成论文摘要