AutoPooling: Automated Pooling Search for Multi-valued Features in Recommendations
PROCEEDINGS OF THE 17TH ACM INTERNATIONAL CONFERENCE ON WEB SEARCH AND DATA MINING, WSDM 2024(2024)
摘要
Large-scale recommender systems usually contain hundreds of multi-valued feature fields, which have different number of values in each field. For easier computation in traditional fixed-shape neural networks, pooling operators are widely used to compress the multi-valued feature into a fixed-dimension vector. Most existing works set a single pooling method for all fields, but this leads to sub-optimal results, because different feature fields have different information distributions and thus require different pooling methods. In this work, we propose an AutoML-based framework, called AutoPooling, which can automatically and efficiently search for the optimal pooling operator for each multivalued feature. Specifically, learnable weights are assigned to all candidate pooling operators in each feature field. Then an AutoML-based algorithm is used to learn both model parameters and the field-aware weights. Finally, the optimal pooling operator can be acquired based on the associated weights. We evaluate the proposed framework on both public and industrial datasets. The results show that AutoPooling significantly outperforms the benchmarks. Further experiment results show that our method is robust in various deep recommend models and different search spaces.
更多查看译文
关键词
Recommender Systems,AutoML,Pooling,Multi-valued Features
AI 理解论文
溯源树
样例
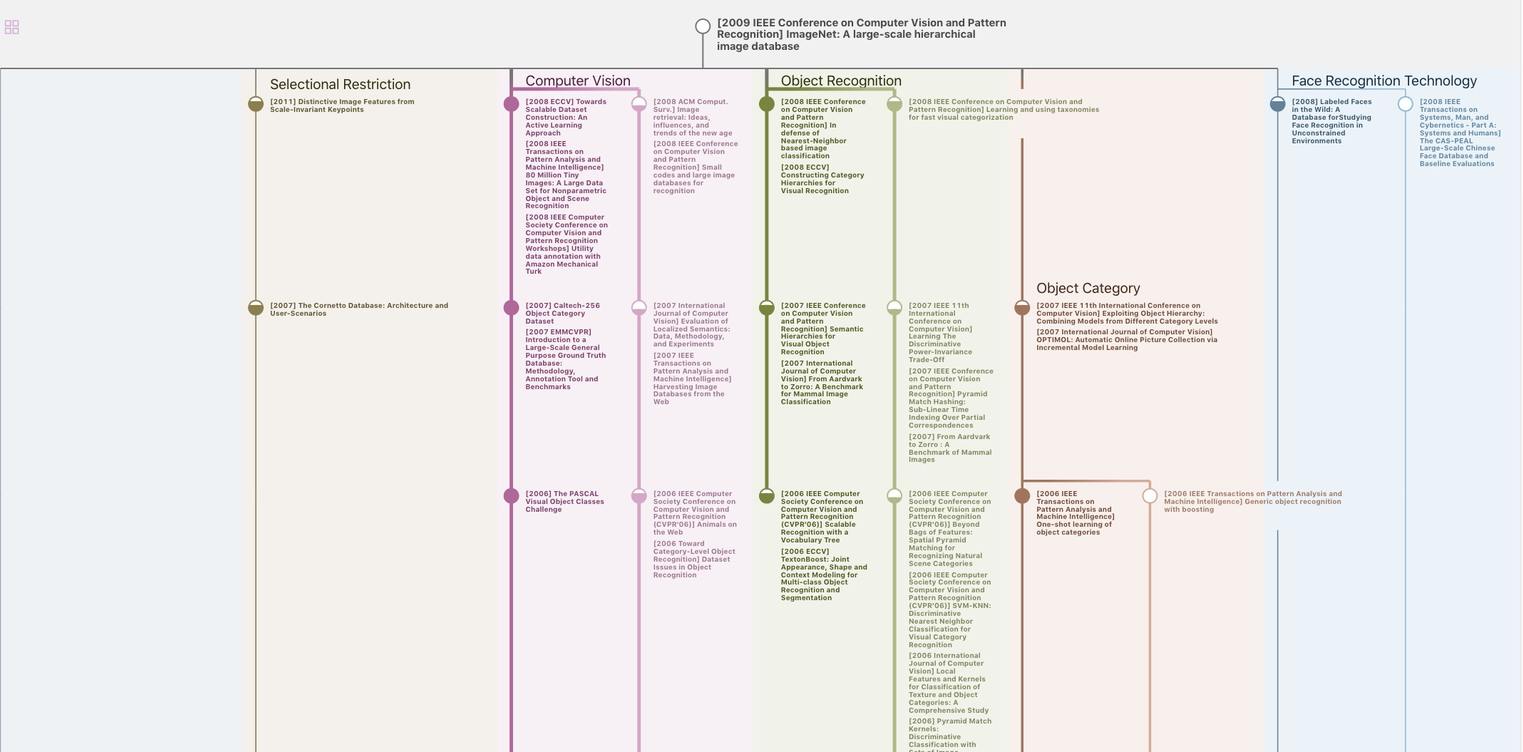
生成溯源树,研究论文发展脉络
Chat Paper
正在生成论文摘要